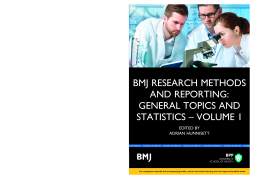
BOOK
BMJ Research Methods and Reporting: General topics & statistics volume 1
(2016)
Additional Information
Book Details
Abstract
Sample size calculations: should the emperor's clothes be off the peg or made to measure?
Implementation research: what it is and how to do it
How to obtain the confidence interval from a P value
The Cochrane Collaboration's tool for assessing risk of bias in randomised trials
Clinical prediction rules
Prognosis research strategy (PROGRESS) 1: A framework for researching clinical outcomes
Out of sight but not out of mind: how to search for unpublished clinical trial evidence
Interpreting diagnostic accuracy studies for patient care
Demystifying trial networks and network meta-analysis
Interpreting and reporting clinical trials with results of borderline significance
Assessing equity in systematic reviews: realising the recommendations of the Commission on Social Determinants of Health
Assessing the value of diagnostic tests: a framework for designing and evaluating trials
Three techniques for integrating data in mixed methods studies
Dangers of non-specific composite outcome measures in clinical trials
Cascade diagrams for depicting complex interventions in randomised trials
Recommendations for examining and interpreting funnel plot asymmetry in meta-analyses of randomised controlled trials
Brackets (parentheses) in formulas
Comparisons within randomised groups can be very misleading
Uncertainties in baseline risk estimates and confidence in treatment effects
How to obtain the P value from a confidence interval
Diagnostic accuracy studies: how to report and analyse inconclusive test results
Making inferences on treatment effects from real world data: propensity scores, confounding by indication, and other perils for the unwary in observational research
The impact of outcome reporting bias in randomised controlled trials on a cohort of systematic reviews
Prognosis research strategy (PROGRESS) 4: Stratified medicine research
Value of composite reference standards in diagnostic research
Statistics Notes: Missing outcomes in randomised trials
Multiple imputation for missing data in epidemiological and clinical research: potential and pitfalls
Table of Contents
Section Title | Page | Action | Price |
---|---|---|---|
Book Cover | C | ||
Title | i | ||
Copyright | ii | ||
About the publisher | iii | ||
About The BMJ | iii | ||
Contents | iv | ||
About the editors | vii | ||
Introduction to Research Methods and Reporting series | viii | ||
Chapter 1 Sample size calculations: should the emperor’s clothes be off the peg or made to measure? | 1 | ||
An example | 1 | ||
What is the distribution of blood pressure in the population you intend to study? | 1 | ||
How much do you think your treatment will affect systolic blood pressure? | 1 | ||
What α and β levels do you want? | 1 | ||
Other approaches to sample size | 2 | ||
Sample size norms for different designs | 2 | ||
Differences between groups | 2 | ||
Measured outcome variable | 2 | ||
Binary outcome variable— proportions | 2 | ||
Relations between continuous variables | 3 | ||
Conclusions | 3 | ||
Chapter 2 The tyranny of power: is there a better way to calculate sample size? | 4 | ||
Power calculations | 4 | ||
Confidence intervals | 5 | ||
Base sample sizes on estimation | 6 | ||
Chapter 3 How to obtain the confidence interval from a P value | 7 | ||
(a) Calculating the confidence interval for a difference | 7 | ||
(b) Calculating the confidence interval for a ratio (log transformation needed) | 7 | ||
Limitations of the method | 7 | ||
P values presented as inequalities | 7 | ||
Chapter 4 How to obtain the P value from a confidence interval | 8 | ||
(a) P from CI for a difference (no transformation needed) | 8 | ||
(b) CI for a ratio (log transformation needed) | 8 | ||
Limitations of the method | 8 | ||
Chapter 5 Clinicians are right not to like Cohen’s κ | 9 | ||
Introduction | 9 | ||
Calculation of Cohen’s κ | 9 | ||
Absolute and relative measures to quantify observer variation | 10 | ||
Cohen’s κ is a reliability measure | 10 | ||
Do clinical questions concern reliability or agreement? | 10 | ||
Proportion of specific agreement as preferred measure of agreement | 11 | ||
Conclusion | 11 | ||
Chapter 6 The Cochrane Collaboration’s tool for assessing risk of bias in randomised trials | 13 | ||
Development of risk assessment tool | 13 | ||
Evaluation phase | 13 | ||
The risk of bias tool | 14 | ||
Evaluation of initial implementation | 15 | ||
Presentation of assessments | 15 | ||
Summary assessment of risk of bias | 16 | ||
Assessments of risk of bias and synthesis of results | 16 | ||
Discussion | 16 | ||
Chapter 7 Taking healthcare interventions from trial to practice | 18 | ||
Study protocol | 18 | ||
Study fidelity: planned versus actual treatment | 18 | ||
Publication of single studies | 19 | ||
Synthesis of evidence and systematic reviews | 20 | ||
Mapping the components of an intervention | 20 | ||
Taxonomies | 20 | ||
Using the study | 20 | ||
Chapter 8 Clinical prediction rules | 22 | ||
Establishing a clinical prediction rule | 22 | ||
Advantages and disadvantages of prediction rules | 23 | ||
Types of prediction model | 23 | ||
Scoring systems derived from univariate analysis | 23 | ||
Prediction models based on multivariate analysis | 23 | ||
Nomograms | 23 | ||
Prediction using artificial neural networks | 24 | ||
Decision trees (CART analysis) | 24 | ||
Conclusion | 24 | ||
Chapter 9 When is a further clinical trial justified? | 26 | ||
Two problems, and solutions | 26 | ||
The smallest worthwhile effect of exercise for chronic back pain | 27 | ||
Existing evidence of the effect of exercise on chronic back pain | 27 | ||
Does exercise produce worthwhile reductions in chronic back pain? | 27 | ||
What influence would the findings of a new trial have? | 27 | ||
Conclusions | 28 | ||
Chapter 10 Out of sight but not out of mind: how to search for unpublished clinical trial evidence | 30 | ||
Trial registries and results databases | 30 | ||
Regulatory agencies | 31 | ||
Contacting trialists and sponsors | 32 | ||
Other sources of information | 32 | ||
Conclusions | 32 | ||
Chapter 11 Interpreting diagnostic accuracy studies for patient care | 34 | ||
Reporting test accuracy at different thresholds | 34 | ||
Presenting results at a single threshold | 34 | ||
Presenting results at multiple thresholds | 34 | ||
Presenting a performance measure combined across thresholds | 35 | ||
Are false positive and false negative diagnoses equally important? | 35 | ||
Presenting diagnostic accuracy for patients | 35 | ||
Comparing the performance of two diagnostic tests | 36 | ||
Paired measures at specific thresholds | 36 | ||
Summary measure at specific thresholds: net benefit methods | 36 | ||
Single measure averaged across multiple thresholds | 36 | ||
Problems with ROC AUC for diagnostic performance | 36 | ||
AUC or partial AUC? | 36 | ||
Extrapolation beyond available data | 36 | ||
Incorporating relative misclassification costs | 37 | ||
Incorporating disease prevalence | 38 | ||
Summary | 38 | ||
Chapter 12 Demystifying trial networks and network meta-analysis | 40 | ||
Introduction | 40 | ||
Part 1: network geometry | 40 | ||
Part 2: heterogeneity and incoherence | 41 | ||
Part 3: data synthesis | 42 | ||
Summary | 43 | ||
Chapter 13 Interpreting and reporting clinical trials with results of borderline significance | 44 | ||
What is the problem? | 44 | ||
Using confidence intervals | 44 | ||
Inconsistency in language in clinical trial reports | 45 | ||
Possible solutions | 45 | ||
Recommendations | 47 | ||
Chapter 14 Statistics Notes: Missing outcomes in randomised trials | 48 | ||
Chapter 15 Strategy for intention to treat analysis in randomised trials with missing outcome data | 50 | ||
Attempt to follow up all randomised participants | 50 | ||
Perform a plausible main analysis | 50 | ||
Perform sensitivity analyses | 50 | ||
Account for all randomised participants in the sensitivity analyses | 50 | ||
Example of strategy in action | 51 | ||
Discussion | 51 | ||
Chapter 16 Multiple imputation for missing data in epidemiological and clinical research: potential and pitfalls | 53 | ||
Consequences of missing data | 53 | ||
Statistical methods to handle missing data | 53 | ||
What is multiple imputation? | 54 | ||
Pitfalls in multiple imputation analyses | 54 | ||
Omitting the outcome variable from the imputation procedure | 54 | ||
Dealing with non-normally distributed variables | 54 | ||
Plausibility of missing at random assumption | 55 | ||
Data that are missing not at random | 55 | ||
Computational problems | 55 | ||
Practical implications | 55 | ||
Reporting in recent literature | 55 | ||
Suggested reporting guidelines | 56 | ||
Summary | 56 | ||
Chapter 17 Assessing the value of diagnostic tests: a framework for designing and evaluating trials | 58 | ||
Effect of tests on patient health | 58 | ||
Direct test effects | 58 | ||
Test procedure | 58 | ||
Altering clinical decisions and actions | 58 | ||
Feasibility and interpretability | 58 | ||
Test accuracy, diagnostic yield, therapeutic yield, and treatment efficacy | 59 | ||
Diagnostic and therapeutic confidence | 59 | ||
Changing timeframes of decisions and actions | 59 | ||
Influencing patient and clinician perceptions | 60 | ||
Patients | 60 | ||
Adherence to treatment | 60 | ||
Doctors | 60 | ||
Systemic approach to evaluating tests | 60 | ||
Conclusion | 62 | ||
Chapter 18 Three techniques for integrating data in mixed methods studies | 64 | ||
Triangulation protocol | 64 | ||
Following a thread | 65 | ||
Mixed methods matrix | 65 | ||
Conclusion | 66 | ||
Chapter 19 Dangers of non-specific composite outcome measures in clinical trials | 68 | ||
Motivating example: planning a clinical trial | 68 | ||
Explaining treatment effects with a causal mechanism model | 68 | ||
Composite outcomes | 69 | ||
Misclassification of outcome | 69 | ||
Examples from real trials | 70 | ||
Discussion | 70 | ||
Chapter 20 Cascade diagrams for depicting complex interventions in randomised trials | 72 | ||
Hierarchical interventions | 72 | ||
Trials | 72 | ||
More complex interventions | 73 | ||
Discussion | 73 | ||
Chapter 21 Recommendations for examining and interpreting funnel plot asymmetry in meta-analyses of randomised controlled trials | 76 | ||
What is a funnel plot? | 76 | ||
Implications of heterogeneity, reporting bias, and chance | 76 | ||
Heterogeneity | 76 | ||
Reporting bias | 77 | ||
Chance | 78 | ||
Interpreting funnel plot asymmetry | 78 | ||
Statistical tests for funnel plot asymmetry | 78 | ||
Funnel plots and meta-analysis models | 79 | ||
Fixed and random effects models | 79 | ||
Extrapolation of a funnel plot regression line | 79 | ||
Discussion | 80 | ||
Chapter 22 Brackets (parentheses) in formulas | 82 | ||
Chapter 23 Comparisons within randomised groups can be very misleading | 83 | ||
Chapter 24 Uncertainties in baseline risk estimates and confidence in treatment effects | 85 | ||
Risk of bias | 85 | ||
Publication bias | 86 | ||
Imprecision | 86 | ||
Inconsistency | 86 | ||
Indirectness | 86 | ||
Discussion | 87 | ||
Chapter 25 Value of composite reference standards in diagnostic research | 89 | ||
What is a composite reference standard? | 89 | ||
Developing a composite reference standard | 90 | ||
Defining rules to combine component tests | 90 | ||
Selection of component tests | 90 | ||
Extensions to the basic composite reference standard | 91 | ||
Missing values on component tests | 91 | ||
Reporting guidelines | 91 | ||
Conclusions and recommendations | 91 | ||
Chapter 26 Diagnostic accuracy studies: how to report and analyse inconclusive test results | 93 | ||
Inconsistent reporting of inconclusive test results in research on diagnostic accuracy | 93 | ||
Defining inconclusive results | 94 | ||
Types of inconclusive test results | 94 | ||
Invalid inconclusive results | 94 | ||
Uninterpretable and missing index test results | 94 | ||
Valid inconclusive results | 94 | ||
Continuous inconclusive index test results | 94 | ||
How to report inconclusive results | 95 | ||
Valid inconclusive results | 95 | ||
Continuous inconclusive test results | 95 | ||
Categorical/ordinal inconclusive test results | 95 | ||
Invalid inconclusive results | 95 | ||
Reporting uninterpretable and missing inconclusive test results | 95 | ||
How to analyse inconclusive test results | 96 | ||
Scenario 1: Exclude valid inconclusive results completely | 96 | ||
Scenario 2: Exclude valid inconclusive results from binary statistics but report an additional summary statistic that accounts for them | 96 | ||
Scenario 3: Group valid inconclusive results with positive or negative results | 96 | ||
Analysis of continuous inconclusive test results | 97 | ||
Conclusion | 97 | ||
Making inferences on treatment effects from real world data: propensity scores, confounding by indication, and other perils for the unwary in observational research | 99 | ||
Propensity score analyses | 99 | ||
When things go awry | 100 | ||
Unknown bias | 100 | ||
Confounding by indication | 101 | ||
When is it helpful to use a propensity score analysis? | 101 | ||
More titles in The BMJ Series | 103 | ||
More titles in The BMJ Research Methods and Reporting Series | 104 | ||
More titles in The BMJ Research Methods and Reporting Series | 105 | ||
More titles in The BMJ Easily Missed? Series | 106 | ||
More titles in The BMJ Easily Missed? Series | 107 | ||
More titles in The BMJ Easily Missed? Series | 108 | ||
More titles in The BMJ Clinical Review Series | 109 | ||
More titles from BPP School of Health | 110 | ||
More titles in The Progressing your Medical Career Series | 111 | ||
More titles in The Progressing your Medical Career Series | 112 | ||
More Titles in The Progressing Your Medical Career Series | 113 | ||
More titles in The Essential Clinical Handbook Series | 114 | ||
More titles in The Essential Clinical Handbook Series | 115 |