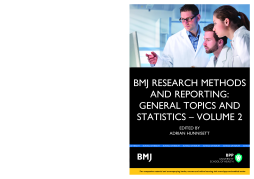
BOOK
BMJ Research Methods and Reporting: General topics & statistics volume 2
(2016)
Additional Information
Book Details
Abstract
The routine use of patient reported outcome measures in healthcare settings
Strengths and weaknesses of hospital standardised mortality ratios
Importance of accurately identifying disease in studies using electronic health records
Verification problems in diagnostic accuracy studies: consequences and solutions
Interpretation of random effects meta-analyses
Differential dropout and bias in randomised controlled trials: when it matters and when it may not
Rethinking pragmatic randomised controlled trials: introducing the "cohort multiple randomised controlled trial" design
Correlation in restricted ranges of data
Is a subgroup effect believable? Updating criteria to evaluate the credibility of subgroup analyses
Target practice: choosing target conditions for test accuracy studies that are relevant to clinical practice
Use of serial qualitative interviews to understand patients' evolving experiences and needs
Use of multiperspective qualitative interviews to understand patients' and carers' beliefs, experiences, and needs
Meta-analysis of individual participant data: rationale, conduct, and reporting
An IV for the RCT: using instrumental variables to adjust for treatment contamination in randomised controlled trials
Random measurement error and regression dilution bias
The double jeopardy of clustered measurement and cluster randomisation
Implementation research: what it is and how to do it
A multicomponent decision tool for prioritising the updating of systematic reviews
The impact of outcome reporting bias in randomised controlled trials on a cohort of systematic reviews
Assessing equity in systematic reviews: realising the recommendations of the Commission on Social Determinants of Health
Prognosis and prognostic research: what, why, and how?
Prognosis and prognostic research: Developing a prognostic model
Prognosis and prognostic research: validating a prognostic model
Prognosis and prognostic research: application and impact of prognostic models in clinical practice
Ten steps towards improving prognosis research
Prognosis research strategy (PROGRESS) 1: A framework for researching clinical outcomes
Prognosis Research Strategy (PROGRESS) 2: Prognostic factor research
Prognosis Research Strategy (PROGRESS) 3: Prognostic model research
Prognosis research strategy (PROGRESS) 4: Stratified medicine research
Table of Contents
Section Title | Page | Action | Price |
---|---|---|---|
Book Cover | C | ||
Title | i | ||
Copyright | ii | ||
About the publisher | iii | ||
About The BMJ | iii | ||
Contents | iv | ||
About the editors | vi | ||
Introduction to Research Methods and Reporting series | vii | ||
The routine use of patient reported outcome measures in healthcare settings | 1 | ||
Using an appropriate validated measure | 1 | ||
Data collection and storage | 2 | ||
Minimising missing and duplicated data | 3 | ||
Thinking about data analysis | 3 | ||
Conclusions | 3 | ||
Strengths and weaknesses of hospital standardised mortality ratios | 5 | ||
What is an HSMR? | 5 | ||
How they are used | 5 | ||
Methodological uncertainties | 5 | ||
Numerator | 5 | ||
Denominator | 5 | ||
Risk modelling | 7 | ||
Interpretation | 7 | ||
Coding | 8 | ||
Importance of accurately identifying disease in studies using electronic health records | 9 | ||
How disease classification errors affect study conclusions | 9 | ||
Estimating bias | 9 | ||
Why does this problem happen? | 11 | ||
Assessment of bias | 11 | ||
Conclusion | 12 | ||
Verification problems in diagnostic accuracy studies: consequences and solutions | 14 | ||
Partial verification | 14 | ||
Clinical examples of partial verification | 14 | ||
Potential for bias | 14 | ||
Corrections for partial verification bias | 15 | ||
Differential verification | 15 | ||
Clinical example | 16 | ||
Further corrections for differential verification bias | 17 | ||
Conclusion | 17 | ||
Interpretation of random effects meta-analyses | 19 | ||
Difference between fixed effect and random effects meta-analyses | 19 | ||
Fixed effect meta-analysis | 19 | ||
Random effects meta-analysis | 19 | ||
Use and interpretation of meta-analysis in practice | 20 | ||
Benefits of using prediction intervals | 20 | ||
Examples | 21 | ||
Antidepressants for reducing pain in fibromyalgia syndrome | 21 | ||
Inpatient rehabilitation in geriatric patients | 21 | ||
Discussion | 21 | ||
Differential dropout and bias in randomised controlled trials: when it matters and when it may not | 23 | ||
Introduction | 23 | ||
Example | 23 | ||
Key concepts | 23 | ||
Types of missing data | 23 | ||
Statistical analysis: what works and what does not work | 24 | ||
Simulation study | 25 | ||
Discussion | 26 | ||
Rethinking pragmatic randomised controlled trials: introducing the “cohort multiple randomised controlled trial” design | 28 | ||
Introduction | 28 | ||
Problems with randomised controlled trials | 28 | ||
Recruitment | 28 | ||
Ethics | 28 | ||
Patient preferences | 28 | ||
Treatment comparisons | 28 | ||
Previous solutions | 29 | ||
The “cohort multiple randomised controlled trial” design | 29 | ||
Randomisation: random selection of some | 29 | ||
Information and consent: “patient centred” | 30 | ||
Benefits of the approach | 30 | ||
Role of the cmRCT design | 30 | ||
Challenges of the design | 30 | ||
Examples of the cmRCT design | 31 | ||
Summary | 31 | ||
Correlation in restricted ranges of data | 33 | ||
Is a subgroup effect believable? Updating criteria to evaluate the credibility of subgroup analyses | 34 | ||
Introduction | 34 | ||
Relative versus absolute effect in subgroup analyses | 34 | ||
The original seven criteria for subgroup analyses | 35 | ||
New criteria to judge the credibility of subgroup effects | 35 | ||
1 Is the subgroup variable a characteristic measured at baseline or after randomisation? | 35 | ||
2 Was the direction of the subgroup effect specified a priori? | 35 | ||
3 Is the significant subgroup effect independent? | 35 | ||
4 Is the interaction consistent across closely related outcomes within the study? | 36 | ||
Discussion | 37 | ||
Target practice: choosing target conditions for test accuracy studies that are relevant to clinical practice | 39 | ||
Defining disease for test accuracy studies | 39 | ||
The target condition | 39 | ||
Why target condition should be clinically defined | 40 | ||
Clinical implications | 41 | ||
Research implications | 41 | ||
Use of serial qualitative interviews to understand patients’ evolving experiences and needs | 43 | ||
When to use serial interviews | 43 | ||
How do you conduct serial interview studies? | 43 | ||
Recruitment | 43 | ||
Data generation | 43 | ||
Analysis | 44 | ||
What type of findings might you expect? | 44 | ||
Issues that change over time | 44 | ||
Rich and contextualised accounts | 45 | ||
Pitfalls and how to avoid them | 45 | ||
Ethical issues | 45 | ||
Attrition | 45 | ||
Data overload | 45 | ||
Conclusions | 45 | ||
Use of multiperspective qualitative interviews to understand patients’ and carers’ beliefs, experiences, and needs | 47 | ||
When are multiperspective interviews appropriate? | 47 | ||
How do you conduct multiperspective interview studies? | 47 | ||
Recruitment | 47 | ||
Data generation | 47 | ||
Analysis | 48 | ||
What type of findings might you expect? | 48 | ||
Understanding of relationships and dynamics | 48 | ||
Comparison of perceptions of patients, their family, and carers | 48 | ||
Understanding of individual needs of participants | 49 | ||
Suggestions for improving services | 49 | ||
Potential pitfalls and how to avoid them | 49 | ||
Recruitment issues | 49 | ||
Patients and carers opting to be interviewed together | 49 | ||
Ethical issues | 49 | ||
Lack of clarity about aims and analytical strategy | 49 | ||
Conclusions | 49 | ||
Meta-analysis of individual participant data: rationale, conduct, and reporting | 51 | ||
What are individual participant data? | 51 | ||
What is an individual participant data meta-analysis? | 51 | ||
Incidence of individual participant data meta-analyses over time | 52 | ||
When do an aggregate data meta-analysis and an individual participant data meta-analysis coincide? | 52 | ||
What are the advantages of a meta-analysis of individual participant data? | 53 | ||
Differences in conclusions with regard to a treatment effect | 53 | ||
Example 1: Effectiveness of laparoscopic repair at reducing persistent pain | 53 | ||
Example 2: Effectiveness of paternal white blood cell immunisation at reducing recurrent miscarriage | 53 | ||
Differences in conclusions with regard to how patient level characteristics modify treatment effect | 53 | ||
Example 1: Effect of elevated panel reactive antibodies on the effectiveness of antilymphocyte antibody induction | 53 | ||
Example 2: Effect of gender on the effectiveness of hypertension treatment | 54 | ||
Beyond the “grand mean” | 54 | ||
What are the disadvantages of a meta-analysis of individual participant data? | 54 | ||
How to obtain individual participant data for a meta-analysis | 55 | ||
Reporting individual participant data meta-analyses | 55 | ||
An applied example of an individual participant data meta-analysis of hypertension trials | 55 | ||
Conclusions | 56 | ||
An IV for the RCT: using instrumental variables to adjust for treatment contamination in randomised controlled trials | 58 | ||
Introduction | 58 | ||
Background: What do we do now, and what’s wrong with it? | 58 | ||
Using instrumental variables to help us understand results of randomised controlled trials | 59 | ||
Real world examples | 60 | ||
What are the benefits and limitations of CA ITT estimates? | 60 | ||
Advanced IV analysis | 61 | ||
Conclusion | 61 | ||
Random measurement error and regression dilution bias | 63 | ||
Introduction | 63 | ||
Example | 63 | ||
Random measurement error in the exposure (X) variable | 63 | ||
Measurement error in the outcome (Y) variable | 64 | ||
Why does the slope not flatten in this situation? | 64 | ||
Recommendations for researchers | 65 | ||
The double jeopardy of clustered measurement and cluster randomisation | 67 | ||
Clustered measurement | 67 | ||
Combined clustering: “double jeopardy” | 67 | ||
Recent example | 68 | ||
What can be done to minimise double clustering? | 69 | ||
Conclusion | 69 | ||
Implementation research: what it is and how to do it | 70 | ||
Defining implementation research | 70 | ||
Principles of implementation research | 70 | ||
Implementation outcome variables | 71 | ||
Implementation strategies | 71 | ||
Implementation influencing variables | 71 | ||
Implementation research questions | 71 | ||
Implementation specific research methods | 72 | ||
Pragmatic trials | 73 | ||
Effectiveness-implementation hybrid trials | 73 | ||
Quality improvement studies | 73 | ||
Participatory action research | 74 | ||
Mixed methods | 74 | ||
Conclusion | 74 | ||
A multicomponent decision tool for prioritising the updating of systematic reviews | 76 | ||
Development and evaluation of the qualitative decision tree | 76 | ||
Development and evaluation of the quantitative tool | 76 | ||
Development and evaluation of the multicomponent updating decision tool | 76 | ||
Overview of the multicomponent updating decision tool | 77 | ||
Documentation and presentation of decisions | 77 | ||
Discussion | 77 | ||
The impact of outcome reporting bias in randomised controlled trials on a cohort of systematic reviews | 81 | ||
Methods | 82 | ||
Assessment of systematic reviews | 82 | ||
Classification of randomised controlled trials in systematic reviews | 82 | ||
Accuracy of classification | 83 | ||
Amount and impact of missing trial data | 83 | ||
Results | 84 | ||
Assessments of systematic reviews | 84 | ||
Full reporting of review primary outcomes in trials | 84 | ||
Classification of trials | 84 | ||
Accuracy of classification | 84 | ||
Amount and impact of missing trial data | 85 | ||
Discussion | 86 | ||
Strengths and limitations of the study | 86 | ||
Comparison with other studies | 87 | ||
Implications for systematic reviews | 87 | ||
Future research | 89 | ||
Assessing equity in systematic reviews: realising the recommendations of the Commission on Social Determinants of Health | 91 | ||
Background to health inequalities and inequities | 91 | ||
The need for adequate reporting of health equity effects | 91 | ||
Process of developing recommendations | 91 | ||
Challenges and recommendations | 92 | ||
1. Developing a logic model | 92 | ||
2. Defining disadvantage and for whom interventions are intended | 92 | ||
3. Deciding on the appropriate study design(s) | 92 | ||
4. Identifying the appropriate outcomes | 92 | ||
5. Process evaluation and understanding context | 93 | ||
6. Data analysis and presentation | 93 | ||
7. Applicability of findings | 94 | ||
Conclusions | 94 | ||
Prognosis and prognostic research: what, why, and how? | 96 | ||
What is prognosis? | 96 | ||
Multivariable research | 96 | ||
Use of prognostic models | 96 | ||
Differences from aetiological research | 97 | ||
How to study prognosis? | 97 | ||
Objective | 97 | ||
Study sample | 97 | ||
Study design | 97 | ||
Predictors | 97 | ||
Outcome | 98 | ||
Required number of patients | 98 | ||
Validation and application of prognostic models | 98 | ||
Prognosis and prognostic research: Developing a prognostic model | 100 | ||
Preliminaries | 100 | ||
Selecting candidate predictors | 100 | ||
Evaluating data quality | 100 | ||
Data handling decisions | 100 | ||
Selecting variables | 101 | ||
Modelling continuous predictors | 101 | ||
Assessing performance | 101 | ||
Example of prognostic model for survival with kidney cancer | 101 | ||
Discussion | 102 | ||
Prognosis and prognostic research: validating a prognostic model | 105 | ||
Why prognostic models may not predict well | 105 | ||
Design of a validation study | 105 | ||
Comparing predictions with observations | 105 | ||
Case studies | 106 | ||
Predicting operative mortality of patients having cardiac surgery | 106 | ||
Predicting postoperative mortality after colorectal surgery | 106 | ||
Predicting failure of non-invasive positive pressure ventilation | 106 | ||
Predicting complications of acute cough in preschool children | 106 | ||
Discussion | 107 | ||
Prognosis and prognostic research: application and impact of prognostic models in clinical practice | 109 | ||
Limitations to application | 109 | ||
Extrapolation versus validation | 109 | ||
Adequate prediction versus application | 109 | ||
Usability | 110 | ||
Changes over time | 110 | ||
Evidence beyond validation studies | 110 | ||
Adjusting and updating prognostic models to improve performance | 110 | ||
Impact of prognostic models | 110 | ||
When to apply a prognostic model? | 111 | ||
Concluding remarks | 111 | ||
Ten steps towards improving prognosis research | 113 | ||
Problems with prognosis research | 113 | ||
Purpose | 113 | ||
Funding | 114 | ||
Protocols | 114 | ||
Predictors | 115 | ||
Outcomes | 115 | ||
Methods | 115 | ||
Publication | 115 | ||
Reporting | 115 | ||
Synthesis | 115 | ||
Impact of research | 116 | ||
Conclusion | 116 | ||
Prognosis research strategy (PROGRESS) 1: A framework for researching clinical outcomes | 118 | ||
What is fundamental prognosis research? | 120 | ||
Importance of fundamental prognosis research in the pathways toward improved health outcomes | 120 | ||
Importance for public health policy | 120 | ||
Importance for comparative effectiveness and health services research | 121 | ||
Importance for health technology assessment of imaging and other tests | 121 | ||
Importance for trials and decision models | 121 | ||
Importance for new approaches, mechanisms, and targets for trials | 121 | ||
Importance for overcoming the limitations of diagnosis | 122 | ||
Importance for discovering new diseases | 122 | ||
Recommendations for improving the quality and impact of prognosis research | 123 | ||
Fuelling changes in medicine and healthcare | 123 | ||
Electronic health records | 123 | ||
Visibility of the field | 124 | ||
Teaching and training | 124 | ||
Patient and public involvement | 124 | ||
Conclusion | 124 | ||
Prognosis research strategy (PROGRESS) 4: Stratified medicine research | 127 | ||
What is stratified medicine? | 127 | ||
Why is prognosis research important for stratified medicine? | 128 | ||
Assessing priorities for stratified medicine | 129 | ||
Evaluation in randomised trials | 129 | ||
Assessment of tests as a health technology | 129 | ||
Cost effectiveness evaluations | 130 | ||
Healthcare policy and delivery | 130 | ||
Recommendations for improving prognosis research for stratified medicine | 130 | ||
False negative findings (type II errors) | 130 | ||
False positive findings (type I errors) | 130 | ||
Analyses restricted to just individuals testing positive for a factor, or just individuals receiving treatment | 131 | ||
Biological reasoning and prioritisation of funding areas | 131 | ||
Conclusions | 132 | ||
More titles in The BMJ Series | 134 | ||
More titles in The BMJ Research Methods and Reporting Series | 135 | ||
More titles in The BMJ Research Methods and Reporting Series | 136 | ||
More titles in The BMJ Easily Missed? Series | 137 | ||
More titles in The BMJ Easily Missed? Series | 138 | ||
More titles in The BMJ Clinical Review Series | 139 | ||
More titles from BPP School of Health | 140 | ||
More titles in The Progressing Your Medical Career Series | 141 | ||
More titles in The Progressing Your Medical Career Series | 142 | ||
More titles in The Progressing Your Medical Career Series | 143 | ||
More titles in The Essential Clinical Handbook Series | 144 | ||
More titles in The Essential Clinical Handbook Series | 145 |