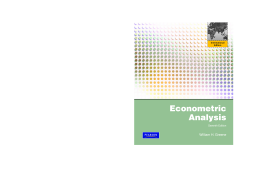
Additional Information
Book Details
Abstract
For first-year graduate courses in Econometrics for Social Scientists.
This title is a Pearson Global Edition. The Editorial team at Pearson has worked closely with educators around the world to include content which is especially relevant to students outside the United States.
This text serves as a bridge between an introduction to the field of econometrics and the professional literature for graduate students in the social sciences, focusing on applied econometrics and theoretical concepts.
Table of Contents
Section Title | Page | Action | Price |
---|---|---|---|
Cover | Cover | ||
Econometric Analysis | 1 | ||
Brief Contents | 4 | ||
Contents | 6 | ||
Examples and Applications | 25 | ||
Preface | 33 | ||
Part I The Linear Regression Model | 41 | ||
Econometrics | 41 | ||
Introduction | 41 | ||
The Paradigm of Econometrics | 41 | ||
The Practice of Econometrics | 43 | ||
Econometric Modeling | 44 | ||
Plan of the Book | 47 | ||
Preliminaries | 49 | ||
Numerical Examples | 49 | ||
Software and Replication | 49 | ||
Notational Conventions | 49 | ||
The Linear Regression Model | 51 | ||
Introduction | 51 | ||
The Linear Regression Model | 52 | ||
Assumptions of the Linear Regression Model | 55 | ||
Linearity of the Regression Model | 55 | ||
Full Rank | 59 | ||
Regression | 60 | ||
Spherical Disturbances | 61 | ||
Data Generating Process for the Regressors | 63 | ||
Normality | 63 | ||
Independence | 64 | ||
Summary and Conclusions | 65 | ||
Least Squares | 66 | ||
Introduction | 66 | ||
Least Squares Regression | 66 | ||
The Least Squares Coefficient Vector | 67 | ||
Application: An Investment Equation | 68 | ||
Algebraic Aspects of the Least Squares Solution | 70 | ||
Projection | 71 | ||
Partitioned Regression and Partial Regression | 72 | ||
Partial Regression and Partial Correlation Coefficients | 76 | ||
Goodness of Fit and the Analysis of Variance | 79 | ||
The Adjusted R-Squared and a Measure of Fit | 82 | ||
R-Squared and the Constant Term in the Model | 84 | ||
Comparing Models | 85 | ||
Linearly Transformed Regression | 86 | ||
Summary and Conclusions | 87 | ||
The Least Squares Estimator | 91 | ||
Introduction | 91 | ||
Motivating Least Squares | 92 | ||
The Population Orthogonality Conditions | 92 | ||
Minimum Mean Squared Error Predictor | 93 | ||
Minimum Variance Linear Unbiased Estimation | 94 | ||
Finite Sample Properties of Least Squares | 94 | ||
Unbiased Estimation | 95 | ||
Bias Caused by Omission of Relevant Variables | 96 | ||
Inclusion of Irrelevant Variables | 98 | ||
The Variance of the Least Squares Estimator | 98 | ||
The Gauss–Markov Theorem | 100 | ||
The Implications of Stochastic Regressors | 100 | ||
Estimating the Variance of the Least Squares Estimator | 101 | ||
The Normality Assumption | 103 | ||
Large Sample Properties of the Least Squares Estimator | 103 | ||
Consistency of the Least Squares Estimator of β | 103 | ||
Asymptotic Normality of the Least Squares Estimator | 105 | ||
Consistency of s2 and the Estimator of Asy. Var[b] | 107 | ||
Asymptotic Distribution of a Function of b: The Delta Method | 108 | ||
Asymptotic Efficiency | 109 | ||
Maximum Likelihood Estimation | 113 | ||
Interval Estimation | 115 | ||
Forming a Confidence Interval for a Coefficient | 116 | ||
Confidence Intervals Based on Large Samples | 118 | ||
Confidence Interval for a Linear Combination of Coefficients: The Oaxaca Decomposition | 119 | ||
Prediction and Forecasting | 120 | ||
Prediction Intervals | 121 | ||
Predicting y When the Regression Model Describes Log y | 121 | ||
Prediction Interval for y When the Regression Model Describes Log y | 123 | ||
Forecasting | 127 | ||
Data Problems | 128 | ||
Multicollinearity | 129 | ||
Pretest Estimation | 131 | ||
Principal Components | 132 | ||
Missing Values and Data Imputation | 134 | ||
Measurement Error | 137 | ||
Outliers and Influential Observations | 139 | ||
Summary and Conclusions | 142 | ||
Hypothesis Tests and Model Selection | 148 | ||
Introduction | 148 | ||
Hypothesis Testing Methodology | 148 | ||
Restrictions and Hypotheses | 149 | ||
Nested Models | 150 | ||
Testing Procedures—Neyman–Pearson Methodology | 151 | ||
Size, Power, and Consistency of a Test | 151 | ||
A Methodological Dilemma: Bayesian versus Classical Testing | 152 | ||
Two Approaches to Testing Hypotheses | 152 | ||
Wald Tests Based on the Distance Measure | 155 | ||
Testing a Hypothesis about a Coefficient | 155 | ||
The F Statistic and the Least Squares Discrepancy | 157 | ||
Testing Restrictions Using the Fit of the Regression | 161 | ||
The Restricted Least Squares Estimator | 161 | ||
The Loss of Fit from Restricted Least Squares | 162 | ||
Testing the Significance of the Regression | 166 | ||
Solving Out the Restrictions and a Caution about Using R2 | 166 | ||
Nonnormal Disturbances and Large-Sample Tests | 167 | ||
Testing Nonlinear Restrictions | 171 | ||
Choosing between Nonnested Models | 174 | ||
Testing Nonnested Hypotheses | 174 | ||
An Encompassing Model | 175 | ||
Comprehensive Approach—The J Test | 176 | ||
A Specification Test | 177 | ||
Model Building—A General to Simple Strategy | 178 | ||
Model Selection Criteria | 179 | ||
Model Selection | 180 | ||
Classical Model Selection | 180 | ||
Bayesian Model Averaging | 181 | ||
Summary and Conclusions | 183 | ||
Functional Form and Structural Change | 189 | ||
Introduction | 189 | ||
Using Binary Variables | 189 | ||
Binary Variables in Regression | 189 | ||
Several Categories | 192 | ||
Several Groupings | 192 | ||
Threshold Effects and Categorical Variables | 194 | ||
Treatment Effects and Difference in Differences Regression | 195 | ||
Nonlinearity in the Variables | 198 | ||
Piecewise Linear Regression | 198 | ||
Functional Forms | 200 | ||
Interaction Effects | 201 | ||
Identifying Nonlinearity | 202 | ||
Intrinsically Linear Models | 205 | ||
Modeling and Testing for a Structural Break | 208 | ||
Different Parameter Vectors | 208 | ||
Insufficient Observations | 209 | ||
Change in a Subset of Coefficients | 210 | ||
Tests of Structural Break with Unequal Variances | 211 | ||
Predictive Test of Model Stability | 214 | ||
Summary and Conclusions | 215 | ||
Nonlinear, Semiparametric, and Nonparametric Regression Models | 221 | ||
Introduction | 221 | ||
Nonlinear Regression Models | 222 | ||
Assumptions of the Nonlinear Regression Model | 222 | ||
The Nonlinear Least Squares Estimator | 224 | ||
Large Sample Properties of the Nonlinear Least Squares Estimator | 226 | ||
Hypothesis Testing and Parametric Restrictions | 229 | ||
Applications | 231 | ||
Computing the Nonlinear Least Squares Estimator | 240 | ||
Median and Quantile Regression | 242 | ||
Least Absolute Deviations Estimation | 243 | ||
Quantile Regression Models | 247 | ||
Partially Linear Regression | 250 | ||
Nonparametric Regression | 252 | ||
Summary and Conclusions | 255 | ||
Endogeneity and Instrumental Variable Estimation | 259 | ||
Introduction | 259 | ||
Assumptions of the Extended Model | 263 | ||
Estimation | 264 | ||
Least Squares | 265 | ||
The Instrumental Variables Estimator | 265 | ||
Motivating the Instrumental Variables Estimator | 267 | ||
Two-Stage Least Squares | 270 | ||
Two Specification Tests | 273 | ||
The Hausman and Wu Specification Tests | 274 | ||
A Test for Overidentification | 278 | ||
Measurement Error | 279 | ||
Least Squares Attenuation | 280 | ||
Instrumental Variables Estimation | 282 | ||
Proxy Variables | 282 | ||
Nonlinear Instrumental Variables Estimation | 286 | ||
Weak Instruments | 289 | ||
Natural Experiments and the Search for Causal Effects | 291 | ||
Summary and Conclusions | 294 | ||
Part II Generalized Regression Model and Equation Systems | 297 | ||
The Generalized Regression Model and Heteroscedasticity | 297 | ||
Introduction | 297 | ||
Inefficient Estimation by Least Squares and Instrumental Variables | 298 | ||
Finite-Sample Properties of Ordinary Least Squares | 299 | ||
Asymptotic Properties of Ordinary Least Squares | 299 | ||
Robust Estimation of Asymptotic Covariance Matrices | 301 | ||
Instrumental Variable Estimation | 302 | ||
Efficient Estimation by Generalized Least Squares | 304 | ||
Generalized Least Squares (GLS) | 304 | ||
Feasible Generalized Least Squares (FGLS) | 306 | ||
Heteroscedasticity and Weighted Least Squares | 308 | ||
Ordinary Least Squares Estimation | 309 | ||
Inefficiency of Ordinary Least Squares | 310 | ||
The Estimated Covariance Matrix of b | 310 | ||
Estimating the Appropriate Covariance Matrix for Ordinary Least Squares | 312 | ||
Testing for Heteroscedasticity | 315 | ||
White’s General Test | 315 | ||
The Breusch–Pagan/Godfrey LM Test | 316 | ||
Weighted Least Squares | 317 | ||
Weighted Least Squares with Known Ω | 318 | ||
Estimation When Ω Contains Unknown Parameters | 319 | ||
Applications | 320 | ||
Multiplicative Heteroscedasticity | 320 | ||
Groupwise Heteroscedasticity | 322 | ||
Summary and Conclusions | 325 | ||
Systems of Equations | 330 | ||
Introduction | 330 | ||
The Seemingly Unrelated Regressions Model | 332 | ||
Generalized Least Squares | 333 | ||
Seemingly Unrelated Regressions with Identical Regressors | 335 | ||
Feasible Generalized Least Squares | 336 | ||
Testing Hypotheses | 336 | ||
A Specification Test for the SUR Model | 337 | ||
The Pooled Model | 339 | ||
Seemingly Unrelated Generalized Regression Models | 344 | ||
Nonlinear Systems of Equations | 345 | ||
Systems of Demand Equations: Singular Systems | 347 | ||
Cobb–Douglas Cost Function | 347 | ||
Flexible Functional Forms: The Translog Cost Function | 350 | ||
Simultaneous Equations Models | 354 | ||
Systems of Equations | 355 | ||
A General Notation for Linear Simultaneous Equations Models | 358 | ||
The Problem of Identification | 361 | ||
Single Equation Estimation and Inference | 366 | ||
System Methods of Estimation | 369 | ||
Testing in the Presence of Weak Instruments | 374 | ||
Summary and Conclusions | 376 | ||
Models for Panel Data | 383 | ||
Introduction | 383 | ||
Panel Data Models | 384 | ||
General Modeling Framework for Analyzing Panel Data | 385 | ||
Model Structures | 386 | ||
Extensions | 387 | ||
Balanced and Unbalanced Panels | 388 | ||
Well-Behaved Panel Data | 388 | ||
The Pooled Regression Model | 389 | ||
Least Squares Estimation of the Pooled Model | 389 | ||
Robust Covariance Matrix Estimation | 390 | ||
Clustering and Stratification | 392 | ||
Robust Estimation Using Group Means | 394 | ||
Estimation with First Differences | 395 | ||
The Within- and Between-Groups Estimators | 397 | ||
The Fixed Effects Model | 399 | ||
Least Squares Estimation | 400 | ||
Small T Asymptotics | 402 | ||
Testing the Significance of the Group Effects | 403 | ||
Fixed Time and Group Effects | 403 | ||
Time-Invariant Variables and Fixed Effects Vector Decomposition | 404 | ||
Random Effects | 410 | ||
Least Squares Estimation | 412 | ||
Generalized Least Squares | 413 | ||
Feasible Generalized Least Squares When Σ Is Unknown | 414 | ||
Testing for Random Effects | 416 | ||
Hausman’s Specification Test for the Random Effects Model | 419 | ||
Extending the Unobserved Effects Model: Mundlak’s Approach | 420 | ||
Extending the Random and Fixed Effects Models: Chamberlain’s Approach | 421 | ||
Nonspherical Disturbances and Robust Covariance Estimation | 425 | ||
Robust Estimation of the Fixed Effects Model | 425 | ||
Heteroscedasticity in the Random Effects Model | 427 | ||
Autocorrelation in Panel Data Models | 428 | ||
Cluster (and Panel) Robust Covariance Matrices for Fixed and Random Effects Estimators | 428 | ||
Spatial Autocorrelation | 429 | ||
Endogeneity | 434 | ||
Hausman and Taylor’s Instrumental Variables Estimator | 434 | ||
Consistent Estimation of Dynamic Panel Data Models: Anderson and Hsiao’s IV Estimator | 438 | ||
Efficient Estimation of Dynamic Panel Data Models—The Arellano/Bond Estimators | 440 | ||
Nonstationary Data and Panel Data Models | 450 | ||
Nonlinear Regression with Panel Data | 451 | ||
A Robust Covariance Matrix for Nonlinear Least Squares | 451 | ||
Fixed Effects | 452 | ||
Random Effects | 454 | ||
Systems of Equations | 455 | ||
Parameter Heterogeneity | 456 | ||
The Random Coefficients Model | 457 | ||
A Hierarchical Linear Model | 460 | ||
Parameter Heterogeneity and Dynamic Panel Data Models | 461 | ||
Summary and Conclusions | 466 | ||
Part III Estimation Methodology | 472 | ||
Estimation Frameworks in Econometrics | 472 | ||
Introduction | 472 | ||
Parametric Estimation and Inference | 474 | ||
Classical Likelihood-Based Estimation | 474 | ||
Modeling Joint Distributions with Copula Functions | 476 | ||
Semiparametric Estimation | 479 | ||
GMM Estimation in Econometrics | 479 | ||
Maximum Empirical Likelihood Estimation | 480 | ||
Least Absolute Deviations Estimation and Quantile Regression | 481 | ||
Kernel Density Methods | 482 | ||
Comparing Parametric and Semiparametric Analyses | 483 | ||
Nonparametric Estimation | 484 | ||
Kernel Density Estimation | 485 | ||
Properties of Estimators | 487 | ||
Statistical Properties of Estimators | 488 | ||
Extremum Estimators | 489 | ||
Assumptions for Asymptotic Properties of Extremum Estimators | 489 | ||
Asymptotic Properties of Estimators | 492 | ||
Testing Hypotheses | 493 | ||
Summary and Conclusions | 494 | ||
Minimum Distance Estimation and the Generalized Method of Moments | 495 | ||
Introduction | 495 | ||
Consistent Estimation: The Method of Moments | 496 | ||
Random Sampling and Estimating the Parameters of Distributions | 497 | ||
Asymptotic Properties of the Method of Moments Estimator | 501 | ||
Summary—The Method of Moments | 503 | ||
Minimum Distance Estimation | 503 | ||
The Generalized Method of Moments (GMM) Estimator | 508 | ||
Estimation Based on Orthogonality Conditions | 508 | ||
Generalizing the Method of Moments | 510 | ||
Properties of the GMM Estimator | 514 | ||
Testing Hypotheses in the GMM Framework | 519 | ||
Testing the Validity of the Moment Restrictions | 519 | ||
GMM Counterparts to the WALD, LM, and LR Tests | 520 | ||
GMM Estimation of Econometric Models | 522 | ||
Single-Equation Linear Models | 522 | ||
Single-Equation Nonlinear Models | 528 | ||
Seemingly Unrelated Regression Models | 531 | ||
Simultaneous Equations Models with Heteroscedasticity | 533 | ||
GMM Estimation of Dynamic Panel Data Models | 536 | ||
Summary and Conclusions | 547 | ||
Maximum Likelihood Estimation | 549 | ||
Introduction | 549 | ||
The Likelihood Function and Identification of the Parameters | 549 | ||
Efficient Estimation: The Principle of Maximum Likelihood | 551 | ||
Properties of Maximum Likelihood Estimators | 553 | ||
Regularity Conditions | 554 | ||
Properties of Regular Densities | 555 | ||
The Likelihood Equation | 557 | ||
The Information Matrix Equality | 557 | ||
Asymptotic Properties of the Maximum Likelihood Estimator | 557 | ||
Consistency | 558 | ||
Asymptotic Normality | 559 | ||
Asymptotic Efficiency | 560 | ||
Invariance | 561 | ||
Conclusion | 561 | ||
Estimating the Asymptotic Variance of the Maximum Likelihood Estimator | 561 | ||
Conditional Likelihoods, Econometric Models, and the GMM Estimator | 563 | ||
Hypothesis and Specification Tests and Fit Measures | 564 | ||
The Likelihood Ratio Test | 566 | ||
The Wald Test | 567 | ||
The Lagrange Multiplier Test | 569 | ||
An Application of the Likelihood-Based Test Procedures | 571 | ||
Comparing Models and Computing Model Fit | 573 | ||
Vuong’s Test and the Kullback–Leibler Information Criterion | 574 | ||
Two-Step Maximum Likelihood Estimation | 576 | ||
Pseudo-Maximum Likelihood Estimation and Robust Asymptotic Covariance Matrices | 582 | ||
Maximum Likelihood and GMM Estimation | 583 | ||
Maximum Likelihood and M Estimation | 583 | ||
Sandwich Estimators | 585 | ||
Cluster Estimators | 586 | ||
Applications of Maximum Likelihood Estimation | 588 | ||
The Normal Linear Regression Model | 588 | ||
The Generalized Regression Model | 592 | ||
Multiplicative Heteroscedasticity | 594 | ||
Autocorrelation | 597 | ||
Seemingly Unrelated Regression Models | 600 | ||
The Pooled Model | 600 | ||
The SUR Model | 602 | ||
Exclusion Restrictions | 602 | ||
Simultaneous Equations Models | 607 | ||
Maximum Likelihood Estimation of Nonlinear Regression Models | 608 | ||
Panel Data Applications | 613 | ||
ML Estimation of the Linear Random Effects Model | 614 | ||
Nested Random Effects | 616 | ||
Random Effects in Nonlinear Models: MLE Using Quadrature | 620 | ||
Fixed Effects in Nonlinear Models: Full MLE | 624 | ||
Latent Class and Finite Mixture Models | 628 | ||
A Finite Mixture Model | 629 | ||
Measured and Unmeasured Heterogeneity | 631 | ||
Predicting Class Membership | 631 | ||
A Conditional Latent Class Model | 632 | ||
Determining the Number of Classes | 634 | ||
A Panel Data Application | 635 | ||
Summary and Conclusions | 638 | ||
Simulation-Based Estimation and Inference and Random Parameter Models | 643 | ||
Introduction | 643 | ||
Random Number Generation | 645 | ||
Generating Pseudo-Random Numbers | 645 | ||
Sampling from a Standard Uniform Population | 646 | ||
Sampling from Continuous Distributions | 647 | ||
Sampling from a Multivariate Normal Population | 648 | ||
Sampling from Discrete Populations | 648 | ||
Simulation-Based Statistical Inference: The Method of Krinsky and Robb | 649 | ||
Bootstrapping Standard Errors and Confidence Intervals | 651 | ||
Monte Carlo Studies | 655 | ||
A Monte Carlo Study: Behavior of a Test Statistic | 657 | ||
A Monte Carlo Study: The Incidental Parameters Problem | 659 | ||
Simulation-Based Estimation | 661 | ||
Random Effects in a Nonlinear Model | 661 | ||
Monte Carlo Integration | 663 | ||
Halton Sequences and Random Draws for Simulation-Based Integration | 665 | ||
Computing Multivariate Normal Probabilities Using the GHK Simulator | 667 | ||
Simulation-Based Estimation of Random Effects Models | 669 | ||
A Random Parameters Linear Regression Model | 674 | ||
Hierarchical Linear Models | 679 | ||
Nonlinear Random Parameter Models | 681 | ||
Individual Parameter Estimates | 682 | ||
Mixed Models and Latent Class Models | 690 | ||
Summary and Conclusions | 693 | ||
Bayesian Estimation and Inference | 695 | ||
Introduction | 695 | ||
Bayes Theorem and the Posterior Density | 696 | ||
Bayesian Analysis of the Classical Regression Model | 698 | ||
Analysis with a Noninformative Prior | 699 | ||
Estimation with an Informative Prior Density | 701 | ||
Bayesian Inference | 704 | ||
Point Estimation | 704 | ||
Interval Estimation | 705 | ||
Hypothesis Testing | 706 | ||
Large-Sample Results | 708 | ||
Posterior Distributions and the Gibbs Sampler | 708 | ||
Application: Binomial Probit Model | 711 | ||
Panel Data Application: Individual Effects Models | 714 | ||
Hierarchical Bayes Estimation of a Random Parameters Model | 716 | ||
Summary and Conclusions | 718 | ||
Part IV Cross Sections, Panel Data, and Microeconometrics | 721 | ||
Discrete Choice | 721 | ||
Introduction | 721 | ||
Models for Binary Outcomes | 723 | ||
Random Utility Models for Individual Choice | 724 | ||
A Latent Regression Model | 726 | ||
Functional Form and Regression | 727 | ||
Estimation and Inference in Binary Choice Models | 730 | ||
Robust Covariance Matrix Estimation | 732 | ||
Marginal Effects and Average Partial Effects | 733 | ||
Average Partial Effects | 736 | ||
Interaction Effects | 739 | ||
Measuring Goodness of Fit | 741 | ||
Hypothesis Tests | 743 | ||
Endogenous Right-Hand-Side Variables in Binary Choice Models | 746 | ||
Endogenous Choice-Based Sampling | 750 | ||
Specification Analysis | 751 | ||
Omitted Variables | 753 | ||
Heteroscedasticity | 754 | ||
Binary Choice Models for Panel Data | 756 | ||
The Pooled Estimator | 757 | ||
Random Effects Models | 758 | ||
Fixed Effects Models | 761 | ||
A Conditional Fixed Effects Estimator | 762 | ||
Mundlak’s Approach, Variable Addition, and Bias Reduction | 767 | ||
Dynamic Binary Choice Models | 769 | ||
A Semiparametric Model for Individual Heterogeneity | 771 | ||
Modeling Parameter Heterogeneity | 773 | ||
Nonresponse, Attrition, and Inverse Probability Weighting | 774 | ||
Bivariate and Multivariate Probit Models | 778 | ||
Maximum Likelihood Estimation | 779 | ||
Testing for Zero Correlation | 782 | ||
Partial Effects | 782 | ||
A Panel Data Model for Bivariate Binary Response | 784 | ||
Endogenous Binary Variable in a Recursive Bivariate Probit Model | 785 | ||
Endogenous Sampling in a Binary Choice Model | 789 | ||
A Multivariate Probit Model | 792 | ||
Summary and Conclusions | 795 | ||
Discrete Choices and Event Counts | 800 | ||
Introduction | 800 | ||
Models for Unordered Multiple Choices | 801 | ||
Random Utility Basis of the Multinomial Logit Model | 801 | ||
The Multinomial Logit Model | 803 | ||
The Conditional Logit Model | 806 | ||
The Independence from Irrelevant Alternatives Assumption | 807 | ||
Nested Logit Models | 808 | ||
The Multinomial Probit Model | 810 | ||
The Mixed Logit Model | 811 | ||
A Generalized Mixed Logit Model | 812 | ||
Application: Conditional Logit Model for Travel Mode Choice | 813 | ||
Estimating Willingness to Pay | 819 | ||
Panel Data and Stated Choice Experiments | 821 | ||
Aggregate Market Share Data—The BLP Random Parameters Model | 822 | ||
Random Utility Models for Ordered Choices | 824 | ||
The Ordered Probit Model | 827 | ||
A Specification Test for the Ordered Choice Model | 831 | ||
Bivariate Ordered Probit Models | 832 | ||
Panel Data Applications | 834 | ||
Ordered Probit Models with Fixed Effects | 834 | ||
Ordered Probit Models with Random Effects | 835 | ||
Extensions of the Ordered Probit Model | 838 | ||
Threshold Models—Generalized Ordered Choice Models | 839 | ||
Thresholds and Heterogeneity—Anchoring Vignettes | 840 | ||
Models for Counts of Events | 842 | ||
The Poisson Regression Model | 843 | ||
Measuring Goodness of Fit | 844 | ||
Testing for Overdispersion | 845 | ||
Heterogeneity and the Negative Binomial Regression Model | 846 | ||
Functional Forms for Count Data Models | 847 | ||
Truncation and Censoring in Models for Counts | 850 | ||
Panel Data Models | 855 | ||
Robust Covariance Matrices for Pooled Estimators | 856 | ||
Fixed Effects | 857 | ||
Random Effects | 858 | ||
Two-Part Models: Zero Inflation and Hurdle Models | 861 | ||
Endogenous Variables and Endogenous Participation | 866 | ||
Summary and Conclusions | 869 | ||
Limited Dependent Variables—Truncation, Censoring, and Sample Selection | 873 | ||
Introduction | 873 | ||
Truncation | 873 | ||
Truncated Distributions | 874 | ||
Moments of Truncated Distributions | 875 | ||
The Truncated Regression Model | 877 | ||
The Stochastic Frontier Model | 879 | ||
Censored Data | 885 | ||
The Censored Normal Distribution | 886 | ||
The Censored Regression (Tobit) Model | 888 | ||
Estimation | 890 | ||
Two-Part Models and Corner Solutions | 892 | ||
Some Issues in Specification | 898 | ||
Heteroscedasticity | 898 | ||
Nonnormality | 899 | ||
Panel Data Applications | 900 | ||
Models for Duration | 901 | ||
Models for Duration Data | 902 | ||
Duration Data | 902 | ||
A Regression-Like Approach: Parametric Models of Duration | 903 | ||
Theoretical Background | 903 | ||
Models of the Hazard Function | 904 | ||
Maximum Likelihood Estimation | 906 | ||
Exogenous Variables | 907 | ||
Heterogeneity | 908 | ||
Nonparametric and Semiparametric Approaches | 909 | ||
Incidental Truncation and Sample Selection | 912 | ||
Incidental Truncation in a Bivariate Distribution | 913 | ||
Regression in a Model of Selection | 913 | ||
Two-Step and Maximum Likelihood Estimation | 916 | ||
Sample Selection in Nonlinear Models | 920 | ||
Panel Data Applications of Sample Selection Models | 923 | ||
Common Effects in Sample Selection Models | 924 | ||
Attrition | 926 | ||
Evaluating Treatment Effects | 928 | ||
Regression Analysis of Treatment Effects | 930 | ||
The Normality Assumption | 932 | ||
Estimating the Effect of Treatment on the Treated | 933 | ||
Propensity Score Matching | 934 | ||
Regression Discontinuity | 937 | ||
Summary and Conclusions | 938 | ||
Part V Time Series and Macroeconometrics | 943 | ||
Serial Correlation | 943 | ||
Introduction | 943 | ||
The Analysis of Time-Series Data | 946 | ||
Disturbance Processes | 949 | ||
Characteristics of Disturbance Processes | 949 | ||
AR(1) Disturbances | 950 | ||
Some Asymptotic Results for Analyzing Time-Series Data | 952 | ||
Convergence of Moments—The Ergodic Theorem | 953 | ||
Convergence to Normality—A Central Limit Theorem | 955 | ||
Least Squares Estimation | 958 | ||
Asymptotic Properties of Least Squares | 958 | ||
Estimating the Variance of the Least Squares Estimator | 959 | ||
GMM Estimation | 961 | ||
Testing for Autocorrelation | 962 | ||
Lagrange Multiplier Test | 962 | ||
Box and Pierce’s Test and Ljung’s Refinement | 962 | ||
The Durbin–Watson Test | 963 | ||
Testing in the Presence of a Lagged Dependent Variable | 963 | ||
Summary of Testing Procedures | 964 | ||
Efficient Estimation When Ω Is Known | 964 | ||
Estimation When Ω Is Known | 966 | ||
AR(1) Disturbances | 966 | ||
Application: Estimation of a Model with Autocorrelation | 967 | ||
Estimation with a Lagged Dependent Variable | 969 | ||
Autoregressive Conditional Heteroscedasticity | 970 | ||
The ARCH(1) Model | 971 | ||
ARCH(q), ARCH-in-Mean, and Generalized ARCH Models | 972 | ||
Maximum Likelihood Estimation of the Garch Model | 974 | ||
Testing for Garch Effects | 976 | ||
Pseudo–Maximum Likelihood Estimation | 977 | ||
Summary and Conclusions | 979 | ||
Nonstationary Data | 982 | ||
Introduction | 982 | ||
Nonstationary Processes and Unit Roots | 982 | ||
Integrated Processes and Differencing | 982 | ||
Random Walks, Trends, and Spurious Regressions | 984 | ||
Tests for Unit Roots in Economic Data | 987 | ||
The Dickey–Fuller Tests | 988 | ||
The KPSS Test of Stationarity | 998 | ||
Cointegration | 999 | ||
Common Trends | 1002 | ||
Error Correction and VAR Representations | 1003 | ||
Testing for Cointegration | 1005 | ||
Estimating Cointegration Relationships | 1007 | ||
Application: German Money Demand | 1007 | ||
Cointegration Analysis and a Long-Run Theoretical Model | 1008 | ||
Testing for Model Instability | 1009 | ||
Nonstationary Panel Data | 1010 | ||
Summary and Conclusions | 1011 | ||
Part VI Appendices | 1013 | ||
Appendix A Matrix Algebra | 1013 | ||
Terminology | 1013 | ||
Algebraic Manipulation of Matrices | 1013 | ||
Equality of Matrices | 1013 | ||
Transposition | 1014 | ||
Matrix Addition | 1014 | ||
Vector Multiplication | 1015 | ||
A Notation for Rows and Columns of a Matrix | 1015 | ||
Matrix Multiplication and Scalar Multiplication | 1015 | ||
Sums of Values | 1017 | ||
A Useful Idempotent Matrix | 1018 | ||
Geometry of Matrices | 1019 | ||
Vector Spaces | 1019 | ||
Linear Combinations of Vectors and Basis Vectors | 1021 | ||
Linear Dependence | 1022 | ||
Subspaces | 1023 | ||
Rank of a Matrix | 1024 | ||
Determinant of a Matrix | 1026 | ||
A Least Squares Problem | 1027 | ||
Solution of a System of Linear Equations | 1029 | ||
Systems of Linear Equations | 1029 | ||
Inverse Matrices | 1030 | ||
Nonhomogeneous Systems of Equations | 1032 | ||
Solving the Least Squares Problem | 1032 | ||
Partitioned Matrices | 1032 | ||
Addition and Multiplication of Partitioned Matrices | 1033 | ||
Determinants of Partitioned Matrices | 1033 | ||
Inverses of Partitioned Matrices | 1033 | ||
Deviations from Means | 1034 | ||
Kronecker Products | 1034 | ||
Characteristic Roots and Vectors | 1035 | ||
The Characteristic Equation | 1035 | ||
Characteristic Vectors | 1036 | ||
General Results for Characteristic Roots and Vectors | 1036 | ||
Diagonalization and Spectral Decomposition of a Matrix | 1037 | ||
Rank of a Matrix | 1037 | ||
Condition Number of a Matrix | 1039 | ||
Trace of a Matrix | 1039 | ||
Determinant of a Matrix | 1040 | ||
Powers of a Matrix | 1040 | ||
Idempotent Matrices | 1042 | ||
Factoring a Matrix | 1042 | ||
The Generalized Inverse of a Matrix | 1043 | ||
Quadratic Forms and Definite Matrices | 1044 | ||
Nonnegative Definite Matrices | 1045 | ||
Idempotent Quadratic Forms | 1046 | ||
Comparing Matrices | 1046 | ||
Calculus and Matrix Algebra | 1047 | ||
Differentiation and the Taylor Series | 1047 | ||
Optimization | 1050 | ||
Constrained Optimization | 1052 | ||
Transformations | 1054 | ||
Appendix B Probability and Distribution Theory | 1055 | ||
Introduction | 1055 | ||
Random Variables | 1055 | ||
Probability Distributions | 1055 | ||
Cumulative Distribution Function | 1056 | ||
Expectations of a Random Variable | 1057 | ||
Some Specific Probability Distributions | 1059 | ||
The Normal Distribution | 1059 | ||
The Chi-Squared, t, and F Distributions | 1061 | ||
Distributions with Large Degrees of Freedom | 1063 | ||
Size Distributions: The Lognormal Distribution | 1064 | ||
The Gamma and Exponential Distributions | 1064 | ||
The Beta Distribution | 1065 | ||
The Logistic Distribution | 1065 | ||
The Wishart Distribution | 1065 | ||
Discrete Random Variables | 1066 | ||
The Distribution of a Function of a Random Variable | 1066 | ||
Representations of a Probability Distribution | 1068 | ||
Joint Distributions | 1070 | ||
Marginal Distributions | 1070 | ||
Expectations in a Joint Distribution | 1071 | ||
Covariance and Correlation | 1071 | ||
Distribution of a Function of Bivariate Random Variables | 1072 | ||
Conditioning in a Bivariate Distribution | 1074 | ||
Regression: The Conditional Mean | 1074 | ||
Conditional Variance | 1075 | ||
Relationships Among Marginal and Conditional Moments | 1075 | ||
The Analysis of Variance | 1077 | ||
The Bivariate Normal Distribution | 1077 | ||
Multivariate Distributions | 1078 | ||
Moments | 1078 | ||
Sets of Linear Functions | 1079 | ||
Nonlinear Functions | 1080 | ||
The Multivariate Normal Distribution | 1081 | ||
Marginal and Conditional Normal Distributions | 1081 | ||
The Classical Normal Linear Regression Model | 1082 | ||
Linear Functions of a Normal Vector | 1083 | ||
Quadratic forms in a Standard Normal Vector | 1083 | ||
The F Distribution | 1085 | ||
A Full Rank Quadratic Form | 1085 | ||
Independence of a Linear and a Quadratic Form | 1086 | ||
Appendix C Estimation and Inference | 1087 | ||
Introduction | 1087 | ||
Samples and Random Sampling | 1088 | ||
Descriptive Statistics | 1088 | ||
Statistics as Estimators—Sampling Distributions | 1091 | ||
Point Estimation of Parameters | 1095 | ||
Estimation in a Finite Sample | 1095 | ||
Efficient Unbiased Estimation | 1098 | ||
Interval Estimation | 1100 | ||
Hypothesis Testing | 1102 | ||
Classical Testing Procedures | 1102 | ||
Tests Based on Confidence Intervals | 1105 | ||
Specification Tests | 1106 | ||
Appendix D Large-Sample Distribution Theory | 1106 | ||
Introduction | 1106 | ||
Large-Sample Distribution Theory | 1107 | ||
Convergence in Probability | 1107 | ||
Other forms of Convergence and Laws of Large Numbers | 1110 | ||
Convergence of Functions | 1113 | ||
Convergence to a Random Variable | 1114 | ||
Convergence in Distribution: Limiting Distributions | 1116 | ||
Central Limit Theorems | 1118 | ||
The Delta Method | 1123 | ||
Asymptotic Distributions | 1124 | ||
Asymptotic Distribution of a Nonlinear Function | 1126 | ||
Asymptotic Expectations | 1127 | ||
Sequences and the Order of a Sequence | 1128 | ||
Appendix E Computation and Optimization | 1129 | ||
Introduction | 1129 | ||
Computation in Econometrics | 1130 | ||
Computing Integrals | 1130 | ||
The Standard Normal Cumulative Distribution Function | 1130 | ||
The Gamma and Related Functions | 1131 | ||
Approximating Integrals by Quadrature | 1132 | ||
Optimization | 1133 | ||
Algorithms | 1135 | ||
Computing Derivatives | 1136 | ||
Gradient Methods | 1137 | ||
Aspects of Maximum Likelihood Estimation | 1140 | ||
Optimization with Constraints | 1141 | ||
Some Practical Considerations | 1142 | ||
The EM Algorithm | 1144 | ||
Examples | 1146 | ||
Function of One Parameter | 1146 | ||
Function of Two Parameters: The Gamma Distribution | 1147 | ||
A Concentrated Log-Likelihood Function | 1148 | ||
Appendix F Data Sets Used in Applications | 1149 | ||
References | 1155 | ||
Combined Author and Subject Index | 1211 |