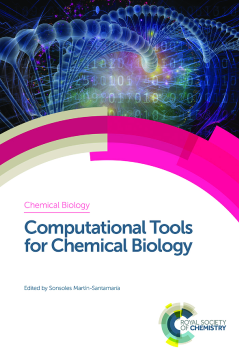
Additional Information
Book Details
Abstract
The rapid development of efficient computational tools has allowed researchers to tackle biological problems and to predict, analyse and monitor, at an atomic level, molecular recognition processes. This book offers a fresh perspective on how computational tools can aid the chemical biology research community and drive new research.
Chapters from internationally renowned leaders in the field introduce concepts and discuss the impact of technological advances in computer hardware and software in explaining and predicting phenomena involving biomolecules, from small molecules to macromolecular systems. Important topics from the understanding of biomolecules to the modification of their functions are addressed, as well as examples of the application of tools in drug discovery, glycobiology, protein design and molecular recognition. Not only are the cutting-the-edge methods addressed, but also their limitations and possible future development.
For anyone wishing to learn how computational chemistry and molecular modelling can provide information not easily accessible through other experimental methods, this book will be a valuable resource. It will be of interest to postgraduates and researchers in the biological and chemical sciences, medicinal and pharmaceutical chemistry, and theoretical chemistry.
Sonsoles Martín-Santamaría completed her PhD in Organic and Pharmaceutical Chemistry in 1998 at the University Complutense of Madrid. Following postdoctoral work at Imperial College London and at the Univercity of Alcalá, she joined the University CEU San Pablo in Madrid as a "Ramón y Cajal" Researcher. Since 2012 she has been the Principal Investigator of the “Computational Chemical Biology” group at the University CEU San Pablo and, since 2014, has been a staff scientist for CIB-CSIC, Madrid.
Table of Contents
Section Title | Page | Action | Price |
---|---|---|---|
Cover | Cover | ||
Preface | v | ||
Contents | vii | ||
Chapter 1 Computational Chemistry and Molecular Modelling Basics | 1 | ||
1.1 Introduction | 1 | ||
1.2 Techniques in Biomolecular Simulations | 2 | ||
1.2.1 Molecular Mechanics and Force Fields | 2 | ||
1.2.2 Basic Simulation Techniques | 4 | ||
1.2.3 Basic Data Analysis | 8 | ||
1.2.4 Software | 11 | ||
1.2.5 Examples | 12 | ||
1.3 Protein Structure Prediction | 13 | ||
1.3.1 Sequence Alignment and Secondary Structure Prediction | 13 | ||
1.3.2 Comparative Modelling Approaches | 15 | ||
1.3.3 Function Prediction | 18 | ||
1.3.4 Analysing the Quality of the Modelled Structure | 18 | ||
1.3.5 Software and Web Based Servers | 19 | ||
1.4 Computer-based Drug Design | 20 | ||
1.4.1 Pre-requisites for SBDD—Sampling Algorithms and Scoring Functions | 20 | ||
1.4.2 Structure Based Drug Design (SBDD) | 24 | ||
1.4.3 Ligand Based Drug Design (LBDD) | 26 | ||
1.4.4 Pharmacophores | 26 | ||
1.4.5 Compound Optimisation | 27 | ||
1.4.6 Software and Web Based Servers | 29 | ||
Acknowledgments | 30 | ||
References | 30 | ||
Chapter 2 Molecular Dynamics Computer Simulations of Biological Systems | 39 | ||
2.1 Introduction | 39 | ||
2.2 The Basics of Molecular Dynamics | 40 | ||
2.2.1 Force Fields for Biomolecular Simulations | 41 | ||
2.2.2 Multiscale Modelling | 44 | ||
2.2.3 Advanced Force Fields | 45 | ||
2.3 Extracting the Information from MD | 46 | ||
2.3.1 Free Energy Difference Between Two States | 47 | ||
2.3.2 Enhanced Configurational Sampling | 47 | ||
2.3.3 Simulating Rare Events | 49 | ||
2.3.4 Computing Elastic Properties in Biomolecular Simulations | 50 | ||
2.4 MD Simulation vs. Experiment | 54 | ||
2.4.1 NMR and MD: Structure and Dynamics | 55 | ||
2.4.2 Structure of Biomolecules and Diffraction: Solving the Phase Problem with MD | 57 | ||
2.5 Future Directions | 58 | ||
2.6 Conclusion | 61 | ||
Acknowledgments | 63 | ||
References | 63 | ||
Chapter 3 Designing Chemical Tools with Computational Chemistry | 69 | ||
3.1 Introduction | 69 | ||
3.2 Structure Based Approaches for Chemical Biology | 72 | ||
3.3 Structural Dynamics as a Source of Novel Chemical Tools | 74 | ||
3.4 Combining Bioinformatics, Chemoinformatics and Structural Information to Explore Protein Functions | 79 | ||
3.5 Deep Networks and Big Data in the Discovery of New Drugs and Chemical Tools | 81 | ||
3.6 Conclusions and Perspectives | 83 | ||
References | 84 | ||
Chapter 4 Computational Design of Protein Function | 87 | ||
4.1 Introduction | 87 | ||
4.2 The ‘Inside-out' Design Protocol | 89 | ||
4.2.1 Description of the Method | 89 | ||
4.2.2 Enzymes Designed Though the ‘Inside-out' Approach: Kemp Eliminases | 92 | ||
4.3 QM/MM Approaches to Enzyme Design | 94 | ||
4.3.1 Description of the Methods | 94 | ||
4.3.2 Engineered Butyrylcholinesterase for Cocaine Detoxification | 96 | ||
4.3.3 Electron Transfer Reactions Catalysed by Metalloproteins | 99 | ||
4.4 Summary and Outlook | 101 | ||
Acknowledgments | 102 | ||
References | 102 | ||
Chapter 5 Computational Enzymology: Modelling Biological Catalysts | 108 | ||
5.1 Introduction | 108 | ||
5.2 General Framework | 109 | ||
5.2.1 The Transition State and the Energy Barrier | 109 | ||
5.2.2 Quantum Mechanics Molecular Mechanics (QM/MM) Methods | 110 | ||
5.3 Building the Model(s) of the Enzyme-Substrate Complex(es) | 114 | ||
5.3.1 Starting Structure and System Setup | 114 | ||
5.3.2 Molecular Dynamics Simulations | 114 | ||
5.4 Potential Energy Methods | 115 | ||
5.4.1 Reaction Path Calculation | 115 | ||
5.4.2 Transition State Localisation | 117 | ||
5.4.3 Analysis | 118 | ||
5.5 Free Energy Simulations | 122 | ||
5.5.1 Umbrella Sampling Method | 123 | ||
5.5.2 Free Energy Perturbation Theory | 127 | ||
5.5.3 String Method: Minimum Free Energy Paths | 132 | ||
5.6 Calculation of the Reaction Rate Constant | 136 | ||
5.6.1 Ensemble-averaged Variational Transition State Theory with Multi-dimensional Tunnelling (EA-VTST/MT) | 136 | ||
5.7 Further Considerations about the Relationship between the Activation Free Energy and the Extension of the Sampling of the Configurational Space | 139 | ||
References | 141 | ||
Chapter 6 Computational Chemistry Tools in Glycobiology: Modelling of Carbohydrate-Protein Interactions | 145 | ||
6.1 What are the Carbohydrates? | 145 | ||
6.2 From Mono to Polysaccharides: An Overview of the Increasing Complexity | 147 | ||
6.2.1 Monosaccharides | 147 | ||
6.2.2 Disaccharides: The Glycosidic Linkage and the Exo-anomeric Effect | 148 | ||
6.2.3 Studying the Conformations Around the Glycosidic Linkage | 149 | ||
6.2.4 Oligosaccharides | 149 | ||
6.2.5 N-glycans | 150 | ||
6.2.6 Polysaccharides | 150 | ||
6.3 Computational Methodologies for the Study of Carbohydrates | 151 | ||
6.4 Force Fields for Carbohydrates | 153 | ||
6.5 Modelling Carbohydrate-Protein Interactions | 155 | ||
6.6 Conclusions | 159 | ||
Acknowledgments | 159 | ||
References | 159 | ||
Chapter 7 Molecular Modelling of Nucleic Acids | 165 | ||
7.1 Introduction | 165 | ||
7.2 QM Methods | 166 | ||
7.2.1 Basic Methodological Description | 166 | ||
7.2.2 Examples of Use | 167 | ||
7.3 Hybrid QM/MM | 167 | ||
7.3.1 Basic Methodological Description | 167 | ||
7.3.2 Examples of Use | 168 | ||
7.4 Atomistic Force-field Simulations | 170 | ||
7.4.1 Basic Methodological Description | 170 | ||
7.4.2 Force-field Refinements | 172 | ||
7.4.3 Recent Examples of Force-field Studies of Nucleic Acids | 175 | ||
7.5 The Coarse-grain Approach | 177 | ||
7.5.1 Basic Methodological Description | 178 | ||
7.5.2 Coarse-grained Methods for Predicting RNA Structures | 182 | ||
7.6 Mesoscopic Models | 184 | ||
7.6.1 Basic Methodological Description | 185 | ||
7.6.2 Nucleosome Fibre Simulations | 186 | ||
7.6.3 Chromosome Simulations | 187 | ||
7.7 Conclusions | 188 | ||
Acknowledgments | 188 | ||
References | 189 | ||
Chapter 8 Uncovering GPCR and G Protein Function by Protein Structure Network Analysis | 198 | ||
8.1 Introduction | 198 | ||
8.2 Experimental | 201 | ||
8.2.1 Materials | 201 | ||
8.2.2 Methods | 201 | ||
8.3 Results and Discussion | 205 | ||
8.3.1 Modelling Allosteric Communication in GPCRs | 205 | ||
8.3.2 Modelling Allosteric Communication in G Proteins | 213 | ||
8.4 Conclusions | 216 | ||
Acknowledgments | 216 | ||
References | 217 | ||
Chapter 9 Current Challenges in the Computational Modelling of Molecular Recognition Processes | 221 | ||
9.1 Modelling the Dynamics of the Proteins | 221 | ||
9.2 Three-dimensional Structure Prediction and Homology Modelling | 224 | ||
9.3 Modelling of Protein-Protein Interactions | 225 | ||
9.4 Prediction of Protein-Protein Interactions: Docking | 226 | ||
9.5 Computational Studies of Complex Protein Systems | 229 | ||
9.6 Computational Modelling of Nanostructures | 232 | ||
9.6.1 Modelling of Gold Nanoparticles | 233 | ||
9.6.2 Modelling of Nanowires | 234 | ||
9.6.3 Modelling of Nanotubes | 235 | ||
9.6.4 Modelling of Nanomachines | 236 | ||
9.7 Models of Signalling Networks | 237 | ||
Acknowledgments | 240 | ||
References | 240 | ||
Chapter 10 Novel Insights into Membrane Transport from Computational Methodologies | 247 | ||
10.1 Introduction | 247 | ||
10.2 Computational Methods | 248 | ||
10.3 Unassisted Diffusion Across Lipid Bilayers | 252 | ||
10.4 Passive Transport by Ion Channels | 255 | ||
10.5 Facilitated Diffusion by Transporters | 259 | ||
10.6 Signalling via Receptors | 264 | ||
10.7 Conclusions | 268 | ||
Acknowledgments | 268 | ||
References | 269 | ||
Chapter 11 Application of Molecular Modelling to Speed-up the Lead Discovery Process | 281 | ||
11.1 Introduction | 281 | ||
11.1.1 The ‘Pharmaceutical Crisis' | 281 | ||
11.1.2 The Drug Discovery Process | 282 | ||
11.1.3 The Contribution of Molecular Modelling to Improve Drug Discovery | 284 | ||
11.1.4 Quantum and Molecular Mechanics in Drug Design | 285 | ||
11.1.5 An Introduction to Structure- and Ligand-based Molecular Modelling | 285 | ||
11.2 Structure-based Molecular Modelling | 286 | ||
11.2.1 Sources of 3D Structures | 286 | ||
11.2.2 Docking | 289 | ||
11.2.3 De Novo Drug Design | 291 | ||
11.2.4 Introducing Dynamics | 293 | ||
11.3 Ligand-based Molecular Modelling | 296 | ||
11.3.1 Similarity Searching: Same Shape, Same Activity | 297 | ||
11.3.2 Pharmacophore Modelling | 299 | ||
11.3.3 QSAR | 300 | ||
11.3.4 Use of In Silico Ligand-based Approaches: A Practical Case Study on Antitubercular Agents | 304 | ||
11.4 Conclusions | 305 | ||
Abbreviations | 306 | ||
Acknowledgments | 307 | ||
References | 307 | ||
Chapter 12 Molecular Modelling and Simulations Applied to Challenging Drug Discovery Targets | 317 | ||
12.1 Introduction | 317 | ||
12.2 Deciphering Metalloenzyme Catalysis via Computations | 319 | ||
12.2.1 Ribonuclease H | 319 | ||
12.2.2 Epoxide hydrolase | 321 | ||
12.3 Simulating Membrane Proteins | 323 | ||
12.3.1 Membrane Enzymes: The Case of FAAH | 323 | ||
12.3.2 Ion Channels: The Case of the Kv11.1 Channel | 324 | ||
12.3.3 GPCR: The Case of the Human Adenosine Receptor A2A Embedded in Neuronal-like Membrane | 328 | ||
12.4 Tackling Target Flexibility Through Simulations | 331 | ||
12.4.1 Lactate Dehydrogenase | 331 | ||
12.4.2 Intrinsically Disordered Proteins | 333 | ||
12.4.3 Targeting RNA in Trinucleotide Repeats Diseases | 335 | ||
12.5 Conclusions | 338 | ||
References | 338 | ||
Chapter 13 The Polypharmacology Gap Between Chemical Biology and Drug Discovery | 349 | ||
13.1 Introduction: Chemical Biology and the Limits of Reductionism | 349 | ||
13.1.1 Polypharmacology in Drug Discovery | 349 | ||
13.1.2 Selectivity in Chemical Biology | 351 | ||
13.2 Systems Pharmacology: Databases and Methods | 353 | ||
13.2.1 Databases of Chemical, Biological and Pharmacological Data | 353 | ||
13.2.2 Computational Methods to Predict Polypharmacology | 354 | ||
13.3 Case Study 1: The Impact of Chemical Probe Polypharmacology on PARP Drug Discovery | 355 | ||
13.3.1 The History of PARP Biology: From Probes to Drugs | 355 | ||
13.3.2 PJ34: A PARP Chemical Tool Binding to PIM Kinases | 357 | ||
13.3.3 Differential Off-target Kinase Pharmacology Between Clinical PARP Inhibitors | 360 | ||
13.4 Case Study 2: Distant Off-target Pharmacology among MLP Chemical Probes | 363 | ||
13.5 Conclusions and Outlook | 365 | ||
Acknowledgments | 366 | ||
References | 366 | ||
Subject Index | 371 |