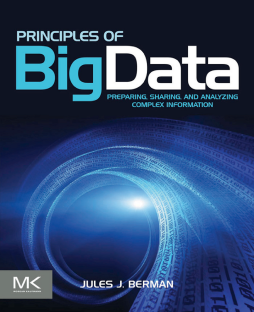
Additional Information
Book Details
Abstract
Principles of Big Data helps readers avoid the common mistakes that endanger all Big Data projects. By stressing simple, fundamental concepts, this book teaches readers how to organize large volumes of complex data, and how to achieve data permanence when the content of the data is constantly changing. General methods for data verification and validation, as specifically applied to Big Data resources, are stressed throughout the book. The book demonstrates how adept analysts can find relationships among data objects held in disparate Big Data resources, when the data objects are endowed with semantic support (i.e., organized in classes of uniquely identified data objects). Readers will learn how their data can be integrated with data from other resources, and how the data extracted from Big Data resources can be used for purposes beyond those imagined by the data creators.
- Learn general methods for specifying Big Data in a way that is understandable to humans and to computers
- Avoid the pitfalls in Big Data design and analysis
- Understand how to create and use Big Data safely and responsibly with a set of laws, regulations and ethical standards that apply to the acquisition, distribution and integration of Big Data resources
"By stressing simple, fundamental concepts, this book teaches readers how to organize large volumes of complex data, and how to achieve data permanence when the content of the data is constantly changing. General methods for data verification and validation, as specifically applied to Big Data resources, are stressed throughout the book." --ODBMS.org, March 2014
"The book is written in a colloquial style and is full of anecdotes, quotations from famous people, and personal opinions." --ComputingReviews.com, February 2014
"The author has produced a sober, serious treatment of this emerging phenomenon, avoiding hype and gee-whiz cases in favor of concepts and mature advice. For example, the author offers ten distinctions between big data and small data, including such factors as goals, location, data structure, preparation, and longevity. This characterization provides much greater insight into the phenomenon than the standard 3V treatment (volume, velocity, and variety)." --ComputingReviews.com, October 2013