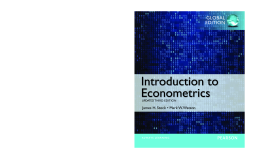
Additional Information
Book Details
Abstract
For courses in Introductory Econometrics
Engaging applications bring the theory and practice of modern econometrics to life
Ensure students grasp the relevance of econometrics with Introduction to Econometrics–the text that connects modern theory and practice with motivating, engaging applications.
The Third Edition Update maintains a focus on currency, while building on the philosophy that applications should drive the theory, not the other way around.
This program provides a better teaching and learning experience–for you and your students. Here’s how:
-
Keeping it current with new and updated discussions on topics of particular interest to today’s students.
-
Presenting consistency through theory that matches application.
-
Offering a full array of pedagogical features.
MyEconLab® is not included. Students, if MyEconLab is a recommended/mandatory component of the course, please ask your instructor for the correct ISBN. MyEconLab should only be purchased when required by an instructor.
Table of Contents
Section Title | Page | Action | Price |
---|---|---|---|
Cover | Cover | ||
Title Page | TitlePage | ||
Copyright | Copyright | ||
Contents | 9 | ||
Preface | 31 | ||
PART ONE Introduction and Review | 46 | ||
CHAPTER 1 Economic Questions and Data | 47 | ||
1.1 Economic Questions We Examine | 47 | ||
Question | 48 | ||
PART TWO Fundamentals of Regression Analysis | 155 | ||
CHAPTER 4 Linear Regression with One Regressor | 155 | ||
4.1 The Linear Regression Model | 155 | ||
4.2 Estimating the Coefficients of the Linear Regression Model | 160 | ||
The Ordinary Least Squares Estimator | 162 | ||
OLS Estimates of the Relationship Between Test Scores and the Student-Teacher Ratio | 164 | ||
Why Use the OLS Estimator? | 165 | ||
4.3 Measures of Fit | 167 | ||
The R2 | 167 | ||
The Standard Error of the Regression | 168 | ||
Application to the Test Score Data | 169 | ||
4.4 The Least Squares Assumptions | 170 | ||
Assumption | 170 | ||
Assumption | 172 | ||
Assumption | 173 | ||
Use of the Least Squares Assumptions | 174 | ||
4.5 Sampling Distribution of the OLS Estimators | 175 | ||
The Sampling Distribution of the OLS Estimators | 176 | ||
4.6 Conclusion | 179 | ||
APPENDIX 4.1 The California Test Score Data Set | 187 | ||
APPENDIX 4.2 Derivation of the OLS Estimators | 187 | ||
APPENDIX 4.3 Sampling Distribution of the OLS Estimator | 188 | ||
CHAPTER 5 Regression with a Single Regressor: Hypothesis Tests and Confidence Intervals | 192 | ||
5.1 Testing Hypotheses About One of the Regression Coefficients | 192 | ||
Two-Sided Hypotheses Concerning β | 193 | ||
One-Sided Hypotheses Concerning β1 | 196 | ||
Testing Hypotheses About the Intercept β0 | 198 | ||
5.2 Confidence Intervals for a Regression Coefficient | 199 | ||
5.3 Regression When X Is a Binary Variable | 201 | ||
Interpretation of the Regression Coefficients | 201 | ||
5.4 Heteroskedasticity and Homoskedasticity | 203 | ||
What Are Heteroskedasticity and Homoskedasticity? | 204 | ||
Mathematical Implications of Homoskedasticity | 206 | ||
What Does This Mean in Practice? | 207 | ||
5.5 The Theoretical Foundations of Ordinary Least Squares | 209 | ||
Linear Conditionally Unbiased Estimators and the Gauss-Markov Theorem | 210 | ||
Regression Estimators Other Than OLS | 211 | ||
5.6 Using the t-Statistic in Regression When the Sample Size Is Small | 212 | ||
The t-Statistic and the Student t Distribution | 212 | ||
Use of the Student t Distribution in Practice | 213 | ||
5.7 Conclusion | 214 | ||
APPENDIX 5.1 Formulas for OLS Standard Errors | 223 | ||
APPENDIX 5.2 The Gauss-Markov Conditions and a Proof of the Gauss-Markov Theorem | 224 | ||
CHAPTER 6 Linear Regression with Multiple Regressors | 228 | ||
6.1 Omitted Variable Bias | 228 | ||
Definition of Omitted Variable Bias | 229 | ||
A Formula for Omitted Variable Bias | 231 | ||
Addressing Omitted Variable Bias by Dividing the Data into Groups | 233 | ||
6.2 The Multiple Regression Model | 235 | ||
The Population Regression Line | 235 | ||
The Population Multiple Regression Model | 236 | ||
6.3 The OLS Estimator in Multiple Regression | 238 | ||
The OLS Estimator | 239 | ||
Application to Test Scores and the Student-Teacher Ratio | 240 | ||
6.4 Measures of Fit in Multiple Regression | 242 | ||
The Standard Error of the Regression (SER) | 242 | ||
The R2 | 242 | ||
The \"Adjusted R2 | 243 | ||
Application to Test Scores | 244 | ||
6.5 The Least Squares Assumptions in Multiple Regression | 245 | ||
PART THREE Further Topics in Regression Analysis | 396 | ||
CHAPTER 10 Regression with Panel Data | 396 | ||
10.1 Panel Data | 397 | ||
Example: Traffic Deaths and Alcohol Taxes | 398 | ||
10.2 Panel Data with Two Time Periods: \"Before and After\" Comparisons | 400 | ||
10.3 Fixed Effects Regression | 403 | ||
The Fixed Effects Regression Model | 403 | ||
Estimation and Inference | 405 | ||
Application to Traffic Deaths | 407 | ||
10.4 Regression with Time Fixed Effects | 407 | ||
Time Effects Only | 408 | ||
Both Entity and Time Fixed Effects | 409 | ||
10.5 The Fixed Effects Regression Assumptions and Standard Errors for Fixed Effects Regression | 411 | ||
The Fixed Effects Regression Assumptions | 411 | ||
Standard Errors for Fixed Effects Regression | 413 | ||
10.6 Drunk Driving Laws and Traffic Deaths | 414 | ||
10.7 Conclusion | 418 | ||
APPENDIX 10.1 The State Traffic Fatality Data Set | 426 | ||
APPENDIX 10.2 Standard Errors for Fixed Effects Regression | 426 | ||
CHAPTER 11 Regression with a Binary Dependent Variable | 431 | ||
11.1 Binary Dependent Variables and the Linear Probability Model | 432 | ||
Binary Dependent Variables | 432 | ||
The Linear Probability Model | 434 | ||
11.2 Probit and Logit Regression | 437 | ||
Probit Regression | 437 | ||
Logit Regression | 442 | ||
Comparing the Linear Probability, Probit, and Logit Models | 444 | ||
11.3 Estimation and Inference in the Logit and Probit Models | 444 | ||
Nonlinear Least Squares Estimation | 445 | ||
Maximum Likelihood Estimation | 446 | ||
Measures of Fit | 447 | ||
11.4 Application to the Boston HMDA Data | 448 | ||
11.5 Conclusion | 455 | ||
APPENDIX 11.1 The Boston HMDA Data Set | 464 | ||
APPENDIX 11.2 Maximum Likelihood Estimation | 464 | ||
APPENDIX 11.3 Other Limited Dependent Variable Models | 467 | ||
CHAPTER 12 Instrumental Variables Regression | 470 | ||
12.1 The IV Estimator with a Single Regressor and a Single Instrument | 471 | ||
The IV Model and Assumptions | 471 | ||
The Two Stage Least Squares Estimator | 472 | ||
Why Does IV Regression Work? | 473 | ||
The Sampling Distribution of the TSLS Estimator | 477 | ||
Application to the Demand for Cigarettes | 479 | ||
12.2 The General IV Regression Model | 481 | ||
TSLS in the General IV Model | 483 | ||
Instrument Relevance and Exogeneity in the General IV Model | 484 | ||
The IV Regression Assumptions and Sampling Distribution of the TSLS Estimator | 485 | ||
Inference Using the TSLS Estimator | 486 | ||
Application to the Demand for Cigarettes | 487 | ||
12.3 Checking Instrument Validity | 488 | ||
Assumption | 489 | ||
Assumption | 491 | ||
12.4 Application to the Demand for Cigarettes | 494 | ||
12.5 Where Do Valid Instruments Come From? | 499 | ||
Three Examples | 500 | ||
12.6 Conclusion | 504 | ||
APPENDIX 12.1 The Cigarette Consumption Panel Data Set | 513 | ||
APPENDIX 12.2 Derivation of the Formula for the TSLS Estimator in Equation (12.4) | 513 | ||
APPENDIX 12.3 Large-Sample Distribution of the TSLS Estimator | 514 | ||
APPENDIX 12.4 Large-Sample Distribution of the TSLS Estimator When the Instrument Is Not Valid | 515 | ||
APPENDIX 12.5 Instrumental Variables Analysis with Weak Instruments | 517 | ||
APPENDIX 12.6 TSLS with Control Variables | 519 | ||
CHAPTER 13 Experiments and Quasi-Experiments | 521 | ||
13.1 Potential Outcomes, Causal Effects, and Idealized Experiments | 522 | ||
Potential Outcomes and the Average Causal Effect | 522 | ||
Econometric Methods for Analyzing Experimental Data | 524 | ||
13.2 Threats to Validity of Experiments | 525 | ||
Threats to Internal Validity | 525 | ||
Threats to External Validity | 529 | ||
13.3 Experimental Estimates of the Effect of Class Size Reductions | 530 | ||
Experimental Design | 531 | ||
Analysis of the STAR Data | 532 | ||
Comparison of the Observational and Experimental Estimates of Class Size Effects | 537 | ||
13.4 Quasi-Experiments | 539 | ||
Examples | 540 | ||
The Differences-in-Differences Estimator | 542 | ||
Instrumental Variables Estimators | 545 | ||
Regression Discontinuity Estimators | 546 | ||
13.5 Potential Problems with Quasi-Experiments | 548 | ||
Threats to Internal Validity | 548 | ||
Threats to External Validity | 550 | ||
13.6 Experimental and Quasi-Experimental Estimates in Heterogeneous Populations | 550 | ||
OLS with Heterogeneous Causal Effects | 551 | ||
IV Regression with Heterogeneous Causal Effects | 552 | ||
13.7 Conclusion | 555 | ||
APPENDIX 13.1 The Project STAR Data Set | 564 | ||
APPENDIX 13.2 IV Estimation When the Causal Effect Varies Across Individuals | 564 | ||
APPENDIX 13.3 The Potential Outcomes Framework for Analyzing Data from Experiments | 566 | ||
PART FOUR Regression Analysis of Economic Time Series Data | 568 | ||
CHAPTER 14 Introduction to Time Series Regression and Forecasting | 568 | ||
14.1 Using Regression Models for Forecasting | 569 | ||
14.2 Introduction to Time Series Data and Serial Correlation | 570 | ||
Real GDP in the United States | 570 | ||
Lags, First Differences, Logarithms, and Growth Rates | 571 | ||
Autocorrelation | 574 | ||
Other Examples of Economic Time Series | 575 | ||
14.3 Autoregressions | 577 | ||
The First-Order Autoregressive Model | 577 | ||
The pth-Order Autoregressive Model | 580 | ||
14.4 Time Series Regression with Additional Predictors and the Autoregressive Distributed Lag Model | 583 | ||
Forecasting GDP Growth Using the Term Spread Stationarity | 583 | ||
Time Series Regression with Multiple Predictors | 587 | ||
Forecast Uncertainty and Forecast Intervals | 590 | ||
14.5 Lag Length Selection Using Information Criteria | 593 | ||
Determining the Order of an Autoregression | 593 | ||
Lag Length Selection in Time Series Regression with Multiple Predictors | 596 | ||
14.6 Nonstationarity I: Trends | 597 | ||
What Is a Trend? | 597 | ||
Problems Caused by Stochastic Trends | 600 | ||
Detecting Stochastic Trends: Testing for a Unit AR Root | 602 | ||
Avoiding the Problems Caused by Stochastic Trends | 607 | ||
14.7 Nonstationarity II: Breaks | 607 | ||
What Is a Break? | 608 | ||
Testing for Breaks | 608 | ||
Pseudo Out-of-Sample Forecasting | 613 | ||
Avoiding the Problems Caused by Breaks | 619 | ||
14.8 Conclusion | 619 | ||
APPENDIX 14.1 Time Series Data Used in Chapter 14 | 629 | ||
APPENDIX 14.2 Stationarity in the AR(1) Model | 630 | ||
APPENDIX 14.3 Lag Operator Notation | 631 | ||
APPENDIX 14.4 ARMA Models | 632 | ||
APPENDIX 14.5 Consistency of the BIC Lag Length Estimator | 633 | ||
CHAPTER 15 Estimation of Dynamic Causal Effects | 635 | ||
15.1 An Initial Taste of the Orange Juice Data | 636 | ||
15.2 Dynamic Causal Effects | 639 | ||
Causal Effects and Time Series Data | 639 | ||
Two Types of Exogeneity | 642 | ||
15.3 Estimation of Dynamic Causal Effects with Exogenous Regressors | 643 | ||
The Distributed Lag Model Assumptions | 644 | ||
Autocorrelated ut, Standard Errors, and Inference | 645 | ||
Dynamic Multipliers and Cumulative Dynamic Multipliers | 646 | ||
15.4 Heteroskedasticity- and Autocorrelation-Consistent Standard Errors | 647 | ||
Distribution of the OLS Estimator with Autocorrelated Errors | 648 | ||
HAC Standard Errors | 650 | ||
15.5 Estimation of Dynamic Causal Effects with Strictly Exogenous Regressors | 652 | ||
The Distributed Lag Model with AR(1) Errors | 653 | ||
OLS Estimation of the ADL Model | 656 | ||
GLS Estimation | 657 | ||
The Distributed Lag Model with Additional Lags and AR(p) Errors | 659 | ||
15.6 Orange Juice Prices and Cold Weather | 662 | ||
15.7 Is Exogeneity Plausible? Some Examples | 670 | ||
U.S. Income and Australian Exports | 670 | ||
Oil Prices and Inflation | 671 | ||
Monetary Policy and Inflation | 672 | ||
The Growth Rate of GDP and the Term Spread | 672 | ||
15.8 Conclusion | 673 | ||
APPENDIX 15.1 The Orange Juice Data Set | 680 | ||
APPENDIX 15.2 The ADL Model and Generalized Least Squares in Lag Operator Notation | 680 | ||
CHAPTER 16 Additional Topics in Time Series Regression | 684 | ||
16.1 Vector Autoregressions | 684 | ||
The VAR Model | 685 | ||
A VAR Model of the Growth Rate of GDP and the Term Spread | 688 | ||
16.2 Multiperiod Forecasts | 689 | ||
Iterated Multiperiod Forecasts | 689 | ||
Direct Multiperiod Forecasts | 691 | ||
Which Method Should You Use? | 694 | ||
16.3 Orders of Integration and the DF-GLS Unit Root Test | 695 | ||
Other Models of Trends and Orders of Integration | 695 | ||
The DF-GLS Test for a Unit Root | 697 | ||
Why Do Unit Root Tests Have Nonnormal Distributions? | 700 | ||
16.4 Cointegration | 702 | ||
Cointegration and Error Correction | 702 | ||
How Can You Tell Whether Two Variables Are Cointegrated? | 704 | ||
Estimation of Cointegrating Coefficients | 705 | ||
Extension to Multiple Cointegrated Variables | 707 | ||
Application to Interest Rates | 708 | ||
16.5 Volatility Clustering and Autoregressive Conditional Heteroskedasticity | 710 | ||
Volatility Clustering | 710 | ||
Autoregressive Conditional Heteroskedasticity | 712 | ||
Application to Stock Price Volatility | 713 | ||
16.6 Conclusion | 716 | ||
PART FIVE The Econometric Theory of Regression Analysis | 722 | ||
CHAPTER 17 The Theory of Linear Regression with One Regressor | 722 | ||
17.1 The Extended Least Squares Assumptions and the OLS Estimator | 723 | ||
The Extended Least Squares Assumptions | 723 | ||
The OLS Estimator | 725 | ||
17.2 Fundamentals of Asymptotic Distribution Theory | 725 | ||
Convergence in Probability and the Law of Large Numbers | 726 | ||
The Central Limit Theorem and Convergence in Distribution | 728 | ||
Slutsky's Theorem and the Continuous Mapping Theorem | 729 | ||
Application to the t-Statistic Based on the Sample Mean | 730 | ||
17.3 Asymptotic Distribution of the OLS Estimator and t-Statistic | 731 | ||
Consistency and Asymptotic Normality of the OLS Estimators | 731 | ||
Consistency of Heteroskedasticity-Robust Standard Errors | 731 | ||
Asymptotic Normality of the Heteroskedasticity-Robust t-Statistic | 733 | ||
17.4 Exact Sampling Distributions When the Errors Are Normally Distributed | 733 | ||
Distribution of β with Normal Errors\r | 733 | ||
Distribution of the Homoskedasticity-Only t-Statistic | 735 | ||
17.5 Weighted Least Squares | 736 | ||
WLS with Known Heteroskedasticity | 736 | ||
WLS with Heteroskedasticity of Known Functional Form | 737 | ||
Heteroskedasticity-Robust Standard Errors or WLS? | 740 | ||
APPENDIX 17.1 The Normal and Related Distributions and Moments of Continuous Random Variables | 746 | ||
APPENDIX 17.2 Two Inequalities | 749 | ||
CHAPTER 18 The Theory of Multiple Regression | 751 | ||
18.1 The Linear Multiple Regression Model and OLS Estimator in Matrix Form | 752 | ||
The Multiple Regression Model in Matrix Notation | 752 | ||
The Extended Least Squares Assumptions | 754 | ||
The OLS Estimator | 755 | ||
18.2 Asymptotic Distribution of the OLS Estimator and t-Statistic | 756 | ||
The Multivariate Central Limit Theorem | 756 | ||
Asymptotic Normality of β | 757 | ||
Heteroskedasticity-Robust Standard Errors | 758 | ||
Confidence Intervals for Predicted Effects | 759 | ||
Asymptotic Distribution of the t-Statistic | 759 | ||
18.3 Tests of Joint Hypotheses | 759 | ||
Joint Hypotheses in Matrix Notation | 760 | ||
Asymptotic Distribution of the F-Statistic | 760 | ||
Confidence Sets for Multiple Coefficients | 761 | ||
18.4 Distribution of Regression Statistics with Normal Errors | 762 | ||
Matrix Representations of OLS Regression Statistics | 762 | ||
Distribution of β with Normal Errors | 763 | ||
Distribution of s2/u | 764 | ||
Homoskedasticity-Only Standard Errors | 764 | ||
Distribution of the t-Statistic | 765 | ||
Distribution of the F-Statistic | 765 | ||
18.5 Efficiency of the OLS Estimator with Homoskedastic Errors | 766 | ||
The Gauss-Markov Conditions for Multiple Regression | 766 | ||
Linear Conditionally Unbiased Estimators | 766 | ||
The Gauss-Markov Theorem for Multiple Regression | 767 | ||
18.6 Generalized Least Squares | 768 | ||
The GLS Assumptions | 769 | ||
GLS When Ω Is Known | 771 | ||
GLS When Ω Contains Unknown Parameters | 772 | ||
The Zero Conditional Mean Assumption and GLS | 772 | ||
18.7 Instrumental Variables and Generalized Method of Moments Estimation | 774 | ||
The IV Estimator in Matrix Form | 775 | ||
Asymptotic Distribution of the TSLS Estimator | 776 | ||
Properties of TSLS When the Errors Are Homoskedastic | 777 | ||
Generalized Method of Moments Estimation in Linear Models | 780 | ||
APPENDIX 18.1 Summary of Matrix Algebra | 792 | ||
APPENDIX 18.2 Multivariate Distributions | 795 | ||
APPENDIX 18.3 Derivation of the Asymptotic Distribution of ? | 797 | ||
APPENDIX 18.4 Derivations of Exact Distributions of OLS Test Statistics with Normal Errors | 798 | ||
APPENDIX 18.5 Proof of the Gauss-Markov Theorem for Multiple Regression | 799 | ||
APPENDIX 18.6 Proof of Selected Results for IV and GMM Estimation | 800 | ||
Appendix | 803 | ||
References | 811 | ||
Glossary | 817 | ||
Index | 825 | ||
A | 825 | ||
B | 825 | ||
C | 825 | ||
D | 827 | ||
E | 828 | ||
F | 828 | ||
G | 829 | ||
H | 829 | ||
I | 830 | ||
J | 830 | ||
K | 830 | ||
L | 831 | ||
M | 831 | ||
N | 832 | ||
O | 832 | ||
P | 833 | ||
Q | 833 | ||
R | 833 | ||
S | 834 | ||
T | 835 | ||
U | 836 | ||
V | 836 | ||
W | 836 | ||
Z | 836 |