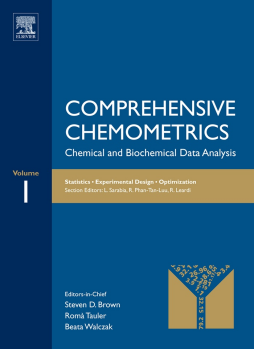
BOOK
Comprehensive Chemometrics
Romà Tauler | Beata Walczak | Steven D. Brown | Steven D. Brown
(2009)
Additional Information
Book Details
Abstract
Designed to serve as the first point of reference on the subject, Comprehensive Chemometrics presents an integrated summary of the present state of chemical and biochemical data analysis and manipulation. The work covers all major areas ranging from statistics to data acquisition, analysis, and applications.
This major reference work provides broad-ranging, validated summaries of the major topics in chemometrics—with chapter introductions and advanced reviews for each area. The level of material is appropriate for graduate students as well as active researchers seeking a ready reference on obtaining and analyzing scientific data.
- Features the contributions of leading experts from 21 countries, under the guidance of the Editors-in-Chief and a team of specialist Section Editors: L. Buydens; D. Coomans; P. Van Espen; A. De Juan; J.H. Kalivas; B.K. Lavine; R. Leardi; R. Phan-Tan-Luu; L.A. Sarabia; and J. Trygg
- Examines the merits and limitations of each technique through practical examples and extensive visuals: 368 tables and more than 1,300 illustrations (750 in full color)
- Integrates coverage of chemical and biological methods, allowing readers to consider and test a range of techniques
- Consists of 2,200 pages and more than 90 review articles, making it the most comprehensive work of its kind
- Offers print and online purchase options, the latter of which delivers flexibility, accessibility, and usability through the search tools and other productivity-enhancing features of ScienceDirect
"An excellent source of up-to-date information…essential for researchers and others working in the field." --Analytical and Bioanalytical Chemistry
"Contains much to interest the spectroscopist…well worthy of a place in any analytical science library." --Spectroscopy Europe
"Highly recommended…Should find a merited place on bookshelves alongside its predecessors." --Alexey L. Pomerantsev and Oxana Ye. Rodionova, Semenov Institute of Chemical Physics RAS, Russia
Table of Contents
Section Title | Page | Action | Price |
---|---|---|---|
e9780444527028v1 | 1 | ||
Series page | 3 | ||
Title page | 4 | ||
Copyright \rPage | 5 | ||
Contents of Volume 1\r | 6 | ||
Contributors to Volume 1 | 8 | ||
Preface | 10 | ||
Editors in Chief | 11 | ||
Contents of All Volumes | 12 | ||
Section Editors | 16 | ||
1.01 An Introduction to the Theory of Sampling: An EssentialPart of Total Quality Management | 20 | ||
Symbols | 36 | ||
1.01.1 Introduction | 21 | ||
1.01.2 Scope | 21 | ||
1.01.3 Definitions and Notations | 22 | ||
1.01.4 Dividing a Complex Problem into its Basic Components | 22 | ||
1.01.5 Exercises Challenging the Reader | 25 | ||
1.01.6 The Critical Importance of Sampling Courses | 28 | ||
1.01.7 The Enemies and Their Link to Geostatistics | 29 | ||
1.01.8 Large-Scale Variability | 29 | ||
1.01.9 Conclusions | 32 | ||
1.01.10 Recommendations | 32 | ||
References | 34 | ||
1.02 Quality of Analytical Measurements: StatisticalMethods for Internal Validation | 36 | ||
1.02.1 Introduction | 37 | ||
1.02.2 Confidence and Tolerance Intervals | 42 | ||
1.02.3 Hypothesis Test | 50 | ||
1.02.4 One-Way Analysis of Variance | 64 | ||
1.02.5 Statistical Inference and Validation | 73 | ||
Appendix | 84 | ||
References | 91 | ||
1.03 Proficiency Testing in Analytical Chemistry | 96 | ||
Symbols | 96 | ||
1.03.1 Overview of Proficiency Testing in Analytical Chemistry | 97 | ||
1.03.2 z-Scoring | 98 | ||
1.03.3 Validation of Test Materials | 109 | ||
1.03.4 Further Information from Proficiency Testing Results | 112 | ||
References | 113 | ||
1.04 Statistical Control of Measures and Processes | 116 | ||
Symbols | 116 | ||
1.04.1 Introduction: Basics of Process Monitoring | 119 | ||
1.04.2 Phases in Process Monitoring | 121 | ||
1.04.3 Shewhart Control Charts | 121 | ||
1.04.4 CUSUM Control Charts | 123 | ||
1.04.5 EWMA Control Charts | 124 | ||
1.04.6 Performance Measures of Control Charts | 125 | ||
1.04.7 Control Charts for Autocorrelated Processes | 127 | ||
1.04.8 Integration of SPC and Engineering Process Control | 130 | ||
1.04.9 Multivariate Control Charts | 131 | ||
1.04.10 Software | 142 | ||
Acknowledgments | 142 | ||
References | 143 | ||
1.05 Quality of Analytical Measurements: UnivariateRegression | 146 | ||
Symbols | 147 | ||
1.05.1 Introduction | 147 | ||
1.05.2 Linear Regression in Calibration: Elements and Procedure | 148 | ||
1.05.3 Statistical Validation of a Calibration Model | 153 | ||
1.05.4 Confidence Intervals and Hypothesis Testing | 159 | ||
1.05.5 The Design of a Calibration | 167 | ||
1.05.6 The Capability of Detection, the Decision Limit, and the Capability of Discrimination Computed from a Regression Model | 170 | ||
1.05.7 Standard Addition Method | 172 | ||
1.05.8 Weighted Least Squares and Generalized Least Squares | 173 | ||
1.05.9 Robust Regression in Calibration | 178 | ||
1.05.10 Errors in Both Variables | 182 | ||
1.05.11 Final Remark | 183 | ||
References | 184 | ||
1.06 Resampling and Testing in Regression Models withEnvironmetrical Applications | 190 | ||
1.06.1 Introduction to Bootstrap | 190 | ||
1.06.2 Bootstrap Resampling Methods for Regression | 192 | ||
1.06.3 Generalized Additive Models | 193 | ||
1.06.4 Constructing CIs in GAMs | 195 | ||
1.06.5 Generalized Additive Model with Interactions | 198 | ||
1.06.6 Bootstrap-Based Methods for Testing Interactions | 199 | ||
References | 203 | ||
1.07 Robust and Nonparametric Statistical Methods | 208 | ||
Symbols | 208 | ||
1.07.1 Introduction | 209 | ||
1.07.2 Location and Scale Estimation | 209 | ||
1.07.3 Correlation and Covariance | 214 | ||
1.07.4 Regression | 216 | ||
1.07.5 Investigation Dependence Structures | 222 | ||
1.07.6 Bibliographic Notes | 227 | ||
Acknowledgments | 228 | ||
References | 228 | ||
1.08 Bayesian Methodology in Statistics | 232 | ||
Symbols | 233 | ||
1.08.1 Introduction and Notation | 233 | ||
1.08.2 Axiomatic Foundations | 235 | ||
1.08.3 Bayesian Methodology | 238 | ||
1.08.4 Reference Analysis | 247 | ||
1.08.5 Inference Summaries | 255 | ||
1.08.6 Discussion | 261 | ||
Acknowledgments | 262 | ||
References | 262 | ||
1.09 Experimental Design: Introduction | 266 | ||
1.09.1 Screening | 267 | ||
1.09.2 Quantitative Study of the Factors | 267 | ||
1.09.3 Response Surface Methodology | 267 | ||
1.09.4 Mixtures or Formulations | 267 | ||
1.09.5 Nonclassical Strategies | 268 | ||
1.10 Screening Strategies | 270 | ||
1.10.1 Introduction | 270 | ||
1.10.2 Screening Saturated Designs | 273 | ||
1.10.3 Supersaturated Designs | 283 | ||
1.10.4 Screening Designs at More than Two Levels | 295 | ||
1.10.5 Multilevel Supersaturated Designs | 300 | ||
1.10.6 Applications of Supersaturated Designs | 310 | ||
1.10.7 Composite Samples and Group Screening | 311 | ||
1.10.8 Sequential Bifurcation | 315 | ||
References | 316 | ||
1.11 The Study of Experimental Factors | 320 | ||
1.11.1 Introduction | 321 | ||
1.11.2 Factorial Designs | 329 | ||
1.11.3 Fractional Factorial Designs | 348 | ||
1.11.4 Concluding Remarks | 361 | ||
Acknowledgments for the Permissions to Use Copyrighted Material | 362 | ||
References | 362 | ||
1.12 Response Surface Methodology | 364 | ||
1.12.1 Introduction | 365 | ||
1.12.2 Elements and Notation | 365 | ||
1.12.3 Optimality of the Variance of the Estimates | 369 | ||
1.12.4 Some Aspects of the Statistical Validation of the Model | 373 | ||
1.12.5 Experimental Design for Fitting Response Surfaces | 385 | ||
1.12.6 Analysis of a Quadratic Response Surface | 394 | ||
1.12.7 Final Remark | 406 | ||
References | 407 | ||
1.13 Experimental Design for Mixture Studies | 410 | ||
1.13.1 Introduction | 410 | ||
1.13.2 Mixture Domain | 412 | ||
1.13.3 Mixture Design for Simplex (-Shaped) Regions | 428 | ||
1.13.4 Designs for Constrained Mixtures | 433 | ||
1.13.5 Analysis and Optimization | 449 | ||
1.13.6 Concluding Remarks | 468 | ||
References | 468 | ||
1.14 Nonclassical Experimental Designs | 472 | ||
1.14.1 Introduction | 472 | ||
1.14.2 Methodological Approach | 474 | ||
1.14.3 Criteria | 476 | ||
1.14.4 Combined Designs | 490 | ||
1.14.5 Final Remark | 513 | ||
References | 516 | ||
1.15 Experimental Designs: Conclusions, Terminology, andSymbols | 520 | ||
1.15.1 Terminology | 521 | ||
1.15.2 Symbols | 522 | ||
1.16 Constrained and Unconstrained Optimization | 526 | ||
Symbols | 526 | ||
1.16.1 Introduction | 527 | ||
1.16.2 Numerical Optimization | 528 | ||
1.16.3 Optimization in Chemometrics | 530 | ||
1.16.4 Unconstrained Optimization Methods | 531 | ||
1.16.5 Globalization Strategies | 543 | ||
1.16.6 Constrained Optimization Methods | 550 | ||
1.16.7 Discussion | 561 | ||
Acknowledgments | 562 | ||
References | 562 | ||
1.17 Sequential Optimization Methods | 566 | ||
Symbols | 566 | ||
1.17.1 Introduction | 568 | ||
1.17.2 Sequential Optimization Methods | 570 | ||
1.17.3 Mixed Sequential–Simultaneous Optimization Methods | 588 | ||
1.17.4 Conclusions | 591 | ||
References | 591 | ||
1.18 Steepest Ascent, Steepest Descent, and GradientMethods | 596 | ||
Symbols | 596 | ||
1.18.1 Introduction | 596 | ||
1.18.2 Method | 599 | ||
1.18.3 Examples | 603 | ||
1.18.4 Conclusion | 608 | ||
References | 608 | ||
1.19 Multicriteria Decision-Making Methods | 610 | ||
1.19.1 Introduction | 610 | ||
1.19.2 Basic Notation | 613 | ||
1.19.3 Illustrative Example | 614 | ||
1.19.4 Multi-Criteria Decision Making Methods | 615 | ||
References | 644 | ||
1.20 Genetic Algorithms | 650 | ||
1.20.1 Introduction | 650 | ||
1.20.2 The Evolution Theory | 651 | ||
1.20.3 How to Transform the Evolution Theory into an Optimization Technique? | 652 | ||
1.20.4 The Problem of Coding | 652 | ||
1.20.5 Steps of the GAs | 654 | ||
1.20.6 Comments about the Parameters of the GAs | 658 | ||
1.20.7 Hybrid Algorithms | 659 | ||
1.20.8 Looking for the Global Maximum: Is it Worthwhile? | 660 | ||
1.20.9 Applications | 660 | ||
1.20.10 A Specific Application: Variable Selection in Spectral Data Sets | 661 | ||
References | 670 | ||
Index to Volume 1 | 674 | ||
e9780444527028v2 | 692 | ||
Series page | 694 | ||
Title page | 695 | ||
Copyright page | 696 | ||
Contents of Volume 2 | 697 | ||
Contributors to Volume 2 | 701 | ||
Preface | 705 | ||
Editors in Chief | 706 | ||
Contents of All Volumes | 707 | ||
Section Editors | 711 | ||
2.01 Background Estimation, Denoising, and Preprocessing | 715 | ||
2.01.1 Introduction | 715 | ||
2.01.2 Improving the Signal-to-Noise Ratio | 716 | ||
2.01.3 Variable Shift, Alignment, and Normalization | 717 | ||
2.01.4 Model-Based Approaches in Preprocessing | 719 | ||
2.01.5 Conclusions and Discussion | 719 | ||
References | 720 | ||
2.02 Denoising and Signal-to-Noise Ratio Enhancement: Classical Filtering | 723 | ||
Symbols | 723 | ||
2.02.1 Introduction | 723 | ||
2.02.2 Nonrecursive Filters | 724 | ||
2.02.3 Recursive Filters | 733 | ||
References | 737 | ||
2.03 Denoising and Signal-to-Noise Ratio Enhancement: Wavelet Transform and Fourier Transform | 739 | ||
Glossary | 739 | ||
2.03.1 Introduction | 740 | ||
2.03.2 Basics of Fourier and Wavelet Analysis | 741 | ||
2.03.3 Off-Line Denoising | 750 | ||
2.03.4 On-line Denoising | 760 | ||
2.03.5 Conclusions | 768 | ||
References | 768 | ||
2.04 Denoising and Signal-to-Noise Ratio Enhancement: Derivatives Derivatives | 771 | ||
Symbols | 771 | ||
2.04.1 Derivatives | 771 | ||
References | 779 | ||
2.05 Denoising and Signal-to-Noise Ratio Enhancement: Splines | 781 | ||
Symbols | 781 | ||
2.05.1 Splines | 781 | ||
References | 795 | ||
2.06 Variable Shift and Alignment | 799 | ||
Symbols | 799 | ||
2.06.1 Introduction | 800 | ||
2.06.2 Data Reduction | 804 | ||
2.06.3 Dynamic Programming | 804 | ||
2.06.4 Alignment Techniques | 806 | ||
2.06.5 Settings and Applications | 819 | ||
References | 820 | ||
2.07 Normalization and Closure | 823 | ||
Symbols | 823 | ||
Glossary | 824 | ||
2.07.1 Introduction | 824 | ||
2.07.2 Statistical Considerations | 826 | ||
2.07.3 General Normalization Methods | 828 | ||
2.07.4 DNA Microarray Normalization | 833 | ||
2.07.5 Assessment and Quality Control of Normalization Performance | 836 | ||
2.07.6 Conclusions | 838 | ||
References | 838 | ||
2.08 Model Based Preprocessing and Background Elimination: OSC, OPLS, and O2PLS | 843 | ||
Symbols | 843 | ||
Glossary | 844 | ||
2.08.1 Orthogonal Signal Correction | 844 | ||
2.08.2 Orthogonal Projections to Latent Structures | 845 | ||
2.08.3 Bidirectional OPLS | 847 | ||
2.08.4 Kernel-Based Orthogonal Projections to Latent Structures | 849 | ||
References | 850 | ||
2.09 Standard Normal Variate, Multiplicative Signal Correction and Extended Multiplicative Signal Correction Preprocessing in Biospectroscopy | 853 | ||
Symbols | 853 | ||
2.09.1 Introduction | 854 | ||
2.09.2 Ideal and Nonideal Spectral Measurements | 854 | ||
2.09.3 Baseline and Scaling Problems | 856 | ||
2.09.4 Linearization of Instrument Response | 857 | ||
2.09.5 Unwanted Instrument-Induced Channel Shifts | 858 | ||
2.09.6 Random Errors | 858 | ||
2.09.7 Advanced Baseline and Scaling Problems | 859 | ||
2.09.8 Formal Description of Extended Multiplicative Signal Correction | 862 | ||
2.09.9 Preprocessing Examples | 864 | ||
2.09.10 Conclusions | 873 | ||
Acknowledgments | 873 | ||
References | 873 | ||
2.10 Batch Process Modeling and MSPC | 877 | ||
2.10.1 Introduction | 877 | ||
2.10.2 Industrial Examples | 879 | ||
2.10.3 Analyzing Historical Batch Process Data | 881 | ||
2.10.4 Online Prediction of Future Trajectories and Final Quality | 898 | ||
2.10.5 Monitoring (MSPC) of Batch Processes | 900 | ||
2.10.6 Control and Optimization of Batch Processes | 906 | ||
2.10.7 Conclusions | 908 | ||
References | 908 | ||
2.11 Evaluation of Preprocessing Methods | 913 | ||
Symbols | 913 | ||
2.11.1 Introduction | 913 | ||
2.11.2 Theoretical Background and Methods | 914 | ||
2.11.3 Example of Evaluation of Preprocessing | 915 | ||
2.11.4 Discussion and Conclusions | 918 | ||
References | 919 | ||
2.12 Linear Soft-Modeling: Introduction | 921 | ||
2.13 Principal Component Analysis: Concept, Geometrical Interpretation, Mathematical Background, Algorithms, History, Practice | 925 | ||
Symbols | 925 | ||
2.13.1 Introduction | 925 | ||
2.13.2 PCA: Basic Concepts and Master Equation | 926 | ||
2.13.3 Geometrical Properties of PCA | 928 | ||
2.13.4 Mathematical Background | 931 | ||
2.13.5 History | 934 | ||
2.13.6 Application Example | 935 | ||
References | 938 | ||
2.14 Independent Component Analysis | 941 | ||
2.14.1 Representation of Multivariate Data | 941 | ||
2.14.2 Blind Source Separation | 944 | ||
2.14.3 Independent Component Analysis | 945 | ||
2.14.4 ICA Estimation Principles | 948 | ||
2.14.5 Validation of Independent Components | 950 | ||
2.14.6 Evaluation of Algorithmic Performance | 951 | ||
2.14.7 Tutorial Examples | 953 | ||
2.14.8 Conclusions | 960 | ||
Acknowledgments | 960 | ||
References | 960 | ||
2.15 Introduction to Multivariate Curve Resolution | 963 | ||
2.15.1 Key Concepts | 963 | ||
2.15.2 History | 967 | ||
2.15.3 Ambiguities | 968 | ||
2.15.4 Applications | 968 | ||
References | 970 | ||
2.16 Two-Way Data Analysis: Evolving Factor Analysis | 975 | ||
2.16.1 Introduction | 975 | ||
2.16.2 The Data | 975 | ||
2.16.3 Abstract Factor Analysis | 976 | ||
2.16.4 Traditional EFA | 978 | ||
2.16.5 Fixed Size Moving Window-EFA | 980 | ||
2.16.6 Exhaustive-EFA | 982 | ||
2.16.7 EFA of Images, Two-Dimensional-EFA | 983 | ||
2.16.8 Computational Aspects | 985 | ||
2.16.9 Using the Results of EFA | 986 | ||
References | 987 | ||
2.17 Two-Way Data Analysis: Detection of Purest Variables | 989 | ||
Symbols | 989 | ||
Glossary | 990 | ||
2.17.1 Introduction | 990 | ||
2.17.2 Key Set Factor Analysis | 991 | ||
2.17.3 Simple-To-Use Interactive Self-Modeling Mixture Analysis | 1001 | ||
2.17.4 Orthogonal Projection Approach | 1010 | ||
2.17.5 Stepwise Maximum Angle Calculation | 1012 | ||
2.17.6 Conclusion | 1017 | ||
Acknowledgment | 1019 | ||
References | 1019 | ||
2.18 Two-Way Data Analysis: Multivariate Curve Resolution – Noniterative Resolution Methods | 1023 | ||
2.18.1 Introduction | 1023 | ||
2.18.2 Evolving Feature and Informative Windows in Two-Way Data | 1024 | ||
2.18.3 Evolving Factor Analysis | 1025 | ||
2.18.4 Heuristic Evolving Latent Projections | 1026 | ||
2.18.5 Window Factor Analysis | 1030 | ||
2.18.6 Subwindow Factor Analysis | 1033 | ||
References | 1035 | ||
2.19 Two-Way Data Analysis: Multivariate Curve Resolution – Iterative Resolution Methods | 1039 | ||
Iterative Resolution Methods | 1039 | ||
2.19.1 Introduction | 1039 | ||
2.19.2 Iterative Methods Based on the Optimization of Transformation Matrices | 1044 | ||
2.19.3 Methods Based on the Optimization of Concentration Profiles and/or Spectra | 1049 | ||
2.19.4 Conclusions | 1054 | ||
References | 1055 | ||
2.20 Two-Way Data Analysis: Multivariate Curve Resolution – Error in Curve Resolution | 1059 | ||
2.20.1 Introduction | 1059 | ||
2.20.2 Characterization of MCR Ambiguities and Error Maps | 1061 | ||
2.20.3 Estimation of MCR Feasible Band Boundaries | 1064 | ||
2.20.4 Error Propagation in MCR Solutions | 1069 | ||
2.20.5 Conclusions | 1075 | ||
References | 1075 | ||
2.21 Multiway Data Analysis: Eigenvector-Based Methods | 1079 | ||
Symbols | 1080 | ||
2.21.1 Introduction | 1081 | ||
2.21.2 Notation and Conventions | 1083 | ||
2.21.3 The Model and Uniqueness Conditions | 1083 | ||
2.21.4 Background and Current Position of GRAM | 1087 | ||
2.21.5 Theoretical Properties of GRAM Results | 1097 | ||
2.21.6 Practical Aspects of GRAM | 1102 | ||
2.21.7 Software | 1108 | ||
2.21.8 Applications | 1108 | ||
2.21.9 Conclusions and Recommendations | 1110 | ||
Appendix 1: Derivation of SK and WSK Methods | 1110 | ||
Appendix 2: Derivation of the DTD Method | 1112 | ||
Appendix 3: The Algebraic Eigenvalue Problem | 1113 | ||
Appendix 4: Matlab Implementation of SK and WSK Methods | 1113 | ||
References | 1114 | ||
2.22 Multilinear Models: Iterative Methods | 1125 | ||
Symbols | 1125 | ||
2.22.1 Introduction | 1126 | ||
2.22.2 PARAFAC Models | 1127 | ||
2.22.3 Tucker Models | 1145 | ||
2.22.4 Hybrid Models | 1156 | ||
Appendix | 1160 | ||
Vectorization, Matricization, and Other Rearrangements of Multiway Arrays | 1162 | ||
References | 1162 | ||
2.23 Multiset Data Analysis: ANOVA Simultaneous Component Analysis and Related Methods | 1167 | ||
2.23.1 Introduction | 1167 | ||
2.23.2 Theory | 1168 | ||
2.23.3 Case Study | 1174 | ||
2.23.4 Relationship of ASCA to Other Methods | 1181 | ||
2.23.5 Further Developments | 1182 | ||
Acknowledgments | 1182 | ||
References | 1183 | ||
2.24 Multiset Data Analysis: Extended Multivariate Curve Resolution | 1187 | ||
2.24.1 Introduction | 1187 | ||
2.24.2 Extension of Multivariate Curve Resolution to the Simultaneous Analysis of Multiple Data Matrices (Multiway Data and Multiset Data) | 1188 | ||
2.24.3 Including Hard Modeling Constraints in Multivariate Curve Resolution | 1201 | ||
2.24.4 Applications | 1205 | ||
2.24.5 Conclusions | 1215 | ||
References | 1215 | ||
2.25 Other Topics in Soft-Modeling: Maximum Likelihood-Based Soft-Modeling Methods | 1221 | ||
2.25.1 Introduction | 1222 | ||
2.25.2 Maximum Likelihood Principal Components Analysis | 1228 | ||
2.25.3 Practical Considerations for MLPCA | 1242 | ||
2.25.4 Related Techniques | 1255 | ||
2.25.5 Applications | 1257 | ||
2.25.6 Conclusions | 1270 | ||
References | 1270 | ||
2.26 Unsupervised Data Mining: Introduction | 1273 | ||
Symbols | 1273 | ||
2.26.1 Introduction | 1273 | ||
2.26.2 Data Sets | 1274 | ||
2.26.3 Uncovering Data Heterogeneity: Cluster Analysis and Data Mapping | 1275 | ||
2.26.4 Clustering Challenges | 1280 | ||
2.26.5 Summary | 1286 | ||
References | 1286 | ||
2.27 Common Clustering Algorithms | 1291 | ||
Symbols | 1292 | ||
2.27.1 Taxonomy of Clustering Algorithms | 1292 | ||
2.27.2 Partitioning Clustering | 1294 | ||
2.27.3 Hierarchical Clustering | 1312 | ||
2.27.4 Hybrid Clustering | 1322 | ||
2.27.5 Constrained Clustering | 1326 | ||
References | 1328 | ||
2.28 Data Mapping: Linear Methods versus Nonlinear Techniques | 1333 | ||
2.28.1 Introduction | 1333 | ||
2.28.2 Linear Methods | 1334 | ||
2.28.3 Nonlinear Methods | 1340 | ||
2.28.4 Discussion | 1344 | ||
References | 1345 | ||
2.29 Density-Based Clustering Methods | 1349 | ||
2.29.1 Introduction | 1349 | ||
2.29.2 Clustering Methods Employing the Concept of Data Density | 1349 | ||
2.29.3 Similarities among Discussed Density-Based Approaches | 1359 | ||
2.29.4 Advantages of the Density-Based Techniques over the Classical Ones | 1359 | ||
2.29.5 Applications | 1361 | ||
References | 1366 | ||
2.30 Model-Based Clustering | 1369 | ||
Symbols | 1369 | ||
2.30.1 Introduction | 1370 | ||
2.30.2 Definition of Mixture Models | 1371 | ||
2.30.3 Maximum Likelihood Estimation | 1371 | ||
2.30.4 Fitting Mixture Models via the EM Algorithm | 1372 | ||
2.30.5 Choice of Starting Values for the EM Algorithm | 1374 | ||
2.30.6 Clustering via Normal Mixtures | 1375 | ||
2.30.7 Spectral Representation of Component-Covariances Matrices | 1376 | ||
2.30.8 Multivariate t-Distribution | 1377 | ||
2.30.9 ML Estimation of Mixtures of t-Distributions | 1377 | ||
2.30.10 Choice of the Number of Components in a Mixture Model | 1379 | ||
2.30.11 Advantages of Mixture Model-Based Clustering | 1380 | ||
2.30.12 Factor Analysis Model for Dimension Reduction | 1380 | ||
2.30.13 Mixtures of Normal Factor Analyzers | 1381 | ||
2.30.14 Mixtures of t-Factor Analyzers | 1385 | ||
2.30.15 Available Software | 1387 | ||
2.30.16 Example | 1387 | ||
2.30.17 Some Recent Extensions for High-Dimensional Data | 1391 | ||
2.30.18 Mixed Feature Data | 1391 | ||
Acknowledgments | 1392 | ||
References | 1393 | ||
2.31 Tree-Based Clustering and Extensions | 1397 | ||
2.31.1 Introduction | 1397 | ||
2.31.2 Regression Trees for Clustering | 1399 | ||
2.31.3 Ensemble Co-occurrence Representation | 1403 | ||
2.31.4 Clustering Co-occurrence Matrices | 1409 | ||
2.31.5 Examples | 1413 | ||
2.31.6 Advantages of Tree-Based Cluster Ensembles | 1416 | ||
2.31.7 Conclusion | 1417 | ||
References | 1417 | ||
Index to Volume 2 | 1421 | ||
e9780444527028v3 | 1451 | ||
Series page | 1453 | ||
Title page | 1454 | ||
Copyright page | 1455 | ||
Contents of Volume 3 | 1456 | ||
Contributors to Volume 3 | 1458 | ||
Preface | 1462 | ||
Editors in Chief | 1463 | ||
Contents of All Volumes | 1464 | ||
Section Editors | 1468 | ||
3.01 Calibration Methodologies | 1472 | ||
3.01.1 Introduction | 1472 | ||
3.01.2 Univariate Regression | 1474 | ||
3.01.3 Multivariate Calibration | 1476 | ||
3.01.4 The Eigenvector (Singular Vector) Basis Set | 1480 | ||
3.01.5 PCR | 1481 | ||
3.01.6 Generic Multivariate Calibration in the Eigenvector Basis Set | 1484 | ||
3.01.7 Selecting Meta-Parameters via the Bias/Variance (Harmony) and Parsimony Balance | 1484 | ||
3.01.8 Tikhonov Regularization and RR | 1487 | ||
3.01.9 PLS | 1493 | ||
3.01.10 PCR, RR, and PLS Intermodel Comparisons | 1494 | ||
3.01.11 Other Multivariate Calibration Methods | 1496 | ||
Appendix 1: Regression Coefficients | 1498 | ||
Appendix 2: MATLAB SVD function | 1498 | ||
Appendix 3: PCR | 1498 | ||
Appendix 4: RR | 1499 | ||
Appendix 5: PLS | 1499 | ||
References | 1500 | ||
3.02 Regression Diagnostics | 1504 | ||
Symbols | 1505 | ||
3.02.1 Introduction | 1506 | ||
3.02.2 Formulation of MLR | 1508 | ||
3.02.3 Diagnostics for MLR | 1519 | ||
3.02.4 Useful Plots in Regression Diagnostics | 1539 | ||
3.02.5 MATLAB Implementation of Regression Diagnostics | 1554 | ||
Appendix 1 The OLS Solution Using Mean-Centered Data | 1555 | ||
Appendix 2 The Confidence Region of OLS Coefficients and Experimental Design | 1555 | ||
Appendix 3 Singular-Value Decomposition | 1556 | ||
References | 1557 | ||
3.03 Validation and Error | 1562 | ||
Symbols | 1562 | ||
3.03.1 Introduction | 1563 | ||
3.03.2 Terminology | 1564 | ||
3.03.3 Calibrations of Increasing Order | 1566 | ||
3.03.4 Uncertainty Estimation | 1571 | ||
3.03.5 Figures of Merit | 1575 | ||
3.03.6 Validation | 1580 | ||
Description of Data Sets and MATLAB Scripts | 1583 | ||
Acknowledgment | 1584 | ||
References | 1584 | ||
3.04 Preprocessing Methods | 1592 | ||
Symbols | 1593 | ||
3.04.1 Introduction | 1594 | ||
3.04.2 Methods for Calibration Data Set Selection | 1597 | ||
3.04.3 Signal Correction Methods | 1609 | ||
3.04.4 Methods for Dimensionality Reduction | 1657 | ||
3.04.5 General Conclusion | 1698 | ||
Acknowledgments | 1698 | ||
References | 1698 | ||
3.05 Variable Selection | 1704 | ||
Symbols | 1704 | ||
Glossary | 1705 | ||
3.05.1 Introduction | 1706 | ||
3.05.2 Criteria for Evaluating Variable Subsets without the Explicit Construction of a Model | 1707 | ||
3.05.3 Criteria for Evaluating Variable Subsets on the Basis of the Regression Results | 1713 | ||
3.05.4 Variable Selection Techniques | 1719 | ||
3.05.5 Case Studies | 1745 | ||
3.05.6 Limitations of Variable Selection | 1751 | ||
References | 1752 | ||
3.06 Missing Data | 1756 | ||
Symbols | 1756 | ||
3.06.1 Introduction | 1758 | ||
3.06.2 Statistical Methods for Handling Missing Data | 1760 | ||
3.06.3 Multivariate Calibration Model Building with Missing Data | 1770 | ||
3.06.4 Software | 1783 | ||
References | 1783 | ||
3.07 Robust Calibration | 1786 | ||
Symbols | 1786 | ||
3.07.1 Introduction | 1787 | ||
3.07.2 Location and Covariance Estimation | 1788 | ||
3.07.3 Linear Calibration in Low Dimensions | 1790 | ||
3.07.4 Principal Component Analysis | 1794 | ||
3.07.5 Linear Calibration in High Dimensions | 1799 | ||
3.07.6 Classification | 1803 | ||
3.07.7 Multiway Analysis | 1807 | ||
3.07.8 Software and Data Availability | 1810 | ||
References | 1810 | ||
3.08 Transfer of Multivariate Calibration Models | 1816 | ||
Symbols | 1816 | ||
3.08.1 Introduction | 1817 | ||
3.08.2 How a Multivariate Calibration Model Can Become Invalid | 1818 | ||
3.08.3 Strategies that Can Be Used before the Model Is Implemented | 1820 | ||
3.08.4 Instrument Standardization Methods | 1822 | ||
3.08.5 Selection of the Standardization Samples | 1837 | ||
3.08.6 Calibration Transfer without Standardization | 1838 | ||
3.08.7 Conclusions | 1843 | ||
3.08.8 Software | 1845 | ||
Acknowledgment | 1845 | ||
References | 1845 | ||
3.09 Three-Way Calibration | 1850 | ||
3.09.1 Introduction | 1851 | ||
3.09.2 The Trilinear Model | 1854 | ||
3.09.3 Historical Algorithms of Trilinear Calibration | 1856 | ||
3.09.4 Alternating Least Squares Methods | 1860 | ||
3.09.5 Data Pretreatment and Preliminary Analyses | 1864 | ||
3.09.6 Data Validation and Postprocessing | 1866 | ||
3.09.7 Figures of Merit | 1868 | ||
3.09.8 Example Analyses | 1869 | ||
Appendix 1 | 1877 | ||
Appendix 2 | 1880 | ||
Appendix 3 | 1881 | ||
References | 1881 | ||
3.10 Model-Based Data Fitting | 1884 | ||
Symbols | 1884 | ||
3.10.1 Introduction | 1885 | ||
3.10.2 Data | 1885 | ||
3.10.3 Models and Parameters | 1887 | ||
3.10.4 Chemical Models | 1889 | ||
3.10.5 Fitting, Sum of Squares, Parameters | 1893 | ||
3.10.6 Other Issues | 1902 | ||
References | 1905 | ||
3.11 Kernel Methods | 1908 | ||
Symbol | 1908 | ||
3.11.1 Introduction | 1908 | ||
3.11.2 Linear and Nonlinear Support Vector Machine Classifiers | 1909 | ||
3.11.3 Support Vector Machine Regression | 1913 | ||
3.11.4 Wider Use of the Kernel Trick | 1915 | ||
3.11.5 Function Estimation in Reproducing Kernel Hilbert Spaces | 1916 | ||
3.11.6 Least Squares Support Vector Machines: Regression and Classification | 1916 | ||
3.11.7 Further Extensions: Kernel PCA/CCA/PLS | 1918 | ||
Acknowledgments | 1920 | ||
References | 1920 | ||
3.12 Linear Approaches for Nonlinear Modeling | 1924 | ||
Symbols | 1924 | ||
3.12.1 Introduction | 1924 | ||
3.12.2 Locally Weighted Regression | 1924 | ||
3.12.3 Radial Basis Function Neural Networks | 1926 | ||
3.12.4 Radial Basis Function Partial Least Squares Regression | 1929 | ||
3.12.5 Neural Fuzzy Systems | 1930 | ||
3.12.6 Conclusions | 1931 | ||
References | 1932 | ||
3.13 Other Methods in Nonlinear Regression | 1934 | ||
Symbols | 1934 | ||
3.13.1 Introduction | 1934 | ||
3.13.2 Classification and Regression Trees | 1936 | ||
3.13.3 Multivariate Adaptive Regression Splines | 1938 | ||
3.13.4 Projection Pursuit Regression | 1942 | ||
3.13.5 Illustrative Example | 1943 | ||
References | 1945 | ||
3.14 Neural Networks | 1948 | ||
Symbols | 1948 | ||
3.14.1 Introduction | 1949 | ||
3.14.2 A Brief History | 1950 | ||
3.14.3 The Artificial Neuron as the Basic Computational Unit | 1951 | ||
3.14.4 From the Neuron to the Network | 1953 | ||
3.14.5 Conclusions | 1974 | ||
References | 1974 | ||
3.15 Classification: Basic Concepts | 1978 | ||
3.15.1 Introduction | 1978 | ||
3.15.2 Linear Discriminant Functions | 1979 | ||
3.15.3 Higher Levels of Classification | 1981 | ||
3.15.4 Conclusion | 1984 | ||
References | 1985 | ||
3.16 Statistical Discriminant Analysis | 1988 | ||
Symbols | 1988 | ||
3.16.1 Introduction | 1988 | ||
3.16.2 Canonical Discriminant Analysis | 1990 | ||
3.16.3 Linear Discriminant Analysis | 1995 | ||
3.16.4 Quadratic Discrimination | 1996 | ||
3.16.5 Shrinkage and Covariance Stabilization | 1997 | ||
3.16.6 Classification in High Dimensions | 2006 | ||
3.16.7 Summary | 2008 | ||
References | 2009 | ||
3.17 Decision Tree Modeling in Classification | 2012 | ||
3.17.1 Introduction | 2013 | ||
3.17.2 Decision Tree Induction | 2015 | ||
3.17.3 Examples | 2022 | ||
3.17.4 Extending Decision Trees | 2029 | ||
3.17.5 Generalizing Feature Space Partitioning | 2031 | ||
3.17.6 Alternative Extensions and Uses of Decision Tree Modeling | 2035 | ||
3.17.7 Software | 2037 | ||
References | 2038 | ||
3.18 Feed-Forward Neural Networks | 2042 | ||
Symbols | 2042 | ||
3.18.1 Introduction | 2042 | ||
3.18.2 The Perceptron | 2043 | ||
3.18.3 Multilayer Perceptrons | 2044 | ||
3.18.4 Optimization of Weights | 2046 | ||
3.18.5 Practical Considerations | 2049 | ||
3.18.6 Applications of Feed-Forward Neural Networks | 2052 | ||
3.18.7 Conclusion | 2055 | ||
References | 2056 | ||
3.19 Validation of Classifiers | 2058 | ||
Symbols | 2058 | ||
3.19.1 Introduction | 2058 | ||
3.19.2 Assessing Predictive Ability Using a Validation Set | 2061 | ||
3.19.3 Assessing Predictive Ability Using Cross-Validation and Bootstrapping | 2061 | ||
3.19.4 Confounding | 2062 | ||
3.19.5 Practical Considerations | 2068 | ||
References | 2069 | ||
3.20 Feature Selection: Introduction | 2072 | ||
Symbols | 2072 | ||
3.20.1 Introduction | 2072 | ||
3.20.2 Data-Driven Science | 2073 | ||
3.20.3 SIMCA | 2074 | ||
3.20.4 Wavelets | 2075 | ||
3.20.5 Genetic Algorithms | 2075 | ||
3.20.6 Conclusions | 2076 | ||
References | 2076 | ||
3.21 Multivariate Approaches: UVE-PLS | 2080 | ||
Symbols | 2080 | ||
3.21.1 Introduction | 2080 | ||
3.21.2 Uninformative Variable Elimination by PLS Algorithm | 2081 | ||
3.21.3 Examples | 2083 | ||
3.21.4 Other Applications | 2087 | ||
References | 2088 | ||
3.22 Multivariate Approaches to Classification using Genetic Algorithms | 2090 | ||
Symbols | 2090 | ||
3.22.1 Introduction | 2090 | ||
3.22.2 Genetic Algorithms | 2093 | ||
3.22.3 PCKaNN | 2095 | ||
3.22.4 Ordinal Classes | 2099 | ||
3.22.5 Robustification of PCKaNN | 2099 | ||
3.22.6 Incorporation of Transverse Learning in PCKaNN | 2100 | ||
3.22.7 Applications of the Pattern Recognition GA | 2101 | ||
3.22.8 Conclusion | 2114 | ||
References | 2115 | ||
3.23 Feature Selection in the Wavelet Domain: Adaptive Wavelets | 2118 | ||
Symbols | 2118 | ||
3.23.1 Introduction | 2120 | ||
3.23.2 Wavelets | 2121 | ||
3.23.3 Statistical Methods Utilizing Adaptive Wavelets | 2130 | ||
3.23.4 Applications of Adaptive Wavelets | 2137 | ||
3.23.5 Concluding Remarks | 2147 | ||
References | 2148 | ||
3.24 Robust Multivariate Methods in Chemometrics | 2152 | ||
Symbols | 2153 | ||
3.24.1 Introduction | 2154 | ||
3.24.2 Designing Robust Multivariate Estimators | 2158 | ||
3.24.3 Robust Regression | 2161 | ||
3.24.4 Robust Alternatives to Principal Component Analysis | 2171 | ||
3.24.5 Robust Alternatives to Partial Least Squares | 2173 | ||
3.24.6 Robust Approaches to Discriminant Analysis | 2181 | ||
3.24.7 Validation | 2186 | ||
References | 2190 | ||
Index to Volume 3 | 2194 | ||
e9780444527028v4 | 2226 | ||
Series page | 2228 | ||
Title page | 2229 | ||
Copyright page | 2230 | ||
Contents of Volume 4 | 2231 | ||
Contributors to Volume 4 | 2233 | ||
Preface | 2237 | ||
Editors in Chief | 2238 | ||
Contents of All Volumes | 2239 | ||
Section Editors | 2243 | ||
4.01 Representative Sampling, Data Quality, Validation – A Necessary Trinity in Chemometrics | 2247 | ||
Symbols | 2247 | ||
4.01.1 Introduction: Sampling of Heterogeneous Lots | 2248 | ||
4.01.2 Heterogeneity | 2248 | ||
4.01.3 Types of Sampling Errors Versus Practical Sampling | 2250 | ||
4.01.4 Representative Mass Reduction | 2254 | ||
4.01.5 Process Sampling (1-D Lots) | 2255 | ||
4.01.6 Sampling Errors – Summary | 2261 | ||
4.01.7 Seven Sampling Unit Operations | 2262 | ||
4.01.8 Data Quality | 2262 | ||
4.01.9 Validation in Chemometrics | 2263 | ||
References | 2264 | ||
4.02 Multivariate Statistical Process Control and Process Control, Using Latent Variables | 2267 | ||
4.02.1 Introduction | 2268 | ||
4.02.2 Traditional SPC Charts | 2269 | ||
4.02.3 Latent Variable Based Process Monitoring | 2275 | ||
4.02.4 Handling Future Observations with Missing Data | 2286 | ||
4.02.5 Adaptive Latent Variable Models | 2287 | ||
4.02.6 Batch Process Monitoring | 2288 | ||
4.02.7 Monitoring Transitions in Continuous Processes | 2292 | ||
4.02.8 Multistage Operations – Mutiblock Analysis | 2293 | ||
4.02.9 Process Control Using Latent Variable Methods | 2294 | ||
4.02.10 MIA for MSPC and Control | 2295 | ||
4.02.11 Concluding Remarks | 2296 | ||
References | 2297 | ||
4.03 Environmental Chemometrics | 2301 | ||
Symbols | 2301 | ||
4.03.1 Introduction | 2301 | ||
4.03.2 Pattern Recognition | 2302 | ||
4.03.3 Mixture Resolution Problem | 2304 | ||
4.03.4 Regression | 2308 | ||
4.03.5 Multivariate Calibration Methods | 2309 | ||
4.03.6 Factor Analysis | 2310 | ||
4.03.7 Probability Estimates | 2312 | ||
4.03.8 Summary | 2316 | ||
References | 2316 | ||
4.04 Application of Chemometrics to Food Chemistry | 2321 | ||
4.04.1 Introduction | 2321 | ||
4.04.2 History | 2323 | ||
4.04.3 Overview | 2324 | ||
4.04.4 Objectives and Possible Improvements | 2348 | ||
Data Sets | 2362 | ||
References | 2370 | ||
4.05 Chemometrics in QSAR | 2375 | ||
4.05.1 Introduction | 2375 | ||
4.05.2 Short History of QSAR and Molecular Descriptors | 2377 | ||
4.05.3 Chemometrics and QSAR Modeling | 2379 | ||
4.05.4 Specific QSAR Approaches | 2384 | ||
4.05.5 Molecular Descriptors | 2390 | ||
4.05.6 Molecular Descriptor Selection | 2397 | ||
4.05.7 Principles for QSAR Modeling | 2403 | ||
4.05.8 Conclusions | 2410 | ||
References | 2410 | ||
4.06 Spectroscopic Imaging | 2419 | ||
Symbols | 2419 | ||
4.06.1 Introduction | 2419 | ||
4.06.2 Introduction to NIR Imaging | 2420 | ||
4.06.3 Chemometrics and NIR Imaging | 2421 | ||
4.06.4 Example Applications | 2428 | ||
4.06.5 Conclusion | 2436 | ||
References | 2436 | ||
4.07 Spectral Map Analysis of Microarray Data | 2443 | ||
4.07.1 Introduction | 2443 | ||
4.07.2 Microarray Technology | 2444 | ||
4.07.3 Data Analysis of Microarrays | 2445 | ||
4.07.4 Factor Analytic Thinking | 2446 | ||
4.07.5 The Distinction between Size and Shape | 2449 | ||
4.07.6 The Biplot Graphic | 2450 | ||
4.07.7 Logarithms | 2450 | ||
4.07.8 Singular Value Decomposition | 2451 | ||
4.07.9 The Golub Data Set | 2452 | ||
4.07.10 Principal Component Analysis | 2452 | ||
4.07.11 Correspondence Analysis | 2454 | ||
4.07.12 Spectral Map Analysis | 2455 | ||
4.07.13 Interpretation of Spectral Maps Using Interactive Graphics | 2457 | ||
4.07.14 The Size–Contrast Diagram | 2459 | ||
4.07.15 Conclusion | 2460 | ||
Acknowledgments | 2460 | ||
References | 2460 | ||
4.08 Analysis of Megavariate Data in Functional Genomics | 2467 | ||
Symbols | 2468 | ||
4.08.1 Introduction | 2468 | ||
4.08.2 Molecular Basis of Functional Genomics | 2469 | ||
4.08.3 Important Considerations in Functional Genomics | 2473 | ||
4.08.4 Data Analysis | 2479 | ||
4.08.5 Concluding Remarks | 2516 | ||
Acknowledgment | 2517 | ||
Appendix | 2518 | ||
A.1 Matlab Codes for FDR Adjustment of Significance Test in PLSR | 2518 | ||
A.2 Matlab Codes for Efficient Regression Algorithm for Megavariate Data | 2519 | ||
A.3 Matlab Codes for Bootstrap of GEMANOVA Model with One Component | 2520 | ||
References | 2521 | ||
4.09 Systems Biology | 2525 | ||
Symbols | 2525 | ||
4.09.1 Introduction | 2526 | ||
4.09.2 Study Setup | 2531 | ||
4.09.3 Data Preprocessing | 2536 | ||
4.09.4 Data Analysis | 2538 | ||
4.09.5 Metabolite Identification | 2545 | ||
4.09.6 Interpretation and Visualization | 2548 | ||
References | 2552 | ||
4.10 Chemometrics Role within the PAT Context: Examples from Primary Pharmaceutical Manufacturing | 2559 | ||
Symbols | 2559 | ||
4.10.1 Introduction | 2559 | ||
4.10.2 NIRS and Chemometrics in API Production | 2565 | ||
4.10.3 Case Studies | 2570 | ||
4.10.4 Conclusions | 2595 | ||
References | 2598 | ||
4.11 Smart Sensors | 2603 | ||
4.11.1 Introduction | 2603 | ||
4.11.2 Toward ‘Smart Sensors’ Featuring On-Sensor Chemometrics | 2603 | ||
4.11.3 Toward Robust Chemometrics for Spectroscopic Analyses | 2604 | ||
4.11.4 Toward Spectrochemical Imaging | 2616 | ||
4.11.5 Future Perspectives of Chemometrics for ‘Smart Sensors’ | 2617 | ||
References | 2620 | ||
4.12 Chemometric Analysis of Sensory Data | 2623 | ||
Symbols | 2624 | ||
4.12.1 Introduction | 2624 | ||
4.12.2 The Methodology of Sensory Analysis | 2627 | ||
4.12.3 Experimental Design | 2633 | ||
4.12.4 Data Formats and Pretreatment | 2636 | ||
4.12.5 Graphical Displays of Data | 2637 | ||
4.12.6 Univariate Statistics | 2641 | ||
4.12.7 Multivariate Analysis | 2643 | ||
4.12.8 Other Methodology in Sensory Analysis | 2659 | ||
4.12.9 Case Studies | 2661 | ||
4.12.10 Discussion | 2664 | ||
References | 2665 | ||
4.13 Chemometrics in Electrochemistry | 2671 | ||
4.13.1 Introduction | 2671 | ||
4.13.2 Experimental Design and Optimization | 2672 | ||
4.13.3 Data Preparation and Transformation | 2676 | ||
4.13.4 Data Exploration and Sample Classification | 2678 | ||
4.13.5 Determination of Concentrations and Calibration | 2679 | ||
4.13.6 Knowledge-Based Expert Systems | 2697 | ||
4.13.7 Conclusions | 2699 | ||
Acknowledgments | 2700 | ||
References | 2701 | ||
4.14 Chemoinformatics | 2705 | ||
Symbols | 2706 | ||
4.14.1 Introduction | 2706 | ||
4.14.2 The Origins and Scope of Chemoinformatics | 2706 | ||
4.14.3 Teaching Computers Chemistry: Data Input Problems | 2709 | ||
4.14.4 In Silico Chemistry: Data Processing and Data Output Problems | 2717 | ||
4.14.5 Internet Resources for Chemistry and Chemoinformatics | 2744 | ||
4.14.6 Conclusions and Further Trends | 2745 | ||
4.14.7 Sources of Further Information and Advice | 2746 | ||
References | 2746 | ||
4.15 High-Performance GRID Computing in Chemoinformatics | 2753 | ||
Glossary | 2754 | ||
4.15.1 Introduction | 2754 | ||
4.15.2 Grid Components and Concepts | 2758 | ||
4.15.3 Interfacing with the Grid | 2767 | ||
4.15.4 Grid Security and Integrity | 2770 | ||
4.15.5 Migrating Existing Applications for the Grid | 2773 | ||
4.15.6 Grid Computing in the Laboratory | 2777 | ||
4.15.7 Case Study: Grid-Based Benchmarking of QSAR Data | 2779 | ||
References | 2782 | ||
Cumulative Index | 2787 |