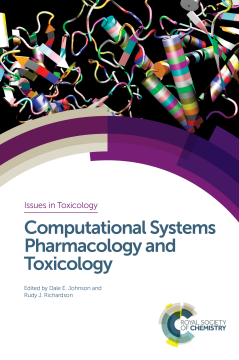
Additional Information
Book Details
Abstract
The network approaches of systems pharmacology and toxicology serve as early predictors of the most relevant screening approach to pursue both in drug discovery and development and ecotoxicological assessments. Computational approaches have the potential to improve toxicological experimental design, enable more rapid drug efficacy and safety testing and also reduce the number of animals used in experimentation. Rapid advances in availability of computing technology hold tremendous promise for advancing applied and basic science and increasing the efficiency of risk assessment.
This book provides an understanding of the basic principles of computational toxicology and the current methods of predictive toxicology using chemical structures, toxicity-related databases, in silico chemical-protein docking, and biological pathway tools. The book begins with an introduction to systems pharmacology and toxicology and computational tools followed by a section exploring modelling adverse outcomes and events. The second part of the book covers the discovery of protein targets and the characterisation of toxicant-protein interactions. Final chapters include case studies and additionally discuss interactions between phytochemicals and Western therapeutics.
This book will be useful for scientists involved in environmental research and risk assessment. It will be a valuable resource for postgraduate students and researchers wishing to learn about key methods used in studying biological targets both from a toxicity and pharmacological activity standpoint.
Dr Dale E. Johnson is currently an Adjunct Professor in Molecular Toxicology at UC Berkeley and an Adjunct Professor in Environmental Health Sciences at the University of Michigan. He teaches computational toxicology, drug discovery & development, and lectures in pharmacogenomics. He is also President and CEO of Emiliem, Inc. and CEO of Elara Bioscience LLC, a software company. He also consults for biopharmaceutical companies and investment firms. Dr Johnson has over 30 years’ experience as a research and development scientist, manager, executive, and entrepreneur in the biopharmaceutical field working in large pharmaceutical and biotech companies and several start-ups. Prior to Emiliem, he served as VP Drug Assessment & Development and VP Preclinical Development at Chiron Corporation and previously worked at Hoechst-Roussel Pharmaceuticals, International Research and Development Corp., Medical Research Division of American Cyanamid, Eos Biotechnology and Ddplatform. Dr Rudy J. Richardson is currently the Dow Professor of Toxicology at the University of Michigan. He also holds a joint appointment in the Neurology Department. Sabbatical leaves at Warner-Lambert/Parke-Davis (now Pfizer) in Ann Arbor and the University of Padua in Italy. Dr Richardson has served several times as the Director of the Toxicology Program, and currently he serves as Graduate Chair of the EHS Department. His research interests include computational toxicology, biomarkers and biosensors of exposure and disease, mechanisms of neurodegenerative disease, chemistry and toxicology of organophosphorus and organic nitro compounds, and interactions of toxicants with target macromolecules.
Table of Contents
Section Title | Page | Action | Price |
---|---|---|---|
Cover | Cover | ||
Computational Systems Pharmacology and Toxicology | i | ||
Preface | vii | ||
Contents | ix | ||
Chapter 1 - Systems Biology Approaches in Pharmacology and Toxicology | 1 | ||
1.1 Introduction | 1 | ||
1.2 Systems Toxicology | 2 | ||
1.3 Chemical Toxicities | 3 | ||
1.3.1 Single-Target Toxicity Concepts | 3 | ||
1.3.2 Toxicological Profiling for Potential Adverse Reactions | 5 | ||
1.3.3 Toxicological Concepts for Safer Chemical Design | 6 | ||
1.3.4 Biomarkers | 8 | ||
1.4 Environmental Toxicology | 9 | ||
1.4.1 Adverse Outcome Pathway | 9 | ||
1.4.2 Expanding Exposure Concepts | 10 | ||
1.4.3 Exposome | 11 | ||
1.5 Systems and Network Pharmacology | 12 | ||
1.5.1 Secondary Pharmacology and Off-Target Effects | 13 | ||
1.5.2 Prediction of Potential Adverse Effects | 14 | ||
1.6 Conclusions | 14 | ||
References | 14 | ||
Chapter 2 - Databases Facilitating Systems Biology Approaches in Toxicology | 19 | ||
2.1 Introduction | 19 | ||
2.2 Categorized Lists of Databases for Systems Toxicology | 21 | ||
2.2.1 TOXNET Databases (Including Those with Direct Links from TOXNET) | 21 | ||
2.2.2 US EPA Chemical Toxicity Databases | 24 | ||
2.2.3 National Toxicology Program Databases | 24 | ||
2.2.4 Additional Toxicity Databases | 25 | ||
2.2.5 Chemical–Gene–Protein Databases | 26 | ||
2.2.6 Pathway-Network Databases | 27 | ||
2.2.7 Chemistry, Structural Alert, and QSAR Databases and Tools | 28 | ||
2.2.8 Drug and Drug Target Databases | 30 | ||
2.3 Websites with Extensive Links to Databases and Tools | 31 | ||
2.4 Conclusions | 31 | ||
References | 32 | ||
Chapter 3 - Tools for Green Molecular Design to Reduce Toxicological Risk | 36 | ||
3.1 Introduction | 37 | ||
3.2 Physiochemical, Genotoxicity, and Blood–Brain Barrier Passage Properties of Chemicals | 38 | ||
3.3 Tools for Green Molecular Design | 39 | ||
3.3.1 Expert Systems | 39 | ||
3.3.2 Decision Trees | 44 | ||
3.3.3 QSAR Tools | 44 | ||
3.3.4 Representative Tools | 45 | ||
3.3.4.1 ACD Percepta (www.acdlabs.com) | 45 | ||
3.3.4.2 ADMET Predictor (www.simulations-plus.com) | 46 | ||
3.3.4.3 Medchem Designer | 46 | ||
3.3.4.4 Derek and Meteor Nexus from Lhasa Limited (www.lhasalimited.org) | 47 | ||
3.3.4.5 Qikprop (www.schrodinger.com/QikProp) | 48 | ||
3.3.4.6 OECD QSAR Toolbox | 48 | ||
3.3.4.7 Toxtree (http://toxtree.sourceforge.net/) | 48 | ||
3.3.4.8 Chemaxon Suite (Marvin Sketch and Metabolizer) (www.chemaxon.com/) | 49 | ||
3.3.4.9 Chemicalize (www.chemicalize.com) | 49 | ||
3.3.4.10 AIM (Analog Identification Methodology) (http://www.epa.gov/tsca-screening-tools/analog-identification-methodology-aim-t... | 49 | ||
3.3.4.11 Chemspider (www.chemspider.com) | 49 | ||
3.3.4.12 Mobyle@RPBS (http://mobyle.rpbs.univ-paris-diderot.fr) | 49 | ||
3.4 Case Study | 50 | ||
3.5 The Design of Ideal Tools for Chemists | 51 | ||
3.6 Conclusions | 54 | ||
References | 54 | ||
Chapter 4 - Linking Environmental Exposure to Toxicity† | 60 | ||
4.1 Introduction | 60 | ||
4.2 The AOP Framework: An Organizing Principle for Toxicological Data | 64 | ||
4.2.1 AOP Knowledge Management | 67 | ||
4.2.2 Phases of AOP Development | 68 | ||
4.2.3 Data Resources for AOP Development | 70 | ||
4.3 Environmental Exposure and Pharmacokinetic Considerations for Adverse Outcome Development | 73 | ||
4.4 The AEP Framework: An Organizing Principle for Exposure Data | 75 | ||
4.4.1 Data resources for AEP development | 79 | ||
4.5 AEP–AOP Integration for Linking Toxicity to Exposure: Applications of the AOP and AEP Frameworks for Risk Assessments and Che... | 80 | ||
4.6 Conclusions and Future Directions | 82 | ||
References | 83 | ||
Chapter 5 - Linking Drug or Phytochemical Exposure to Toxicity | 89 | ||
5.1 Introduction | 89 | ||
5.2 Pharmacokinetic and Toxicokinetic Models | 91 | ||
5.2.1 Structural Models | 91 | ||
5.2.1.1 Compartmental Models | 92 | ||
5.2.1.2 Physiological Models | 96 | ||
5.2.2 Variance Models | 98 | ||
5.2.2.1 Other Modeling Methodologies | 101 | ||
5.3 PK/PD Relationships | 102 | ||
5.3.1 Mathematical Description of Pharmacodynamic Effects | 104 | ||
5.3.1.1 Direct Effects and Effect Compartments | 106 | ||
5.3.1.2 Indirect Effects | 107 | ||
5.3.2 Combined PK/PD and TK/TD Modeling | 109 | ||
5.3.3 Modeling Pharmacodynamics in the Absence of Pharmacokinetic Data: K-PD Models | 110 | ||
5.4 Modeling Drug Interactions with Phytochemicals | 111 | ||
5.4.1 Inhibition of Metabolism | 112 | ||
5.4.2 Induction of Metabolism | 113 | ||
5.4.3 Enhancement of Absorption | 114 | ||
5.4.4 Inhibition of Absorption | 115 | ||
5.4.5 Modeling of Pharmacodynamic Interactions | 115 | ||
5.5 Conclusions | 116 | ||
References | 116 | ||
Chapter 6 - Chemical Similarity, Shape Matching and QSAR | 120 | ||
6.1 Introduction | 120 | ||
6.2 Molecular Similarity, Chemical Spaces and Activity Landscapes | 121 | ||
6.2.1 Molecular Similarity: Concept and Definitions | 121 | ||
6.2.1.1 Molecular Similarity Concept | 121 | ||
6.2.1.2 Applicability of Molecular Similarity Measures | 122 | ||
6.2.1.3 Structure Representations for Molecular Similarity Analysis | 122 | ||
6.2.1.4 Molecular Similarity Functions | 125 | ||
6.2.2 Chemical Spaces and Activity Landscapes | 131 | ||
6.2.3 Applications of Molecular Similarity Analysis | 138 | ||
6.2.3.1 Similarity-Based Virtual Screening | 138 | ||
6.2.3.2 Activity Prediction | 139 | ||
6.2.3.3 Clustering, Networks and Diversity | 141 | ||
6.3 Quantitative Structure–Activity/Property Relationships (QSAR/QSPR) | 142 | ||
6.3.1 Congeneric Series and Consistent Mechanisms | 143 | ||
6.3.2 Diverse Series and Big Data | 148 | ||
6.3.2.1 Prediction of ADMET Properties | 150 | ||
6.3.2.2 Prediction of Potential Drug Targets | 152 | ||
6.3.2.3 Prediction of Activity Towards Individual Targets | 152 | ||
6.3.2.4 Prediction of Physico-Chemical Properties | 153 | ||
6.3.2.5 Open Web-Based QSAR/QSPR Services | 153 | ||
6.4 Conclusion | 153 | ||
Acknowledgements | 154 | ||
References | 154 | ||
Chapter 7 - In silico Chemical–Protein Docking and Molecular Dynamics | 174 | ||
7.1 Introduction | 174 | ||
7.2 Molecular Docking: Overview and Applications | 175 | ||
7.2.1 Genetic Algorithms | 177 | ||
7.2.2 Monte Carlo Procedure | 177 | ||
7.2.3 Matching Algorithms | 177 | ||
7.3 Scoring Ligand Poses | 178 | ||
7.4 Inverse Docking | 179 | ||
7.5 Case Study: Using In silico Docking to Investigate Interactions of 1,3-Dinitrobenzene with Adenosine Deaminase | 179 | ||
7.6 Case Study: Using In silico Docking to Assess Binding of Bisphenol-A to Estrogen-Related Receptor-γ | 180 | ||
7.7 Molecular Dynamics | 182 | ||
7.7.1 Running MD Simulations | 182 | ||
7.7.2 Analysis of MD Trajectories | 183 | ||
7.7.3 Case Study: Gaining Insights into the Conformational Dynamics of Human Neuropathy Target Esterase via MD Simulations of its... | 185 | ||
References | 187 | ||
Chapter 8 - Computational Tools for Chemical Toxicity Testing and Risk Assessment Under the Framework of Adverse Outcome Pathways | 191 | ||
8.1 Introduction | 191 | ||
8.2 The AOP Concept | 193 | ||
8.3 Quantitative Methods in Traditional Apical Endpoints Testing | 194 | ||
8.4 PBPK Modeling and In vitro to In vivo Extrapolation | 196 | ||
8.5 SAR Modeling | 197 | ||
8.6 Computational Modeling of Toxicity Pathways | 198 | ||
8.6.1 Concept of Toxicity Pathways | 198 | ||
8.6.2 Purpose of Modeling Toxicity Pathways | 199 | ||
8.6.3 How to Model Toxicity Pathways | 200 | ||
8.6.4 Case Studies | 203 | ||
8.6.5 Education on Computational Toxicology | 205 | ||
8.6.6 Pathway Modeling Software Tools | 205 | ||
Acknowledgements | 206 | ||
References | 206 | ||
Chapter 9 - In silico Toxicology: An Overview of Toxicity Databases, Prediction Methodologies, and Expert Review | 209 | ||
9.1 Introduction | 209 | ||
9.2 Toxicity Databases | 215 | ||
9.2.1 Overview | 215 | ||
9.2.2 Database Organization | 216 | ||
9.2.3 Genetic Toxicity and Carcinogenicity | 217 | ||
9.2.4 Reproductive and Developmental Toxicity | 219 | ||
9.2.5 Acute and Repeated Dose Toxicity | 220 | ||
9.3 In silico Methodologies | 221 | ||
9.3.1 Overview | 221 | ||
9.3.2 Expert Alerts | 221 | ||
9.3.3 QSARs | 223 | ||
9.3.4 Read-Across | 226 | ||
9.4 Expert Reviews | 227 | ||
9.4.1 Assessing Experimental Data | 227 | ||
9.4.2 Drawing Conclusions from Multiple Systems | 228 | ||
9.4.3 Reviews Accepting or Refuting An In silico Result | 230 | ||
9.4.4 Documenting In silico Results | 233 | ||
9.5 Conclusions | 233 | ||
Acknowledgements | 236 | ||
References | 236 | ||
Chapter 10 - Data Sources for Herbal and Traditional Medicines | 243 | ||
10.1 Introduction | 243 | ||
10.2 TCM Databases | 244 | ||
10.2.1 Chem-TCM (Chemical Database of Traditional Chinese Medicine) | 244 | ||
10.2.2 HIT (Linking Herbal Active Ingredients to Targets) | 244 | ||
10.2.3 TCMSP (Traditional Chinese Medicine Systems Pharmacology Database and Analysis Platform) | 246 | ||
10.2.4 TCMGeneDIT (A Database for Associated TCM, Gene and Disease Information Using Text Mining) | 248 | ||
10.2.5 TCMID (Traditional Chinese Medicine Integrative Database for Herb Molecular Mechanism Analysis) | 249 | ||
10.2.6 TTD (Therapeutic Target Database) | 251 | ||
10.3 Omics Data in TCM | 253 | ||
10.3.1 Genomics in TCM | 253 | ||
10.3.2 Transcriptomics in TCM | 254 | ||
10.3.3 Proteomics in TCM | 256 | ||
10.3.4 Metabonomics in TCM | 256 | ||
10.4 Summary | 257 | ||
Acknowledgements | 257 | ||
References | 257 | ||
Chapter 11 - Network Pharmacology Research Approaches for Chinese Herbal Medicines | 261 | ||
11.1 Introduction | 261 | ||
11.1.1 Modernization of TCM | 263 | ||
11.1.2 Concept of Network Pharmacology | 263 | ||
11.2 Network Pharmacology in TCM Research | 265 | ||
11.3 Network Pharmacology in the Understanding of Herb–Drug Interactions | 268 | ||
11.4 Pharmacogenomics in TCM | 269 | ||
11.5 TCMs in Clinical Trials | 271 | ||
11.6 The Future of Network Pharmacology in Traditional Medicine | 273 | ||
11.7 Conclusion | 274 | ||
References | 274 | ||
Chapter 12 - Chemical–Disease Category Linkage (CDCL): Computational Methods Linking Traditional Chinese Medicines and Western Therapeutics | 279 | ||
12.1 Introduction | 279 | ||
12.1.1 Databases for CDCL Information and Study | 281 | ||
12.1.1.1 TCM and Chemical Constituents | 282 | ||
12.1.1.2 TCM Classification and Systems Approach | 282 | ||
12.1.1.3 Western Therapeutics | 283 | ||
12.1.1.4 Therapeutic Targets and Protein Interactions | 283 | ||
12.1.1.5 Pathway Analysis | 283 | ||
12.1.2 TCM Classifications | 283 | ||
12.1.3 Active Ingredients in Herbs | 286 | ||
12.1.3.1 Wind Cold | 286 | ||
12.1.3.2 Heat (Blood) | 286 | ||
12.1.3.3 Tonify Qi | 287 | ||
12.1.3.4 Tonify Blood | 287 | ||
12.2 Open Access Tools for CDCL Informatics | 287 | ||
12.3 Computational CDCL Studies with Commercial Tools | 289 | ||
12.4 Herb–Drug Interactions | 292 | ||
12.4.1 Pharmacokinetic Interactions | 292 | ||
12.4.2 Pharmacogenomic-Related Interactions | 294 | ||
12.5 Combination Therapies and Future Directions | 294 | ||
12.6 Conclusions | 295 | ||
References | 296 | ||
Chapter 13 - Educational Programs for Computational Toxicology and Pharmacology | 300 | ||
13.1 Introduction | 300 | ||
13.2 Historical Context: Computational Toxicology | 301 | ||
13.2.1 Background | 301 | ||
13.2.2 Programs at University of California Berkeley and University of Michigan | 302 | ||
13.3 Inquiry-Based Science Courses | 303 | ||
13.4 Current Computational Toxicology Courses | 304 | ||
13.4.1 Toxicology Tutorials | 305 | ||
13.4.2 Course Concepts | 305 | ||
13.4.3 Case Studies | 306 | ||
13.4.3.1 Chemical Structural Features Determine Biological Effects | 306 | ||
Case Study Example 1 | 306 | ||
Case Study Example 2.There is wide agreement that chemicals of great concern are those that: persist (P), bioaccumulate (B), and... | 307 | ||
Case Study Example 3 | 307 | ||
Case Study Example 4 | 308 | ||
Typical Report for Case Studies (One Per Team if Applicable) | 308 | ||
13.4.3.2 Herbal Traditional Medicines | 308 | ||
Case Study Example 5 | 308 | ||
Case Study Example 6 | 309 | ||
13.4.3.3 Environmental Chemicals and Health Relationships | 309 | ||
Case Study Example 7.A current topic is selected that recently appeared in the scientific literature or the news media. This top... | 309 | ||
13.5 Course Projects | 310 | ||
13.5.1 Starting Projects | 310 | ||
13.5.2 Typical Project Categories | 310 | ||
13.5.3 Therapeutics vs. Environmental Chemicals | 311 | ||
13.5.4 Challenges in Computational Toxicology | 311 | ||
13.5.4.1 Hazard-Based Information Gathering | 311 | ||
13.6 Sample Project: The Chemical of Concern Question | 312 | ||
13.6.1 Health Effects Inquiry | 313 | ||
13.6.2 Endpoints for Breast Cancer | 313 | ||
13.6.3 Project Question | 314 | ||
13.7 Course Projects Presented and Published | 314 | ||
13.7.1 Projects Presented at National Scientific Meetings | 314 | ||
13.7.1.1 Society of Environmental Toxicology and Chemistry (SETAC) | 314 | ||
13.7.1.2 National Society of Toxicology Through 2015 | 314 | ||
13.7.2 Projects from the Courses Published in Journals | 316 | ||
13.8 Computational Pharmacology as Part of the Principles of Drug Action | 316 | ||
13.9 Conclusion | 318 | ||
References | 319 | ||
Subject Index | 324 |