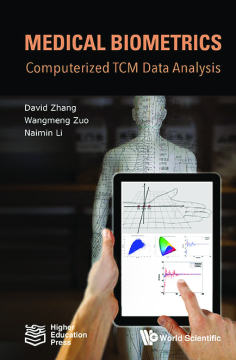
Additional Information
Book Details
Abstract
The introduction of traditional Chinese medicine (TCM) through modern information technology will not only achieve the objective progress of the heritage of thousands of years of TCM, but also deliver novel discoveries for modern medicines.This book is an advanced monograph based on a decade's worth of research work by the authors. After a brief introduction on the four diagnosis approaches in TCM, this book delves into the three main TCM data analysis techniques: computerized tongue, pulse and odor analysis.Both graduate students and researchers in computerized TCM data analysis will benefit from the book as it will provide a comprehensive understanding of the state-of-the-art analysis methods, image / signal acquisition devices, and the related feature extraction and classification methods.
Table of Contents
Section Title | Page | Action | Price |
---|---|---|---|
Contents | ix | ||
Preface | v | ||
PART I: DIAGNOSIS METHODS IN TRADITIONAL CHINESE MEDICINE | 1 | ||
Chapter 1 Introduction | 3 | ||
1.1 Diagnosis Methods in Traditional Chinese Medicine | 3 | ||
1.1.1 Tongue Diagnosis | 3 | ||
1.1.2 Pulse Diagnosis | 5 | ||
1.1.3 Breath Odor Diagnosis | 6 | ||
1.2 Computerized TCM Diagnosis | 7 | ||
1.2.1 Computerized Tongue Diagnosis | 7 | ||
1.2.1.1 Tongue Image Acquisition | 8 | ||
1.2.1.2 Tongue Image Preprocessing | 8 | ||
1.2.1.3 Qualitative Feature Extraction | 10 | ||
1.2.1.4 Diagnostic Classification | 11 | ||
1.2.2 Computerized Pulse Diagnosis | 11 | ||
1.2.2.1 Pulse Signal Acquisition | 12 | ||
1.2.2.2 Baseline Wander Correction | 12 | ||
1.2.2.3 Pulse Feature Extraction | 12 | ||
1.2.2.4 Pulse Diagnosis | 13 | ||
1.2.3 Computerized Breath Odor Diagnosis | 14 | ||
1.2.3.1 Breath Odor Acquisition and Preprocessing | 14 | ||
1.2.3.2 Feature Extraction | 16 | ||
1.2.3.3 Classification and Diagnosis | 16 | ||
1.3 Summary | 17 | ||
References\r | 17 | ||
PART II: COMPUTERIZED TONGUE IMAGE ANALYSIS | 27 | ||
Chapter 2 Tongue Image Acquisition and Preprocessing | 29 | ||
2.1 Tongue Image Acquisition | 29 | ||
2.1.1 Requirement Analysis | 31 | ||
2.1.2 System Design and Implementation | 33 | ||
2.1.2.1 Illuminant | 35 | ||
2.1.2.2 Lighting Condition | 37 | ||
2.1.2.3 Camera | 39 | ||
2.1.2.4 System Implementation | 41 | ||
2.1.3 Performance Analysis | 43 | ||
2.1.3.1 Illumination Uniformity | 43 | ||
2.1.3.2 System Consistency | 44 | ||
2.1.3.3 Accuracy | 48 | ||
2.1.3.4 Typical Tongue Images | 48 | ||
2.2 Color Correction | 49 | ||
2.2.1 Color Correction Algorithms | 51 | ||
2.2.2 Evaluation of Correction Algorithms | 53 | ||
2.2.3 Discussion | 61 | ||
2.2.3.1 Color Correction for Images Acquired by Different Cameras | 62 | ||
2.2.3.2 Color Correction by Different Lighting Conditions | 63 | ||
2.2.3.3 Performance Analysis | 65 | ||
2.2.3.4 Correction on Real Tongue Images | 65 | ||
2.3 Summary | 67 | ||
References\r | 68 | ||
Chapter 3 Automated Tongue Segmentation | 73 | ||
3.1 Bi-Elliptical Deformable Contour | 73 | ||
3.1.1 Bi-Elliptical Deformable Template for the Tongue | 74 | ||
3.1.1.1 Definitions and Notations | 74 | ||
3.1.1.2 The Tongue Template | 75 | ||
3.1.1.3 Energy Function for the Tongue Template | 76 | ||
3.1.2 Combined Model for Tongue Segmentation | 78 | ||
3.1.2.1 Two Kinds of Template Forces | 79 | ||
3.1.2.2 Bi-Elliptical Deformable Contours | 82 | ||
3.1.2.3 Tongue Segmentation Algorithm | 83 | ||
3.1.3 Results and Analysis | 84 | ||
3.2 Snake with Polar Edge Detector | 91 | ||
3.2.1 The Segmentation Algorithm | 91 | ||
3.2.1.1 Polar Edge Detection of Tongue Image | 92 | ||
3.2.1.2 Filtering and Binarization of Edge Image | 94 | ||
3.2.1.3 Initialization and Active Contour Model | 96 | ||
3.2.1.4 Summary of the Automated Tongue Segmentation Method | 97 | ||
3.2.2 Experimental Results | 99 | ||
3.2.2.1 Evaluation on the Edge-Filtering Algorithm | 99 | ||
3.2.2.2 Qualitative Evaluation | 99 | ||
3.2.2.3 Quantitative Evaluation | 99 | ||
3.3 Gabor Magnitude-based Edge Detection and Fast Marching | 104 | ||
3.3.1 2D Gabor Magnitude-based Edge Detection | 105 | ||
3.3.1.1 2D Gabor Magnitude - based Detector | 105 | ||
3.3.1.2 Edge Thresholding | 108 | ||
3.3.2 Contour Detection Using Fast Marching and Active Contour Model | 109 | ||
3.3.2.1 Selection of Stable Segments | 109 | ||
3.3.2.2 Contour Initialization Using Fast Marching | 109 | ||
3.3.2.3 Gradient Vector Flow Snake | 111 | ||
3.3.3 Experimental Results | 111 | ||
3.4 Summary | 114 | ||
References\r | 114 | ||
Chapter 4 Tongue Image Feature Analysis | 117 | ||
4.1 Color Feature Analysis | 117 | ||
4.1.1 Exploratory Tongue Color Analysis | 118 | ||
4.1.1.1 Color Space | 118 | ||
4.1.1.2 Tongue Color Analysis with Locally Linear Embedding | 119 | ||
4.1.1.3 Evaluation Criterion for Parameter Selection | 120 | ||
4.1.1.4 Results and Analysis | 122 | ||
4.1.2 Statistical Analysis of Tongue Color Distribution | 124 | ||
4.1.2.1 Tongue Color Gamut: Generation and Modeling | 125 | ||
4.1.2.2 Tongue Color Centers | 134 | ||
4.1.2.3 Distribution of Typical Image Features | 137 | ||
4.1.2.4 Separation of Tongue Coating and Substance | 141 | ||
4.2 Tongue Texture Analysis | 143 | ||
4.3 Tongue Shape Analysis | 144 | ||
4.3.1 Shape Correction | 144 | ||
4.3.1.1 Automatic Contour Extraction | 145 | ||
4.3.1.2 The Length Criterion | 145 | ||
4.3.1.3 The Area Criterion | 146 | ||
4.3.1.4 The Angle Criterion | 147 | ||
4.3.1.5 Correction by Combination | 148 | ||
4.3.2 Extraction of Shape Features | 149 | ||
4.3.2.1 The Length-based Feature | 149 | ||
4.3.2.2 The Area-based Feature | 151 | ||
4.3.2.3 The Angle-based Feature | 153 | ||
4.3.3 Tongue Shape Classification | 153 | ||
4.3.3.1 Modeling The Classification as A Hierarchy | 155 | ||
4.3.3.2 Calculating Relative Weights | 156 | ||
4.3.3.3 Calculating The Global Weights | 157 | ||
4.3.3.4 Fuzzy Shape Classification | 157 | ||
4.4 Extraction of Other Local Pathological Features | 158 | ||
4.4.1 Petechia | 158 | ||
4.4.2 Tongue Crack | 160 | ||
4.4.3 Tongueprint | 160 | ||
4.4.4 Sublingual Veins | 161 | ||
4.5 Summary | 162 | ||
References\r | 163 | ||
Chapter 5 Computerized Tongue Diagnosis | 167 | ||
5.1 Bayesian Network for Computerized Tongue Diagnosis | 167 | ||
5.1.1 Quantitative Pathological Features Extraction | 167 | ||
5.1.2 Bayesian Networks | 169 | ||
5.1.3 Experimental Results | 171 | ||
5.1.3.1 Bayesian Network Classifier Based on Textural Features | 174 | ||
5.1.3.2 Bayesian Network Classifier Based on Chromatic Features | 174 | ||
5.1.3.3 Bayesian Network Classifier Based on Combined Features | 176 | ||
5.2 Diagnosis Based on Hyperspectral Tongue Images | 178 | ||
5.2.1 Hyperspectral Tongue Images | 179 | ||
5.2.2 The SVM Classifier Applied to Hyperspectral Tongue Images | 180 | ||
5.2.2.1 Linear SVM: Linearly Separable | 180 | ||
5.2.2.2 Linear SVM: Linearly Non-Separable | 181 | ||
5.2.2.3 Non-linear SVM | 182 | ||
5.2.3 Experimental Results | 183 | ||
5.2.3.1 Experiment 1: Comparing Linear and Non-Linear SVM, RBFNN and K-NN Classifiers | 183 | ||
5.2.3.2 Experiment 2: Evaluating the Diagnostic Performance of SVM | 184 | ||
5.3 Summary | 186 | ||
References\r | 187 | ||
PART III: COMPUTERIZED PULSE SIGNAL ANALYSIS | 189 | ||
Chapter 6 Pulse Signal Acquisition and Preprocessing | 191 | ||
6.1 Pressure Pulse Signal Acquisition | 191 | ||
6.1.1 Application Scenario and Requirement Analysis | 192 | ||
6.1.2 System Architecture | 193 | ||
6.1.2.1 Mechanical Structure | 194 | ||
6.1.2.2 Sensor | 196 | ||
6.1.2.3 Circuit | 199 | ||
6.1.2.4 Software Architecture | 201 | ||
6.1.3 Multi-Channel Pulse Signals | 201 | ||
6.2 Baseline Wander Correction of Pulse Signals | 206 | ||
6.2.1 Detecting the Onsets of Pulse Wave | 207 | ||
6.2.2 Wavelet Based Cascaded Adaptive Filter | 209 | ||
6.2.2.1 The Design of CAF Filter | 209 | ||
6.2.2.2 Detection the Level of Baseline Wander Using ER | 212 | ||
6.2.2.3 The Discrete Meyer Wavelet Filter | 216 | ||
6.2.2.4 Cubic Spline Estimation Filter | 219 | ||
6.2.3 Results on Actual Pulse Signals | 221 | ||
6.3 Summary | 223 | ||
References | 224 | ||
Chapter 7 Feature Extraction of Pulse Signals | 227 | ||
7.1 Spatial Feature Extraction | 227 | ||
7.1.1 Fiducial Point-based Methods | 227 | ||
7.1.2 Approximate Entropy | 229 | ||
7.2 Frequency Feature Extraction | 230 | ||
7.2.1 Hilbert-Huang Transform | 230 | ||
7.2.2 Wavelet and Wavelet Packet Transform | 232 | ||
7.2.2.1 Wavelet Transform | 233 | ||
7.2.2.2 Wavelet Packet Transform | 233 | ||
7.3 AR Model | 234 | ||
7.4 Gaussian Mixture Model | 236 | ||
7.4.1 Two-term Gaussian Model | 236 | ||
7.4.2 Feature Selection | 240 | ||
7.4.3 FCM Clustering | 242 | ||
7.5 Summary | 242 | ||
References | 243 | ||
Chapter 8 Classification of Pulse Signals | 245 | ||
8.1 Pulse Waveform Classification | 245 | ||
8.1.1 Modules of Pulse Waveform Classification | 246 | ||
8.1.1.1 Digital Pulse Waveform Acquisition | 246 | ||
8.1.1.2 Pulse Waveform Preprocessing | 248 | ||
8.1.1.3 Pulse Waveform Feature Extraction and Classification | 249 | ||
8.1.2 The EDFC and GEKC Classifiers | 251 | ||
8.1.2.1 ERP, DFWKNN, and KDFWKNN | 251 | ||
8.1.2.2 EDFC and GEKC | 252 | ||
8.1.3 Experimental Results | 255 | ||
8.2 Arrhythmic Pulses Detection | 257 | ||
8.2.1 Clinical Value of Pulse Rhythm Analysis | 257 | ||
8.2.2 Automatic Recognition of Pulse Rhythms | 259 | ||
8.2.2.1 Lempel-Ziv Complexity Analysis | 259 | ||
8.2.2.2 Definitions and Basic Facts | 260 | ||
8.2.2.3 Automatic Recognition of Pulse Patterns Distinctive in Rhythm | 263 | ||
8.2.3 Experimental Results | 272 | ||
8.3 Combination of Heterogeneous Features for Pulse Diagnosis | 274 | ||
8.3.1 Multiple Kernel Learning | 275 | ||
8.3.1.1 Kernel Functions | 276 | ||
8.3.1.2 SimpleMKL | 277 | ||
8.3.2 Experimental Results and Discussion | 279 | ||
8.3.2.1 Classification Experimental of Wrist Blood Flow Signal | 279 | ||
8.3.2.2 Pulse Waveform Classification | 282 | ||
8.4 Summary | 282 | ||
References | 283 | ||
PART IV: COMPUTERIZED ODOR SIGNAL ANALYSIS | 287 | ||
Chapter 9 Breath Analysis System: Design and Optimization | 289 | ||
9.1 Breath Analysis | 289 | ||
9.2 Design of Breath Analysis System | 291 | ||
9.2.1 Description of the System | 291 | ||
9.2.1.1 Breath Gas Collecting | 292 | ||
9.2.1.2 Signal Sampling | 293 | ||
9.2.2 Signal Sampling and Preprocessing | 296 | ||
9.2.2.1 Sampling Procedure | 297 | ||
9.2.2.2 Preprocessing | 298 | ||
9.3 Sensor Selection | 299 | ||
9.3.1 Linear Discriminant Analysis | 299 | ||
9.3.1.1 Data Expression | 300 | ||
9.3.1.2 The Optimum Direction of LDA | 301 | ||
9.3.1.3 Difference Between Two Classes as the Linear Combination of Sensors | 302 | ||
9.3.1.4 Weight of Sensor | 304 | ||
9.3.1.5 Algorithm | 304 | ||
9.3.2 Sensor Selection in Breath Analysis System | 304 | ||
9.3.2.1 Sensor Selection for Disease Diagnosis | 305 | ||
9.3.2.2 Sensor Selection for Evaluating the Medical Treatment | 311 | ||
9.3.3 Comparison Experiment and Performance Analysis | 314 | ||
9.3.3.1 Sensor Selection for Disease Diagnosis | 314 | ||
9.3.3.2 Sensor Selection for Evaluating the Medical Treatment | 316 | ||
9.4 Summary | 317 | ||
References | 317 | ||
Chapter 10 Feature Extraction and Classification of Breath Odor Signals | 321 | ||
10.1 Feature Extraction of Odor Signals | 321 | ||
10.1.1 Geometry Features | 322 | ||
10.1.2 Principal Component Analysis | 324 | ||
10.1.3 Wavelet Packet Decomposition | 324 | ||
10.1.4 Gaussian Function Representation | 325 | ||
10.1.5 Gaussian Basis Representation | 331 | ||
10.1.6 Experimental Results | 334 | ||
10.1.6.1 Experiment I: Decide Which Method is the Best | 334 | ||
10.1.6.2 Experiment II: Test for GBR | 336 | ||
10.2 Common Classifiers for Odor Signal Classification | 336 | ||
10.2.1 K Nearest Neighbor | 337 | ||
10.2.2 Artificial Neural Network | 337 | ||
10.2.3 Support Vector Machine | 337 | ||
10.3 Sparse Representation Classification | 338 | ||
10.3.1 Data Expression | 338 | ||
10.3.2 Test Sample Representation by Training Samples | 339 | ||
10.3.3 Samples Sampling Errors | 340 | ||
10.3.4 Voting Rules | 341 | ||
10.3.4.1 Coefficients Based Voting Rule | 341 | ||
10.3.4.2 Residue Based Voting Rule | 341 | ||
10.3.5 Identification Steps | 342 | ||
10.4 Support Vector Ordinal Regression | 342 | ||
10.4.1 Problem Analysis | 342 | ||
10.4.2 Basic Idea of Support Vector Regression | 343 | ||
10.4.3 Support Vector Ordinal Regression | 344 | ||
10.4.4 The Dual Problem | 345 | ||
10.4.5 Identification Steps | 346 | ||
10.5 Evaluation on Classification methods | 347 | ||
10.5.1 Evaluation on SRC | 347 | ||
10.5.2 Evaluation on SRC | 351 | ||
10.6 Summary | 355 | ||
References | 355 | ||
Index | 359 |