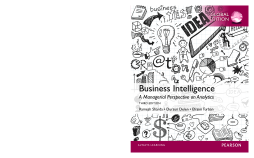
BOOK
Business Intelligence: A Managerial Perspective on Analytics, International Edition
Ramesh Sharda | Dursun Delen | Efraim Turban | David King
(2015)
Additional Information
Book Details
Abstract
For courses on Business Intelligence or Decision Support Systems.
A managerial approach to understanding business intelligence systems.
To help future managers use and understand analytics, Business Intelligence provides students with a solid foundation of BI that is reinforced with hands-on practice.
Table of Contents
Section Title | Page | Action | Price |
---|---|---|---|
Cover | Cover | ||
Title Page | Title | ||
Contents | 7 | ||
Preface \r | 17 | ||
About the Authors\r | 23 | ||
Chapter 1 An Overview of Business\r | 27 | ||
1.1 Opening Vignette: Magpie Sensing | 28 | ||
1.2 Changing Business Environments and Computerized | 30 | ||
The Business Pressures–Responses–Support Model | 30 | ||
1.3 A Framework for Business Intelligence (BI) | 32 | ||
Definitions of BI | 32 | ||
A Brief History of BI | 33 | ||
The Architecture of BI | 34 | ||
The Origins and Drivers of BI | 34 | ||
Application Case 1.1 Sabre Helps Its Clients | 35 | ||
A Multimedia Exercise in Business Intelligence | 36 | ||
1.4 Intelligence Creation, Use, and BI Governance | 37 | ||
A Cyclical Process of Intelligence Creation and Use | 37 | ||
Intelligence and Espionage | 38 | ||
1.5 Transaction Processing Versus Analytic Processing | 39 | ||
1.6 Successful BI Implementation | 40 | ||
The Typical BI User Community | 40 | ||
Appropriate Planning and Alignment with the Business | 40 | ||
Real-Time, On-Demand BI Is Attainable | 41 | ||
Developing or Acquiring BI Systems | 42 | ||
Justification and Cost–Benefit Analysis | 42 | ||
Security and Protection of Privacy | 42 | ||
Integration of Systems and Applications | 42 | ||
1.7 Analytics Overview | 43 | ||
Descriptive Analytics | 44 | ||
Predictive Analytics | 44 | ||
Application Case 1.2 Eliminating Inefficiencies | 45 | ||
Application Case 1.3 Analysis at the Speed | 46 | ||
Prescriptive Analytics | 46 | ||
Application Case 1.4 Moneyball: Analytics in Sports | 47 | ||
Application Case 1.5 Analyzing Athletic Injuries | 48 | ||
Analytics Applied to Different Domains | 48 | ||
Application Case 1.6 Industrial and Commercial Bank of China | 49 | ||
Analytics or Data Science? | 50 | ||
1.8 Brief Introduction to Big Data Analytics | 51 | ||
What Is Big Data? | 51 | ||
Application Case 1.7 Gilt Groupe’s Flash Sales | 52 | ||
1.9 Plan of the Book | 53 | ||
1.10 Resources, Links, and the Teradata University | 55 | ||
Resources and Links | 55 | ||
Vendors, Products, and Demos | 55 | ||
Periodicals | 55 | ||
The Teradata University Network Connection | 55 | ||
The Book’s Web Site | 55 | ||
Key Terms | 56 | ||
Questions for Discussion | 56 | ||
Exercises | 56 | ||
End-of-Chapter Application Case | 57 | ||
References | 59 | ||
Chapter 2 Data Warehousing | 61 | ||
2.1 Opening Vignette: Isle of Capri Casinos | 62 | ||
2.2 Data Warehousing Definitions and Concepts | 64 | ||
What Is a Data Warehouse? | 64 | ||
A Historical Perspective to Data Warehousing | 64 | ||
Characteristics of Data Warehousing | 66 | ||
Data Marts | 67 | ||
Operational Data Stores | 67 | ||
Enterprise Data Warehouses (EDW) | 68 | ||
Application Case 2.1 A Better Data Plan: Well-Established | 68 | ||
Metadata | 69 | ||
2.3 Data Warehousing Process Overview | 70 | ||
Application Case 2.2 Data Warehousing Helps | 71 | ||
2.4 Data Warehousing Architectures | 73 | ||
Alternative Data Warehousing Architectures | 76 | ||
Which Architecture Is the Best? | 79 | ||
2.5 Data Integration and the Extraction, Transformation | 80 | ||
Data Integration | 81 | ||
Application Case 2.3 BP Lubricants Achieves BIGS Success | 81 | ||
Extraction, Transformation, and Load | 83 | ||
2.6 Data Warehouse Development | 85 | ||
Application Case 2.4 Things Go Better with Coke’s | 86 | ||
Data Warehouse Development Approaches | 88 | ||
Application Case 2.5 Starwood Hotels & Resorts Manages | 89 | ||
Additional Data Warehouse Development Considerations | 91 | ||
Representation of Data in Data Warehouse | 92 | ||
Analysis of Data in Data Warehouse | 93 | ||
OLAP Versus OLTP | 93 | ||
OLAP Operations | 94 | ||
2.7 Data Warehousing Implementation Issues | 97 | ||
Application Case 2.6 EDW Helps Connect State | 99 | ||
Massive Data Warehouses and Scalability | 100 | ||
2.8 Real-Time Data Warehousing | 101 | ||
Application Case 2.7 Egg Plc Fries the Competition | 102 | ||
2.9 Data Warehouse Administration, Security | 105 | ||
The Future of Data Warehousing | 107 | ||
2.10 Resources, Links, and the Teradata University Network | 110 | ||
Resources and Links | 110 | ||
Cases | 110 | ||
Vendors, Products, and Demos | 111 | ||
Periodicals | 111 | ||
Additional References | 111 | ||
The Teradata University Network (TUN) Connection | 111 | ||
Key Terms | 112 | ||
Questions for Discussion | 112 | ||
Exercises | 113 | ||
End-of-Chapter Application Case | 114 | ||
References | 116 | ||
Chapter 3 Business Reporting, Visual Analytics, and Business | 119 | ||
3.1 Opening Vignette: Self-Service Reporting | 120 | ||
3.2 Business Reporting Definitions and Concepts | 123 | ||
What Is a Business Report? | 124 | ||
Application Case 3.1 Delta Lloyd Group Ensures Accuracy | 126 | ||
Components of Business Reporting Systems | 127 | ||
Application Case 3.2 Flood of Paper | 128 | ||
3.3 Data and Information Visualization | 129 | ||
Application Case 3.3 Tableau Saves Blastrac | 130 | ||
A Brief History of Data Visualization | 131 | ||
Application Case 3.4 TIBCO Spotfire Provides | 133 | ||
3.4 Different Types of Charts and Graphs | 134 | ||
Basic Charts and Graphs | 134 | ||
Specialized Charts and Graphs | 135 | ||
3.5 The Emergence of Data Visualization and Visual Analytics | 138 | ||
Visual Analytics | 140 | ||
High-Powered Visual Analytics Environments | 140 | ||
3.6 Performance Dashboards | 143 | ||
Dashboard Design | 145 | ||
Application Case 3.5 Dallas Cowboys Score Big | 145 | ||
Application Case 3.6 Saudi Telecom Company Excels | 146 | ||
What to Look For in a Dashboard | 148 | ||
Best Practices in Dashboard Design | 148 | ||
Benchmark Key Performance Indicators | 149 | ||
Wrap the Dashboard Metrics with Contextual | 149 | ||
Validate the Dashboard Design by a Usability | 149 | ||
Prioritize and Rank Alerts/Exceptions Streamed | 149 | ||
Enrich Dashboard with Business-User Comments | 149 | ||
Present Information in Three Different Levels | 149 | ||
Pick the Right Visual Construct Using Dashboard Design Principles | 150 | ||
Provide for Guided Analytics | 150 | ||
3.7 Business Performance Management | 150 | ||
Closed-Loop BPM Cycle | 150 | ||
Application Case 3.7 IBM Cognos Express Helps | 153 | ||
3.8 Performance Measurement | 154 | ||
Key Performance Indicator (KPI) | 154 | ||
Performance Measurement System | 155 | ||
3.9 Balanced Scorecards | 156 | ||
The Four Perspectives | 156 | ||
The Meaning of Balance in BSC | 158 | ||
Dashboards Versus Scorecards | 159 | ||
3.10 Six Sigma as a Performance Measurement System | 159 | ||
The DMAIC Performance Model | 160 | ||
Balanced Scorecard Versus Six Sigma | 160 | ||
Effective Performance Measurement | 160 | ||
Application Case 3.8 Expedia.com’s Customer Satisfaction | 162 | ||
Key Terms | 164 | ||
Questions for Discussion | 165 | ||
Exercises | 165 | ||
End-of-Chapter Application Case | 166 | ||
References | 168 | ||
Chapter 4 Data Mining | 169 | ||
4.1 Opening Vignette: Cabela’s Reels in More | 170 | ||
4.2 Data Mining Concepts and Applications | 172 | ||
Definitions, Characteristics, and Benefits | 173 | ||
Application Case 4.1 Smarter Insurance: Infinity | 174 | ||
How Data Mining Works | 179 | ||
Application Case 4.2 Harnessing Analytics to Combat | 179 | ||
Data Mining Versus Statistics | 183 | ||
4.3 Data Mining Applications | 183 | ||
Application Case 4.3 A Mine on Terrorist Funding | 186 | ||
4.4 Data Mining Process | 187 | ||
Step 1: Business Understanding | 187 | ||
Step 2: Data Understanding | 188 | ||
Step 3: Data Preparation | 188 | ||
Step 4: Model Building | 190 | ||
Step 5: Testing and Evaluation | 192 | ||
Step 6: Deployment | 192 | ||
Application Case 4.4 Data Mining in Cancer Research | 193 | ||
Other Data Mining Standardized Processes | 194 | ||
4.5 Data Mining Methods | 196 | ||
Classification | 196 | ||
Estimating the True Accuracy of Classification Models | 197 | ||
Application Case 4.5 2degrees Gets a 1275 Percent | 203 | ||
Cluster Analysis for Data Mining | 204 | ||
Association Rule Mining | 206 | ||
4.6 Data Mining Software Tools | 210 | ||
Application Case 4.6 Data Mining Goes to Hollywood: | 213 | ||
4.7 Data Mining Privacy Issues, Myths, and Blunders | 216 | ||
Data Mining and Privacy Issues | 216 | ||
Application Case 4.7 Predicting Customer Buying | 217 | ||
Data Mining Myths and Blunders | 218 | ||
Key Terms | 220 | ||
Questions for Discussion | 220 | ||
Exercises | 221 | ||
End-of-Chapter Application Case | 223 | ||
References | 223 | ||
Chapter 5 Text and Web Analytics | 225 | ||
5.1 Opening Vignette: Machine Versus Men | 226 | ||
5.2 Text Analytics and Text Mining Overview | 229 | ||
Application Case 5.1 Text Mining for Patent Analysis | 232 | ||
5.3 Natural Language Processing | 233 | ||
Application Case 5.2 Text Mining Improves Hong | 235 | ||
5.4 Text Mining Applications | 237 | ||
Marketing Applications | 238 | ||
Security Applications | 238 | ||
Application Case 5.3 Mining for Lies | 239 | ||
Biomedical Applications | 241 | ||
Academic Applications | 242 | ||
Application Case 5.4 Text mining and Sentiment | 243 | ||
5.5 Text Mining Process | 244 | ||
Task 1: Establish the Corpus | 245 | ||
Task 2: Create the Term–Document Matrix | 246 | ||
Task 3: Extract the Knowledge | 248 | ||
Application Case 5.5 Research Literature Survey | 250 | ||
5.6 Sentiment Analysis | 253 | ||
Application Case 5.6 Whirlpool Achieves Customer | 255 | ||
Sentiment Analysis Applications | 256 | ||
Sentiment Analysis Process | 258 | ||
Methods for Polarity Identification | 259 | ||
Using a Lexicon | 260 | ||
Using a Collection of Training Documents | 261 | ||
Identifying Semantic Orientation of Sentences | 261 | ||
Identifying Semantic Orientation of Document | 261 | ||
5.7 Web Mining Overview | 262 | ||
Web Content and Web Structure Mining | 264 | ||
5.8 Search Engines | 267 | ||
Anatomy of a Search Engine | 267 | ||
Development Cycle | 267 | ||
Web Crawler | 268 | ||
Document Indexer | 268 | ||
Response Cycle | 269 | ||
Query Analyzer | 269 | ||
Document Matcher/Ranker | 269 | ||
Search Engine Optimization | 270 | ||
Methods for Search Engine Optimization | 271 | ||
Application Case 5.7 Understanding Why Customers | 272 | ||
5.9 Web Usage Mining (Web Analytics) | 274 | ||
Web Analytics Technologies | 274 | ||
Application Case 5.8 Allegro Boosts Online Click-Through | 275 | ||
Web Analytics Metrics | 277 | ||
Web Site Usability | 277 | ||
Traffic Sources | 278 | ||
Visitor Profiles | 279 | ||
Conversion Statistics | 280 | ||
5.10 Social Analytics | 281 | ||
Social Network Analysis | 282 | ||
Social Network Analysis Metrics | 283 | ||
Application Case 5.9 Social Network Analysis Helps | 283 | ||
Connections | 284 | ||
Distributions | 285 | ||
Segmentation | 285 | ||
Social Media Analytics | 285 | ||
How Do People Use Social Media? | 286 | ||
Application Case 5.10 Measuring the Impact of Social | 287 | ||
Measuring the Social Media Impact | 288 | ||
Best Practices in Social Media Analytics | 289 | ||
Application Case 5.11 eHarmony Uses Social | 290 | ||
Key Terms | 293 | ||
Questions for Discussion | 293 | ||
Exercises | 293 | ||
End-of-Chapter Application Case | 294 | ||
References | 296 | ||
Chapter 6 Big Data and Analytics | 299 | ||
6.1 Opening Vignette: Big Data Meets Big Science at CERN | 300 | ||
6.2 Definition of Big Data | 303 | ||
The Vs That Define Big Data | 304 | ||
Application Case 6.1 BigData Analytics Helps | 307 | ||
6.3 Fundamentals of Big Data Analytics | 308 | ||
Business Problems Addressed by Big Data Analytics | 311 | ||
Application Case 6.2 Top 5 Investment Bank Achieves | 312 | ||
6.4 Big Data Technologies | 313 | ||
MapReduce | 313 | ||
Why Use MapReduce? | 315 | ||
Hadoop | 315 | ||
How Does Hadoop Work? | 315 | ||
Hadoop Technical Components | 316 | ||
Hadoop: The Pros and Cons | 317 | ||
NoSQL | 319 | ||
Application Case 6.3 eBay’s Big Data Solution | 320 | ||
6.5 Data Scientist | 321 | ||
Where Do Data Scientists Come From? | 322 | ||
Application Case 6.4 Big Data and Analytics in Politics | 325 | ||
6.6 Big Data and Data Warehousing | 326 | ||
Use Cases for Hadoop | 327 | ||
Use Cases for Data Warehousing | 328 | ||
The Gray Areas (Any One of the Two Would Do the Job) | 329 | ||
Coexistence of Hadoop and Data Warehouse | 329 | ||
6.7 Big Data Vendors | 331 | ||
Application Case 6.5 Dublin City Council Is Leveraging | 333 | ||
Application Case 6.6 Creditreform Boosts | 337 | ||
6.8 Big Data And Stream Analytics | 338 | ||
Stream Analytics Versus Perpetual Analytics | 339 | ||
Critical Event Processing | 340 | ||
Data Stream Mining | 341 | ||
6.9 Applications of Stream Analytics | 341 | ||
e-Commerce | 341 | ||
Telecommunications | 342 | ||
Application Case 6.7 | 342 | ||
Law Enforcement and Cyber Security | 344 | ||
Power Industry | 344 | ||
Financial Services | 344 | ||
Health Sciences | 344 | ||
Government | 345 | ||
Key Terms | 346 | ||
Questions for Discussion | 346 | ||
Exercises | 346 | ||
End-of-Chapter Application Case | 347 | ||
References | 348 | ||
Chapter 7 Business Analytics: Emerging Trends and Future Impacts | 351 | ||
7.1 Opening Vignette: Oklahoma Gas and Electric | 352 | ||
7.2 Location-Based Analytics for Organizations | 353 | ||
Geospatial Analytics | 353 | ||
Application Case 7.1 Great Clips Employs Spatial | 355 | ||
Real-Time Location Intelligence | 357 | ||
Application Case 7.2 Quiznos Targets Customers | 358 | ||
7.3 Analytics Applications for Consumers | 359 | ||
Application Case 7.3 A Life Coach in Your Pocket | 360 | ||
7.4 Recommendation Engines | 362 | ||
7.5 The Web 2.0 Revolution and Online Social Networking | 363 | ||
Representative Characteristics of Web 2.0 | 364 | ||
Social Networking | 364 | ||
A Definition and Basic Information | 365 | ||
Implications of Business and Enterprise Social | 365 | ||
7.6 Cloud Computing and BI | 366 | ||
Service-Oriented DSS | 367 | ||
Data-as-a-Service (DaaS) | 369 | ||
Information-as-a-Service (Information on Demand) | 370 | ||
Analytics-as-a-Service (AaaS)] | 371 | ||
7.7 Impacts of Analytics In Organizations: An Overview | 372 | ||
New Organizational Units | 373 | ||
Restructuring Business Processes and Virtual Teams | 373 | ||
Job Satisfaction | 374 | ||
Job Stress and Anxiety | 374 | ||
Analytics’ Impact on Managers’ Activities and Their Performance | 374 | ||
7.8 Issues of Legality, Privacy, and Ethics | 376 | ||
Legal Issues | 376 | ||
Privacy | 376 | ||
Recent Technology Issues in Privacy and Analytics | 378 | ||
Ethics in Decision Making and Support | 379 | ||
7.9 An Overview of the Analytics Ecosystem | 379 | ||
Analytics Industry Clusters | 380 | ||
Data Infrastructure Providers | 380 | ||
Data Warehouse Industry | 381 | ||
Middleware/BI Platform Industry | 381 | ||
Data Aggregators/Distributors | 382 | ||
Analytics-Focused Software Developers | 382 | ||
Reporting/Analytics | 382 | ||
Predictive Analytics | 382 | ||
Prescriptive Analytics | 383 | ||
Application Developers or System Integrators: | 383 | ||
Analytics User Organizations | 385 | ||
Analytics Industry Analysts and Influencers | 385 | ||
Academic Providers and Certification Agencies | 387 | ||
Key Terms | 389 | ||
Questions for Discussion | 389 | ||
Exercises | 389 | ||
End-of-Chapter Application Case | 390 | ||
References | 391 | ||
Glossary | 393 | ||
Index | 401 | ||
A | 401 | ||
B | 401 | ||
C | 402 | ||
D | 403 | ||
E | 404 | ||
F | 405 | ||
G | 405 | ||
H | 405 | ||
I | 405 | ||
J | 406 | ||
K | 406 | ||
L | 406 | ||
M | 406 | ||
N | 406 | ||
O | 407 | ||
P | 407 | ||
Q | 408 | ||
R | 408 | ||
S | 408 | ||
T | 409 | ||
U | 410 | ||
V | 410 | ||
W | 410 | ||
X | 410 | ||
Y | 410 | ||
Z | 410 |