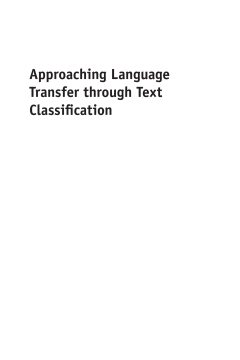
BOOK
Approaching Language Transfer through Text Classification
Dr. Scott Jarvis | Scott A. Crossley
(2012)
Additional Information
Book Details
Abstract
Recent work has pointed to the need for a detection-based approach to transfer capable of discovering elusive crosslinguistic effects through the use of human judges and computer classifiers that can learn to predict learners’ language backgrounds based on their patterns of language use. This book addresses that need. It details the nature of the detection-based approach, discusses how this approach fits into the overall scope of transfer research, and discusses the few previous studies that have laid the groundwork for this approach. The core of the book consists of five empirical studies that use computer classifiers to detect the native-language affiliations of texts written by foreign language learners of English. The results highlight combinations of language features that are the most reliable predictors of learners’ language backgrounds.
Scott Jarvis (Ph.D., Indiana University) holds the title of Professor in the Department of Linguistics at Ohio University, where his main research interests include crosslinguistic influence, cognitive linguistics, and research methods related to the investigation of language proficiency and the measurement of lexical diversity. His work in these areas has appeared in several authored and edited books, numerous book chapters and journal papers in the fields of second language acquisition and multilingualism. Professor Jarvis is also Associate Executive Director for the journal Language Learning.
Scott A. Crossley is an Assistant Professor at Georgia State University. His work involves the application of natural language processing theories and approaches for investigating second language acquisition, text readability, and writing proficiency. His current research interests include lexical proficiency, writing quality, and text coherence and processing.
In this bold and pioneering interdisciplinary study, experts on SLA research, computational analysis and statistics collaborate to try to identify the L1 background of non-native writers. The result is a most impressive work which will take the field of crosslinguistic studies a long way forward. A MUST for all SLA researchers!
Håkan Ringbom, Emeritus Professor, Åbo Akademi University, Finland
This is an articulate, comprehensive, and timely volume on a fascinating yet largely underexplored area. Jarvis and Crossley have produced an impressive collection of research-based evidence on language transfer using a corpus-based approach. The volume is a must-have for students, scholars, and practitioners interested in language transfer, corpus linguistics, forensic linguistics, text classification, second language writing, error analysis, and language assessment.
Pavel Trofimovich, Concordia University, Canada
This book indeed opens a new path in the study of language transfer. The investigations brought together here combine the strengths of earlier work on transfer with those of two other fields, computational linguistics and corpus research, which have much to offer students and researchers interested in second language acquisition and multilingualism. There can be little doubt that the tools used in this seminal work will continue to offer important insights for a long time to come.
Terence Odlin, Ohio State University, USA
Table of Contents
Section Title | Page | Action | Price |
---|---|---|---|
Contents | v | ||
Contributors | vii | ||
1 The Detection-Based Approach: An Overview | 1 | ||
2 Detecting L2 Writers’ L1s on the Basis of Their Lexical Styles | 34 | ||
3 Exploring the Role of n-Grams in L1 Identification | 71 | ||
4 Detecting the First Language of Second Language Writers Using Automated Indices of Cohesion, Lexical Sophistication, Syntactic Complexity and Conceptual Knowledge | 106 | ||
5 Error Patterns and Automatic L1 Identification | 127 | ||
6 The Comparative andCombined Contributions of n-Grams, Coh-Metrix Indices and Error Types in the L1 Classification of Learner Texts | 154 | ||
7 Detection-Based Approaches: Methods, Theories and Applications | 178 |